The 2nd Wave of Generative AI with Sailesh Ramakrishnan & Madhu Iyer, Managing Partners at Rocketship.vc
Madhu Shalini Iyer is a Managing Partner at Rocketship.vc, a Silicon Valley based fund investing globally. She was the Chief Data Officer of Gojek and helped grow the business into a $10 billion unicorn. In addition to being a board member, she started the Singapore office and played an active role in the strategy, new business development, and ‘data as a competitive advantage’. Prior to Gojek, Madhu was part of the founding team of Intuit’s Quickbooks Lending Platform. As the data science leader at Intuit, Madhu helped grow the platform to $300 million and holds 2 patents in the areas of user data augmented algorithms for financial inclusion. Madhu was also the Chief Data Officer for Ethoslending. There she built the underwriting platform and was responsible for all b2c revenue, resulting in $65 million gross market value per month. Madhu was further responsible for building and running the marketing team. Prior, Madhu was a partner at a $150m private equity fund, Stem Financial, in Hong Kong. She started her career as a senior data scientist with a leading think tank in Menlo Park, CA.
Sailesh Ramakrishnan is also a Managing Partner at Rocketship.vc. Prior to Rocketship.vc, Sailesh was CTO and co-founder of LocBox (acquired by Square), a startup focussed on marketing for local businesses. Sailesh worked with Anand and Venky at their previous startup Kosmix, and continued on to Walmart as a Director of Engineering at @WalmartLabs. Before jumping into the startup world, Sailesh worked as a Computer Scientist at NASA Ames Research Center. Sailesh earned his Bachelors degree in Civil Engineering from IIT Madras, his Masters degree in Construction Management from Virginia Tech and another Master degree in Intelligent Systems from University of Pittsburgh. He was a Ph.D. candidate in Artificial Intelligence at the University of Michigan.
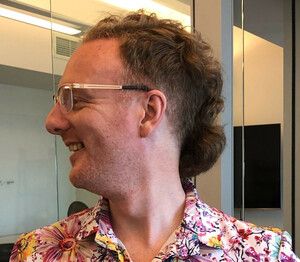
Richie helps individuals and organizations get better at using data and AI. He's been a data scientist since before it was called data science, and has written two books and created many DataCamp courses on the subject. He is a host of the DataFramed podcast, and runs DataCamp's webinar program.
Key Quotes
If you think of the startup as a brightly burning candle, what we are doing with our data is looking at all the reflections surrounding it. Because the reflections are externally visible, they are not on their own. Or any one reflection on its own is not enough to decide great things are happening within the company. But if there is bright light being reflected all around by the startup, then usually it means there's something really fantastic happening.
The one thing that we have learned in the last 10 years of investing with a data-driven approach is that if the data tells you something and it's counterintuitive, don't try to squash that down because you look like the fool in the end, not the data. And so we are, and the counterintuitive stuff, for example, we found the 15 minute delivery space before anybody else had. And we went in and we invested in Europe. And us becoming global investors also as a second example is another sort of our moment. We are a Silicon Valley fund and we wanted to only invest in the Valley when we started. I mean, we didn't know any better, but the data kind of told us better that there was going to be an ecosystem all over the world for startup.
Key Takeaways
Trust data even when it presents counterintuitive insights, as this can lead to pioneering decisions and investments, particularly in emerging markets and new sectors. This approach has historically validated the potential in unexpected areas.
Global insights derived from data can guide expansion beyond traditional markets. Embracing a global perspective can uncover opportunities in regions previously considered non-viable, as seen with the rise of startups in diverse geographical areas.
Maintain an ethical stance while being open to experimentation in AI. This involves setting boundaries for decency and privacy, but also pushing innovation within those limits to explore new possibilities responsibly.
Transcript
Richie: Welcome to DataFramed. This is Richie. The previous episode of DataFramed sought the venture capital perspective on trends in the data industry. And today I have two more venture capitalists to quiz about trends in AI. Sailesh Ramakrishnan and Madhu Shalini Iyer are the managing partners at Rocketship.vc.
They use an algorithmic approach to investing in early stage startups, and as a fan of data driven decision making, I'm keen to hear more about their approach. Unlike many Silicon Valley VC firms, which are very US centric in their investing, Rocketship is deliberately global, so I'm hoping to hear about AI trends from around the world.
The two founders have some strong data and investment credentials. Silas was a co founder and the CTO at Loqbox. and Director of Engineering at Walmart Labs. Meanwhile, Madhu is the Chief Data Officer at Gojek and Ethos Lending, and a partner at Stem Capital Partners. Let's hear what they have to say.
Hi there. Welcome Silas and welcome Madhu to the show. Great to have you both here.
Madhu Iyer: Thank you for having us. You
Richie: Excellent. So we're heading two years into the generative AI revolution and there are now thousands of startups. So do you have a mental model for how you go about categorizing all these new generative AI companies?
Madhu, do you want to go first?
Madhu Iyer: know, we come from data science backgrounds and, and we run a fund that's a very data science heavy fund. And so, you ... See more
It's a data driven fund. What we mean by that is that we are investing in companies. Just like, you know, it's a typical venture capital fund with one difference. Our deed flow comes from our algorithms. We have the largest startup database in the world. It's terabytes of data. And we have a ton of models that run to find out the companies that we want to invest in.
