How Walmart Leverages Data & AI with Swati Kirti, Sr Director of Data Science at Walmart
Swati Kirti is a Senior Director of Data Science, leading the AI/ML charter for Walmart Global Tech’s international business in Canada, Mexico, Central America, Chile, China, and South Africa. She is responsible for building AI/ML models and products to enable automation and data-driven decisions, powering superior customer experience and realizing value for omnichannel international businesses across e-commerce, stores, supply chain, and merchandising.
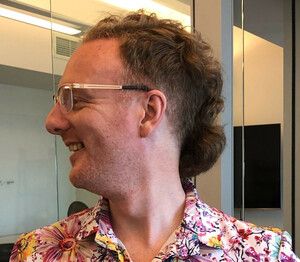
Richie helps individuals and organizations get better at using data and AI. He's been a data scientist since before it was called data science, and has written two books and created many DataCamp courses on the subject. He is a host of the DataFramed podcast, and runs DataCamp's webinar program.
Key Quotes
From a technology perspective, generative AI definitely is the big one where it's really disrupting the way people are shopping, how people are looking for products. People are no more looking for a specific item. They want to have more of a idea or a intent converted into products that are being serviced to them.
In retail, there are many ways in which we can harness data and use it. I think it will be very important to understand how we can use data in a very ethical way, how we put governance on that data that we capture. And even if we are building AI models, then let's make sure that we are very transparent in our approach. It's very explainable, what kind of analytics or AI we're building on top of it. Because that is really important from a customer experience perspective. And I think we should ultimately, retail is all about serving to our customers. So we should definitely keep that going wherever and howsoever we are developing using data in AI.
Key Takeaways
Utilizing generative AI for tasks like intent-based searches can revolutionize the way customers find and interact with products, making searches more efficient and personalized.
Measuring customer experience should include both qualitative and quantitative metrics, such as Net Promoter Score (NPS) and Customer Satisfaction Score (CSAT), to gauge satisfaction accurately and identify areas for improvement.
Building a team with a diverse set of skills, including data scientists, data engineers, and machine learning engineers, is crucial for end-to-end development and implementation of AI products.
Transcript
Richie Cotton: Welcome to DataFramed. This is Ritchie. Data and AI impact every industry, and today we're turning our attention to retail. I want to know all about the different use cases for data and AI to get a sense of how they can be used to improve profits and the customer experience. Since I work for a primarily software based company, I occasionally get a bit of hardware envy.
So I also want to dig into how these technologies can be used in the supply chain for moving physical goods around. On DataFramed, we like to go big, so today I have a guest from one of the world's largest retailers. Swati Kirti is a Senior Director of Data Science at Walmart. where she leads machine learning and AI efforts for Walmart Global Tech, a technology incubator.
Swati is built and now runs a department of data scientists, engineers, and product managers who create AI solutions to improve customer experience and supply chain. She was previously a data scientist at FedEx. Since Walmart is such a huge company, I'll be sure to ask her about scaling data and AI capabilities too.
Let's hear her thoughts.
Hi, Swati. Thank you for joining me on this show.
Swati Kirti: Thank you. Thank you for having me. It's nice to be here.
Richie Cotton: Brilliant. So can you just tell me what are the main use cases of data and AI across Walmart?
Swati Kirti: Yes, I think data and AI play a very important role in the Walmart ecosystem. We have all sorts of use cases around AI, ... See more
Richie Cotton: That's brilliant. So it sounds like it's used almost everywhere. That's pretty amazing. Now since you're a senior director of data science, can you just tell me a bit about the department that you run and talk about what are the main goals?
Swati Kirti: Sure, sure. So I lead a team, a centralized team of data scientists and our main, Charter is to drive a I am a product development to power our international businesses. So Walmart has business in countries like Canada, Mexico, Chile, et cetera. So we as a data science team, we build solutions which are very catered to these markets.
We bring our platform solutions to them, but then also we will very unique to the market. Very, you know, important to the business in the market. How we are able to enable AI for those kind of use cases is all done through my team.
Richie Cotton: Okay. And what does your team consist of? Like, who's actually in your team? And what are the different roles who are using data and AI?
Swati Kirti: We are a team of data scientists and engineers that engineers. So we do end to end product development AI products. Primarily data scientists, data engineers, machine learning engineers are the key competencies that I have in my team.
Richie Cotton: Okay. And how does your team interact with the rest of the business? So, who are the data scientists interacting with in other teams?
Swati Kirti: So we work Close collaborations with product managers, our application engineering teams, business users and UX designers, et cetera. So we basically work in a very four in the box model where we are constantly interacting and collaborating with different leaders in different areas of the business and technology so that we are able to, incubate the right ideas, do the right POCs, iterate, Early on and then build a product, which is, of course, AI enabled, but then very attuned to what business case it's going to solve for and who is going to get the real value out of it.
Richie Cotton: Okay, it seems good that the data team's got a good connection with the business leaders as well, just to make sure that the data science does have some sort of business impact.
Swati Kirti: Yeah, like I said, everything that we build is very geared towards how we are enabling value for our associates in different roles, our business partners, or it's solving for our customer experience making it more frictionless and more satisfying for our customers shopping here.
Richie Cotton: Okay I'd love to get into more detail around that, about how you improve the customer experience. So, can you just tell me how, how does data get used to improve the customer experience?
Swati Kirti: So there are a couple of different ways. I would say for example, on the online channel on our websites, definitely how we curate the information for our customers based on what they're looking for is of utmost importance. We're able to personalize Their shopping experience on our websites, but then we are also able to help them have a complete, Experience in terms of hey if they're leaving this leaving our website and they have missed something that they usually buy We're able to offer them that hey Before you go, you might want to add these things to your cart.
So such kind of different kinds of, personalized shopping experiences is enabled through data and AI on our online channel. But then also in store, you know, we want every customer to have the best experience there. We want to ensure that they are able to find the right products at the right time and the right place where they're looking for it.
Because, stores, stores can be, Very big. So, all that is very data driven and very applicable to the use case of AI on how we are improving those customer journeys.
Richie Cotton: That's really interesting the idea of having personalization and then making sure that people can find the right products as quickly as they can. But it also seems tricky to measure, like a lot of the customer experience feels qualitative. So can you just talk me through a bit on how you might measure consciously these experience metrics?
Swati Kirti: Yes. Yes. It's both qualitative and quantitative wherever possible. We try to be as quantitative as possible. But of course, there is always that qualitative element to every shopping experience. Some of I would say the metrics. Well, there are various Depending on what types of journeys we are supporting in that value chain, right?
Like, there could be various touch points, but then some of the usual ones definitely are things like NPS and csat, which helps us truly understand how satisfied our customers are or how, how our net promoter are touch. But then there are.
How complete the transactions are so that we understand from that that if a person came to shop at our store to define what they were looking for and if They were able to to make Buying decisions based out of that. So those are very indicative of both qualitatively and quantitatively on what are some of the things going well and where there are challenges and opportunities that We should be thinking about improving on
Richie Cotton: I'm wondering if there's a difference between online shopping and in store shopping than some of these things. It feels like online, you can sort of see if someone buys something or not. But in store, it's like, how do you know whether they found the right things or not?
Swati Kirti: Yeah, so that's where the different types of surveys and qualitative aspects come in where we, ask for customer inputs on how was their shopping experience and how would the rate them and things like that. It's definitely more challenging in stores. The brick side of the business is definitely more attuned to not having very precise data like online, but then it definitely is very directional and where to focus