And so that's how we find and invest in companies. because we are so data driven we also use Gen AI in our own models now. we were excited to see a brand of companies emerging, although for us, AI is it's just table stakes in everything that, one does historically we've looked at a lot of companies and has been done for a very long time.
But now with, the spate of companies that have just come up in the last 2 to 4 years we've been looking at it in house to see what is going to be the companies that we get most excited by. So there's foundational models, there's the picks and shovels, you know. companies that do foundational models, companies that do picks and shovels, and then there is the final frontier of application companies and we've looked at all of them and I let Sailesh expound on you each of these three buckets we've been looking at it.
Sailesh Ramakrishnan: Absolutely. As mother mentioned, the fact that we are quant investors or algorithmic investors gives us a very unique perspective on the full spectrum of companies that algorithms see. And if you take the first category that Madhu mentioned, which is foundational models, initially you would have thought of that as just models that were created by large companies, Anthropics, the Coheres and of course the OpenAI's of the world.
And both Facebook and, Google have their large foundation models. and the initial thinking there was, these are very, very large models. They will become sort of a key ingredient across many different applications. But then as space developed, our algorithms started identifying that there's the original class of foundational models, but then there's new classes of foundational models that are arriving as well, where you're either limiting the vocabulary on which the model is built, or actually you're changing the language on where the model is built.
And when I say changing the language, I actually mean not just language from a natural language perspective, but applying this to genomic data, where the language is genetic expression. Applying this to images, where the language is, pixels. Applying this to video. Applying this to, to data coming from climate change, where the language is perhaps the natural processes.
So new classes of foundational models are being created to really bring this large language revolution from traditional language to anything that becomes true as language. In fact, one of the most innovative uses of this we saw was considering motion as language. think of motion very much in the physical world, but motion can be decomposed into confidence as language as well.
So that's the foundational model. The second class are the picks and shovels, as in the companies that are enabling this transition to happen, but they're not necessarily the ones building the core foundation models. They're not necessarily building applications either. And so examples here are vector database companies, companies trying to create ecosystems where these models can be computed, they can be refined, fine tuned.
as well as eventually tested in real world situations. And without these picks and shovels, companies common users not able to take advantage of these models. Only large companies with large development teams can. so these are an integral enabler class of companies. And we are seeing several exciting examples there as well.
But the last category, and this is really where meat of the Gen AI revolution is, is in core application development, where Gen AI is an integral part, but not the only thing in the solution. So you're trying to solve an existing, really hard problem, and Gen AI was the missing piece that was needed in order to make that solution much more workable today than it was in the last, 15, 20 years.
And that's also something that Madhu was mentioning. We've been watching this transition for the last 15, 20 years, but this material change that large models brought about was that missing piece where, you know, you can have a reasonably high quality customer support conversation, but then actually also expect the system to understand enough.
To actually execute a solution and really solve the person's problem rather than just wasting time collecting information and eventually handing off to a human customer support person. I think that translation from a surface level model to deeply solving the problem is what we are seeing in the third class of applications.
And we are seeing this in pretty much. Every space that is out there. There's almost a not a single area whether it be accounting or genomics or healthcare or gaming anything and everything that today we can think of from an innovation perspective has and is being impacted by the Gen AI way.
Richie: I feel like that classification of those
three things, you've got the foundation models, you've got the supporting infrastructure, you've got your databases and your compute platforms of things, and then applications on top of that. That's a very helpful way of thinking about it. it. seems like you're most excited about the application layer of things. So can you talk me through since you're doing algorithmic investing, what's the algorithm showing? which are the most exciting companies in the space?
Sailesh Ramakrishnan: We'd rather not name these companies because these are either inactive conversations in, or we are considering investments in, and there's just a lot of activity going on here. But I will give you examples of the kinds of companies that our models are identifying that are doing something very, very innovative.
Thank you. Let's start with a very dry use case very similar to something that we are ourselves needing to do. There's a lot of information available for large financial companies when they're trying to negotiate a deal or a contract. and this is we're talking millions and millions of pages of documents for corporate for the ecosystem and so on.
And all of this is. In somewhat sort of disorganized fashion available for people to somehow Crystallize in order to make the best decision on contracts with Jenny. I today you can collect all of this information and start asking pointed questions in them saying, Hey, was this company profitable or this company's margins dipped in in three years ago?
Was there a reason? What was the reason that they use and so on? we're able to find some really fantastic examples where the large class off fintech companies, large financial institutions are gaining the benefit off this approach. The second and again, a significantly interesting use case, but much more non traditional happens to be in manufacturing manufacturing.
We see a lot of information about. Machines about places where you have to do repairs, think of it as, you know, air conditioning repair all the way out to preparing jet engines. There's a lot of information and there are only very few experts. And so it takes years of training to become a very qualified person in order to fix, change, modify and improve these devices.
But once again, with the advent of larger language models, you have a way of efficiently consuming all of this information and then using that information from a technical perspective to make these improvements happen. So in both of these examples, trend that we are seeing of the use case, Is one off a copilot where a problem is being solved, but a problem is being solved in conjunction with a human in the loop.
We're also seeing companies that are building solutions where humans are not needed necessarily. examples there include coding solutions where you're looking for, Hey, I want a piece of code to make this translation happen from this system to that system. And the large language models are able to take both the inputs and outputs, figure out what needs to happen and provide you with almost a 95 percent ready solution.
And so that's another example of a company we're seeing. And this is in the sort of the mundane B2B, B2C space. But we are seeing this now the next level of excitement, which is in the B2C space in terms of art, creativity, music. But also things like helping people understand languages better, auto translation with nuance and context.
These are also companies we're seeing. there's so much in this space that I can keep going on for the whole other podcast, so mad chance as well.
Madhu Iyer: Yeah, I think you have covered lot of what we're seeing. I think the one thing that I will just sort of fill in a gap is that health care is a huge segment, you know, you have. You have health tech you know, you have variables and you have new ways of sort of, measuring things from your body that was not possible before.
Just in the last 10, 15 years, we are now being able to use what's called sounds and breathing. and then there's a lot of data. I mean, and it's also very nuanced data. And then there is, there are things like genomics and which, you know, Silas had touched upon in this previous response.
You are now able to sequence genes in a much more elaborate fashion, which was never thought of before. there is, so from the variables to sort of, clinical data to the, the gene side of things to pharma. There's a lot of things you know, that are possible with Gen AI.
And even just mundane things in health tech like chatbot experiences and chat, enhanced chat experiences and enhanced experiences, you know, which are possible because of a feedback loop system. you know, there's a huge amount of companies that we're seeing in that realm that are doing sort of groundbreaking work.
You know, we actually invest in not just B to B and B to C, but we also invest in deep tech and believe it or not, even space technology is enhanced because of Gen AI. And so the applications of it are really wide. And varied from digitizing chemistry to space all the way in deep tech Gen AI is the fundamental layer that's powering and super powering, I would say, and supercharging a lot of these applications,
Richie: I love that between you, you've listed almost every industry there is, and then this is just taking over absolutely everything. But it's amazing how there's that wide range from, you've got some very technical things like FinTech and software development and space research down to more traditional manufacturing and then simple things like customer service chatbots.
so since you're very algorithmically driven in your investments, can you tell me a bit more about what that involves? So you said there's a lot of data in there. What sort of data are you looking at? What sort of techniques are you using?
Madhu Iyer: broadly, I think the one thing that I will just kind of dial a little bit out of the data part of it is to say that, you know, what really is a problem, right? The problem here is that we are venture capital investors. When you make venture capital investments, you have to speak to thousands of companies to pick a few to make your bets on and so the odds are better when you speak to Even your denominator.
The base is large, but you're speaking to a lot of companies. We knew this going in. We're all data practitioners. We have built companies. We have scaled companies and we're interested in companies. what we knew was that A, to become better investors and to pick the best companies, you just have to see a lot of deal flow.
And B, we've done this traditionally in the past as a data science practitioner of trying to find a Y variable with, you know, with millions of Xs. So this is a classical problem. So we wanted to combine those two things. And so as a result of trying to find the best companies, that's our Y, you know, what is a best company?
And you can define that, in hundreds of different ways. And that's why we have hundreds of different models running in our fund. But, what are the Xs now? What are the factors? You know, there's plenty of things. there's a lot of things that are established about a startup the minute it gets founded.
A, there is a record created when, you know, you found a startup and you incorporate a startup. B, you know, it's the founders. C, it's the sector you're in. And that's actually a very nuanced data problem. Like, the classification of a company. Because, you know, if you want to really compare across the world, what are the different kinds of companies, and that forms a trend.
So we actually do bottom up sort of, Investing which is you know, our data kind of goes bottom up. It's not top down We are not trying to juxtapose Anything for example, you started with genai our data told us that genai was a trend a long time ago But we didn't try to sort of say hey find us genai companies.
I'll go, you know We're not trying to juxtapose our own human thoughts so sector is really important and the classification of sectors is really important. Of course, you know folks get funded You Sometimes later, later in the game. And so we also have information about people that have funded it and, you know, and other angels and, and so on and so forth who are funded the company.
And so these are all sort of like, there are some signals that are we have like observational models, which are how fast is the company going and, and what's going on around the world, and then you have predictive models, which are more traditional. It's, you know, you have, you're trying to predict a Y.
With, a certain given amount of excess you can ask that question about what makes a great company. And why do you want to invest in a company that why can be defined in many ways on DSO. There's a lot of models running here in our fund. And there's a lot of different signals that we have found to be very useful.
And I just named a few but you know, you can never tell. I think the ideal answer is a perfect storm of a lot of things, and there are a lot of aha moments, but the one thing that I'll just say, and I'll, I'll, you know, I'll let Sailesh also finish the, finish with his thoughts, but but I will just say that the one thing that we have learned in the last 10 years of investing with a data driven approach, It's that if the data tells you something and it's counterintuitive, don't try to squash that down because you look like the fool in the end, not the data.
And so we are and the counterintuitive stuff. For example, we found the 15 minute delivery space before anybody else had. And we went in and we invested in Europe. and us becoming global investors also as a second example is another sort of aha moment. We are a Silicon Valley fund and we wanted to only invest in the Valley when we started.
I mean, we didn't know any better. But the data kind of told us better that, there was going to be an ecosystem all over the world for startups. And so we became global investors. So those are two examples of sort of the data nuances that we completely trusted we went in with our eyes open with data as parking and it's been a great journey.
Richie: Just to try and summarize what you said. So, when you're looking at the quality of the company, you're sort of looking at the sort of financial metrics, and they're also looking at properties of the founders and management, and they're also looking at the wider macroeconomic environment. so a lot of bits of data there, but then I feel like there's a deep philosophical point that there are lots of ways of measuring what a good company is.
So, Sailesh, do you want to give your take on what does a company being good mean to you?
Sailesh Ramakrishnan: that's actually a very perceptive comment. Richie, this is exactly the challenge that we had to first and foremost consider when we even started the. inspiration for the fund one of the inspirations for the fund were the quant investors on Wall Street because they were the ones who were doing the sort of quantitative statistical rapidly computational training.
And you saw a whole spectrum of people from people who are trying to take arbitrage on millisecond price differences all the way to people who were constructing, holding and building positions. Over days or weeks or months. So when you get inspired by those folks, one of the things you realize is what's in common and what's different, what's in common is the fact that they had data and they were building algorithms.
But the biggest two differences were they were operating in a very liquid market. And so the biggest value they had was immediate feedback. you build up, use all this data, you build a hypothesis, you test the hypothesis and the market tells you whether you were right or wrong within seconds, days, weeks, whatever, very quickly.
Venture investing is a decades long business, most common average time for a startup to become a unicorn is about nine years, There are certain sectors, of course, where companies become unicorns in four years and others, take 12, 13. So the biggest challenge we faced was that we were trying to predict something, the potential for something that was 5, 7, 10 years out in the future.
Right. And so that success the second aspect and then now this was one, the second aspect that the folks on the quant side in public markets had was there were many examples. You can learn from a lot of success examples, right? You predict a stock and it you know, it does well in the next millisecond.
That's a positive example. You predicted the stock going down and it does not, that's a negative example. And so, you know, positive and negative examples were reasonably well distributed in the quant world. Again, for us, as everybody knows, venture business is a very high risk, highly challenging business.
The default for most startups is unfortunately debt. And so the number of potentially successful companies is a very small set. So we are learning a model from a small set of examples. So with that as background, I've already stated how challenging this is. Now, let me tell you what success looks like. So therefore, any measure of success in this environment cannot be something that will take seven, eight years.
You also realize very quickly that startups don't become overnight successes, as in they were completely unknown, you know, eight years, and then suddenly, ninth year, they explode on the horizon. If you look at enough about a company, you'd realize there are many intermediate measures of success. What is their rate of growth?
How well do they employ? What are the quality of the people they employ? How well do they present themselves in the external world? How well do they do in marketing? even trivial things like are they engendering growth in on their social media efforts are all indications of proxies for success on their own.
They're very tiny in terms of the information they provide. But in taken in conjunction with a lot of signals, they're able to give you a better view of what is happening within the company. And I'll use just one quick analogy to illustrate this. To think of the startup as a brightly burning candle, what we are doing with our data is looking at all the reflections surrounding it.
Because the reflections are externally visible, they're not on their own or any one reflection on its own is not enough. To decide, great things are happening within the company. But if there is bright light being reflected all around by the startup, then usually it means there's something really fantastic happening.
Richie: I like the idea that it's trying to guess what the candle looks like just by looking at the reflections. That's a cool analogy. So, I'd like to talk a bit about the macroeconomic signals that you mentioned. Because we've had A few very strong signals over the last few years. I mean, there was the pandemic that was in 2020, 2021.
And the last year was all like, Oh, inflation's here and maybe a recession is going to happen, which sort of, at least in the U S didn't really happen. So what's going on this year? What's changing?
Madhu Iyer: think the one thing going back to your comment about, the macroeconomic and know, conditions and how the data picks it up and COVID what is really fascinating to us and what has been fascinating is that the data never lies within about a month of the world being locked down and actually even just kind of going into it, you know, within a couple of weeks.
data just completely dropped all travel tech companies from the platform, you there was just so many you know, and the delivery companies just started peaking. And so, so our data was very, very responsive to COVID. Very, very quickly. and also our data, frankly, has been very responsive to Jenny.
I at the moment Jenny, I was a trend that got picked up very early as it was starting a of years ago the data started showing a lot of that. what is fascinating for us is that, you know, Globally, some, economies that are thought to still be robust.
You know, and I don't want to get too much into this, but there was a lot of activity in Africa, for example, there's not much activity there anymore in our data. Southeast Asia was one which was really peaking in 2017 through 2020. I'd say Southeast Asia is now not peaking anymore.
There are, there are many interesting observations just globally that we've had about the companies that have peaked and now not are peaking anymore in terms of regions in terms of sectors. I said, Gen AI but there are a few other things that are very interesting right now. Industry 5.
0. there's a couple of investments that we've made that we can't talk about yet. But there are telling trends of a B2B SaaS emergence that's happening all over the world. the requirements have changed. The way of doing business has changed.
Gen AI has also gotten a lot of other ancillary industries sort of coming up to support it. so we're seeing startups and so, one thing I will say is that the macroeconomics that we don't usually, we don't do much with this currency, GDP, you know, level things.
We don't like to touch that because I think there's some innocence that's lost once you start going in. You know, we are, we are startup investors we want to sort of work in the ecosystem, blossom in the ecosystem and, take some risks that the data is going towards in a way that's not completely suppressed with, currency risk and other things like that.
We don't, we don't look at that.
Richie: That's interesting that you can see that some areas have peaked. Is it also possible to see which regions are up and coming? And are you able to talk about any of those?
Sailesh Ramakrishnan: Just wanna add as part of MAD's answer, but what we are seeing in, in the US in the US we are seeing a sort of. Three different trends coming together or three different movements coming together. think it's almost irrefutable that Gen AI explosion was very much centered here in the U.
S. yes, there are companies outside of here, but I think a lot of the excitement of what happened in JNI was centered here in the U. S. And that has given a lot of people a lot more confidence in the U. S. being a leader in taking this technology forward. And so it has its own sort of side effects about how this technology will be used where it will go.
So you are going to start seeing Wave 2 and Wave 3 companies from the original Genii Explosion where people who gained the deep insight and the confidence are taking back forward. The second again here in the U. S. Are sectors that were traditionally not significantly sort of, in the forefront, but now are coming significantly because technology has gotten to a point, but also entrepreneurship has gotten to a point where the companies in these spaces can be possible.
First and not to over abuse the word, it's Spacetech. there is, the wave one of Spacetech was SpaceX, maybe. And wave two of SpaceX were maybe Blue Origin and other very, very sort of wealthy entrepreneurs starting companies. Today we are seeing startups, having the ambition to build everything from satellites to remote sensing, drones to data platforms to consume a lot of that data to even defense tech and offensive weapons.
these are sectors that here we are seeing in the U. S. that are surging forward. And then the third is a wave that is just beginning, but we, I think will become significant. And maybe there's, a little bit of potential impact from politics and changes in administration potentially or not, but, but this wave has to do with the investment that is currently happening within the US, whether it be semiconductors, whether it be logistics and supply chain, whether it be infrastructure the infrastructure support the various inflation reduction act infrastructure investments that have been happening in the last four or five years.
They are leading to lot more activity in sort of traditional sectors, manufacturing, logistics, supply chain, e commerce. as I think Madhu mentioned, B2B SaaS, while it's a global phenomenon, is significantly surging here in the U. S. as well, because all of these changes require whole different class of software to be created, built that can talk to each other and so on.
Madhu Iyer: Is climate tech. Climate tech is something that we don't talk enough. And then there's a lot of ancillary to climate tech as well but that's been, I mean, I think no, no conversation is complete without sustainability, which. You know, which sort of overlaps everything almost.
Sailesh Ramakrishnan: And in terms of geographical prediction, our algorithms actually started noticing a surge in companies and startups being nominated from areas that traditionally didn't have many. And one of the key examples is Japan. I mean, now we are hearing a lot more information about the macroeconomic environment where Japan has come out of its stagnation.
The market is finally, the stock markets are finally about what they were from the 90s, but we were already seeing a surge in startups that were coming out from Japan. So from a global perspective, three regions where we were seeing a surge and one we are particularly excited for are Australia New Zealand, Japan and Korea and all those we are seeing a significant, surge for Global, companies as in companies based in australia and new zealand, but building for the global, market getting a significant upswing think also because of the success of atlassian and canva and and sort of leaders in that environment but also because I think the world is now gotten to a point where the ambition proof is there that you can come from Australia, from Melbourne or from Sydney and go build a really global scale company that has been proven at this point.
And I think it's leading to a tremendous growth.
Madhu Iyer: One thing I will say is that accidental global investors, that's what we were, you know, we started in the valley, we still only have headquarters in the valley. But it was the data that realized that you know, you can't just sort of being this 50 to a hundred mile, a little, you know, microcosm and just be thinking that the world sort of the sun shines and sets here and that was not true.
And and the algos predicted that way early and, how true that is. With so many, many ecosystems, unicorns, and really leaders off the next generation being formed outside the valley and Europe is something again, I cannot stress enough on for any European founders and, you know, other practitioners in Europe listening, we are super excited about you.
We've always been and to see that some of the most sort of generational companies formed out of their it's sort of a path on the back of the Algos that recognized Europe as sort of a vibrant environment. Way back when, although, you know, the ecosystem, of course, is much different than the U.
S. and we are the first ones to recognize that. You know, and much more about that. That's in a podcast in itself. The European ecosystem versus the U. S. ecosystem. But, you know, it's just really, really heartening to see the data sort of going very, very firmly towards Europe. And, and we are talking about A.
I. and data. some of the most transformational companies have come out of there. along with open AI, it's alongside and that's it's fantastic.
Richie: Absolutely. I do love that data and AI is really a global thing now. It's not just Silicon Valley. And I have the same thing living in New York. It's very easy to become insular and say, Oh, well, something happened in Queens. That's a long way away, but actually, the world's a big place. So there's lots of exciting things happening.
to look out for investments in like I said, B2B, SAS, climate tech, space tech, and infrastructure, it seems like we've got all sorts of areas of regions that were up and coming as you've got Japan, Australia, New Zealand, Korea, I'm assuming South Korea, I think North Korea is not great for investing in, but yeah, you must be taking a lot of calls at weird hours then if you're based in San Francisco.
So there's a lot of talk that generative AI might be in a bubble. How likely do you think that is? Silence. you want to take this?
Sailesh Ramakrishnan: I certainly think it is in a bubble, but it's in a bubble in a different sense from what traditionally we think bubbles are about. bubble aspect traditionally is applied to irrational exuberance or euphoria about something that isn't really fundamentally going to live up to that particular.
That is not the case with Gen AI. This is actually a transformational technology, and it has been years in the making, and we are lucky that it is exploding you know, widespread in front of us. That said, there is a bubble with regards to the potential valuations how we are addressing impact and how we are going to pay the these companies.
That, I think, is at a different level of We are seeing companies with just an idea and a slide deck. Incorporating Gen AI, suddenly getting valued at tens of millions of dollars, which I find very much reminiscent of 2000s where at that time, the buzzword was Internet. Anything that had Internet in it was valued by crazy.
Anything that gets marked up with JNI is marked up as crazily valued. So to separate out the answer again very clearly, the technology and its impact that have on us is not a bubble. Certainly not. And in fact this is actually going to be the change you're going to see in the next decade or so.
However, currently we are in a bubble with respect to companies and their valuation. And the expectation about how quickly these businesses would deliver returns and value. That, I think, is certainly in a bubble. I cannot in any reasonable either investor or mathematical way justify how these numbers are arrived
Richie: That's really interesting that you distinguish whether the technology is in a bubble, whether specific companies are in a bubble, and actually maybe there are a few companies that are a bit overvalued, but the technology as a whole is going to deliver. So, we've seen a few examples of wildly hyped technologies over the last few decades. When you think about Internet search in the early 2000s or self driving cars or social media, they've all had their wild hype phases and there's been a lot of innovation and then things have settled down. Do you think the same thing is going to happen with generative AI?
For example, how many more years of innovation are we going to have? Madhu?
Madhu Iyer: I think talking about overhyped technologies there's been many. Crypto is something which is very required futuristically speaking, but, it's obviously gone through its own and there's a huge ecosystem surrounding crypto and everything else, NFTs, and, Everything that comes off it.
One of the other things that I think, was similar was this blockchain but I think that generative AI is like the Internet. It's closest in comparison, a comparable to that is the Internet because it's an infrastructure layer that is required. And that's, going to be transformational.
That has been transformational. That has been to stylish point earlier, has been around for a while. and people have been tinkering with the LLMs are not new concept, and it's very much like the first and the second wave of gen ai. neural networks, for example, now I'm getting into the nitty gritty and the weeds of things.
But neural nets are still powering a lot of things, and before that, there are precursors to that as well, and there's first gen, second gen, there's just a lot that has been additive over the years. Gen AI is additive, and it's going to be additive, it's going to continuously be additive, not just in the application, but everything like the foundational models to picks and shovels it's all going to be additive and it's all going to have like many generations and many iterations of it much like the internet.
so we think it's here to stay forever as it has before as well but just called different things. But now it's in a supercharged phase of its life and of its life cycle. And then there's just much more to come and, we are very hopeful that we will be getting to fund some of these companies because a lot of these companies require capital.
A lot of these projects require capital. The reason we said that application is more interesting to us as VCs is that, look, foundational models. there is a lot. And that is a podcast in itself. our foundational models and do they require data and infrastructure capabilities that only the big fives or the big tens or the big guys can do.
You know, the Googles of the world, the Amazons, are they the ones that are in the best position to build these foundational models? And these income incumbents are too large for any startup to take on. We don't know, the question is out there, but I think at every phase there is next gen stuff just around the corner.
And what was before, you know, people were saying that, look, images and all that, like first let's do text, then we'll do images, then we will do speech. And, but it's all come within a month, within a few months of each other. So the also just not just that it's here, but the pace of the technology is also really quick now.
Yeah. Everything is getting done really quickly. And what we thought was a couple of years away. It's just a few months. And so it is a transformational time in terms of the pace of the innovation and the infrastructure that is already there to support this innovation. So we'll just have to see what next.
I don't think anybody is in really a good spot to predict that, think it's happening way faster than any one of us can fathom right now.
Richie: Absolutely. And that gives an interesting point about the power balance of these companies. So if foundation models are so expensive, then only a very small number of companies can make them. And that's a very different scenario to, it gets cheaper to build foundation models and then hundreds of thousands of companies can build them. so I'd like to switch to talk a bit about the companies who are making use of generative AI technology. So if you are, as a company, considering buying products or services from these, new gen startups, what do you need to consider?
Sailesh Ramakrishnan: that's an interesting question. In fact I was recently asked this question in a slightly different way. I was asked whether companies need a chief AI officer, that's how I see this question my perspective, which is any company needs to have much more broader view of A.
I. And how it would be used within their entire ecosystem. regards to software with regards to data with regards to how even the new I. P. That is generated. How is that reside? And how will that be protected and kept? How would people's personal information not leak out? in sort of how these models are built?
To even liability issues of other data being used in your model and hence getting you the benefit. you saw the, I'm sure the New York Times lawsuits as well as many other publishers lawsuits. So I think there is a single person or a single role that is needed to coordinate a lot of these.
Now in that, it falls into the usual distinct categories of computing, which is, You need somebody who you can provide you with infrastructure. You need somebody who can provide you with a development environment, a testing and training environment. You need somebody who can provide you with layer that allows you to manage the data, whether it be public, private, protected, personal.
And then there is the legal the various other nuanced complexities as well. So at least these are the four different aspects. And so every one of these is a question you have to ask a provider. Like, what is your data policy? how are you ensuring whether my data does not leave your ecosystem?
But more importantly, also the data you use to train the model doesn't expose me to a loss. how will we compute you in the sense of will this be something that I can bring on frame? I'm a, I'm a financial company. My data is one of the most important and secure things that I have. I'm now going to have to let you know.
Your service or product coming to my system, how is that going to work? So from a computational perspective, to a data perspective, to quality and testing, how do I know this thing is working, is unbiased, is not making obvious mistakes that expose? I mean, the Google example of its image generator was one of those almost dull moments in my mind that Did nobody really even test this thing that it can produce these things?
But that's one of the both advantages and challenges of this technology that not everything is knowable. Because this is a generative system, sometimes you may get a different answer for the same question because underneath it, the model and the data has changed. So, this is actually going to require lot more education.
This is not just another engineering software purchase by any stretch. This is going to be something that requires a lot of understanding of, the complexities, and that's why I believe answer to that other question I mentioned, I believe there is a need for a chief AI officer. This is actually a critical need.
Somebody who can understand all of these aspects. Bring it together in the decisions they are making about how generative AI will be used in their organization.
Richie: That's really interesting, the idea that you probably need a chief AI officer at some point in the future to manage all these ideas and different activities around AI. One thing you mentioned was the New York Times lawsuits. I'm wondering, how does this affect what's going to be a successful AI company?
We've talked about what you should avoid, and it seems like having a lawsuit against you is a bit of a red flag, or it should maybe make you be careful about working for this company. Madhu, want to talk about what buyers of generative AI technology products and services, what do they need to be wary about?
Madhu Iyer: I think that's a question that's very difficult for anybody who's a founder or who's been in founding teams to answer an extraordinarily objective way. And so I'll, buy myself some wiggle room there at the offset because, what this is, is that we are at the forefront of sort of, you know, Wanting to be innovative, wanting to, stretch the boundaries a little bit, wanting to sort of, experiment with that sort of a mindset when you try something, and of course, you know, fundamentally, don't think any of us are seeing any company that's at the edge of, wanting to do something nefarious, we are seeing people with an extraordinary good intent, who want to transform and change the world.
And that's the kind of companies all of us are looking at all of us found all of us are surrounded by and I always philosophically like to think that there are more good people than bad in the world. So, you know, let's start with that principle. And that's what we're surrounding ourselves with and that's what we're surrounded with, to be, to be honest, it's not, mine or silacious glory here.
It is the glory of mankind and humanity that everybody's doing good things. But coming now down to the nitty gritty of things, so people have to try and they have to try to push boundaries to solve problems and they have to use what an AI model will sort of, give out Now this is, you know, even I don't know, Mark Andreessen and Kosla had this debate on Twitter and goes on for pages and a lot of responses back and forth.
So, it's a discussion point for a lot of thinkers and a lot of people who have, you know, invested in game changing companies across the world and started game changing companies across the world. So this is one that we are all aware of. And the idea really is. Do you try or do you sort of not try because you're, you're not sure where the solution is going to take you or where the iterativeness of the process is going to take you.
And you know, and my bias is to try. My bias obviously is also to say that, Try it on a problem that is solvable that I mean that requires a solution You know, but I guess I just want to keep it simple. don't have all the answers I think anyone saying they have all the answers is either blessed with something none of us none of us other mortals are or they're just sort of they don't know What they don't know and to sort of try and predict where this goes and there are some clearly bad elements that you can tweak.
For example, if you have an underlying data set that's going to bias you in some way, or if you have cameras looking at people's lives and there is, you know, privacy. And so, so there's some, you know, normal decency decorum. That we all have to sort of put boundaries on, but then there are some other things that we just have to get really comfortable with to say that, are we going to experiment and keep an open mind?
And the answer to that, in my opinion, should be yes. You know, with a reasonable amount of confidence with a reasonable amount of restraints and systems in place to sort of see if something goes all right, we stop it immediately, we should and I think that's where I land is to say that be proactive, be experimental, be open have a good intent, have a good cause but don't be afraid to try.
Richie: I like that. Most people do have good intent in creating good things. So that's a nice positive note. I'd also like to talk a little bit about jobs and careers. Have you seen with this sort of explosion in AI that there are new careers and new roles appearing?
Madhu Iyer: Yeah, I mean, I think the idea of solopreneurs and I'm now taking this back to founders and it's not exactly answering your question. It's a tangential point to your question. were a lot of things that entrepreneurs are required to do, you know, in terms of their sales and marketing and whatnot.
And so they required many founders and. Now you can start a company and you can start many companies as founders using these AI tools. You can, so the idea of a solopreneur is, you know, much more apparent now. so this is actually taking a little the jobs and careers questions to sort of, can founders now start companies successfully just as single founders?
And the answer to that is yes. And from there flows a lot of things, you know, there's been a lot of talk about the fact that, hey, you know, AI is going to come and disrupt careers and jobs. So I love the positivity of the question that it's actually going to create jobs because when the industrial revolution happened way back when, everybody thought that that's it, now there's going to be no jobs.
And in fact, there are more jobs than ever before that have been formed after that. We find also that, you know, Sailesh made a great point about the chief AI officer. I completely resonate. And, you know, he's designated something I'm sure he resonates with a lot of other people that are looking at buying tools that are AI related and whatnot.
But we're also seeing a lot of other gen AI trained engineers coming more on board and there, specialities and hyper specialities within that. and we're seeing that also come up. But I would say that, you know, for me, the solopreneurship is the highlight. And Silas, you should add on,
Sailesh Ramakrishnan: So the thing I would add and the reason why I think jobs are going to change. Significantly, but also for the positive, is I see Gen AI as, at least in the near term, becoming very much playing the role of a co pilot. So not so much replacing as much as being alongside you from a co pilot perspective.
And so I think it's going to make a lot more of us much more capable and enhanced than we were before. And more productive, and that would create more jobs. And Madhu's point about the solopreneur is a clear example of that. Where before I would be worried and not even consider starting a company, now I can.
Now, I stay a solopreneur all throughout my career? No. As I start and I prove something, I'm going to start creating new jobs. I'm going to start creating new opportunities for people. So I think this in a net will be positive. Of course I think everybody in this discussion has to acknowledge that.
With every technological innovation, there is disruption. And so, and disruption causes change, both positive and negative. And at this point, unfortunately, there may be some negative impact. I mean, do we need a thousand percent call center for customer support anymore? Perhaps not. So I think there will always be disruption, both positive and negative.
But putting all of these things together, I think this change is going to be significantly more positive than previously imagined, because it's going to make each one of us more productive. We're going to feel much more supported and capable having these assistants be co pilots on this.
Richie: Absolutely. I love the idea that being a solo entrepreneur is now a viable thing. So just as a single person using technology and AI assistance, you can actually create a viable business beyond standard, I don't know, I'm a consultant or I'm a plumber or something like that. So that's brilliant. so just to wrap up, what are you most excited about in the world of data and AI right now?
Madhu Iyer: you know, I'm most excited about just if you really talk about, what is data science for me, it's data. And so the, idea that we are now being able to have the transformative infrastructure you know, NVIDIA is not where it is now because, you know, it's, it's no mean feat that they are here just the amount of data that we can consume.
And that we can do something with and that's going to just sort of be exponential in terms of, you know, the potential to store more data to collect and to ingest and to process and to spit out that just the idea of the underlying data just being humongous. and us being able to get more comfortable with humongous amounts of data, the possibilities start there.
And I think for me, that's the most important and the interesting thing. Of course, you know, now everything is generating data. I'm wearing a device that's generating data. And so I'm just most excited about the amount of data collection that's happening in real time. And the ability to ingest it, to process it, to do something with it, to be able to get real time sort of feedback.
and so it's just the amount of data that's collected and that can be stored. That's the most exciting thing for me at a fundamental level. Everything else is just on top of that.
Richie: All right, more data sets, bigger data sets, and arranging all of them in real time. That's the dream for a lot of data scientists. I like that. Silas?
Sailesh Ramakrishnan: so my take on it is a little different. Obviously, I also completely agree that we are in a data, you know, world. Everything is data, and I think it's fantastic. But the difference here is the fact that I've been amazed at what we thought or didn't even understand where languages or data sets are now becoming data.
Like we never knew that genomics was going to be data and now it is. In that along those lines, there are many things that we don't typically think of as data today. that I think are now going to become amenable for, computation. And that's super exciting to me. Everything that we get from space, I don't know if you were aware of this, but we have been running a SETI project for about 20 plus years.
The biggest challenge with that project has not been the data, but the fact that there is so much of it, nobody has time to do any analysis. And so finding a way to convert that to drive insight because now we have super powered NVIDIA GPUs. That is exciting. The fact that we are able to explore frontiers that we did not even consider as places that we can go to today.
Because what we thought of was non doable is now tractable and is changing into, into something that is computable.
Madhu Iyer: I think the one thing that I will say is that it's not In summary, everybody kind of Things that writing great algorithms and machine learners out there just more and more, based to manipulate and deal and build on the data is what is sort of sexy, but the real sexiness is the data itself.
You know, I think that's where all of the information lies. And the more you can get sophisticated in terms of collecting that and doing, doing something with it and processing it, the better. In ways that can be advantageous and, you know, and sort of taken advantage of that's more data is more reliable good data, wherever it comes from, however, you can synthesize it.
I think that's where the superpower of the next generation for a life.
Richie: Okay, I think that between you, you've got a full solution there. So it's like if you have one data silo, Oh, actually you have the ability to analyze all this data you're collecting between the two of you, you've got the whole thing covered. So lots to be excited about in the world of data and AI.
Brilliant. Thank you so much for your time.
blog
What is OpenAI's GPT-4o? Launch Date, How it Works, Use Cases & More
blog
AI Ethics: An Introduction
Vidhi Chugh
9 min
podcast
The Venture Mindset with Ilya Strebulaev, Economist Professor at Stanford Graduate School of Business
tutorial
Databricks DBRX Tutorial: A Step-by-Step Guide
Laiba Siddiqui
10 min
tutorial
Phi-3 Tutorial: Hands-On With Microsoft’s Smallest AI Model
tutorial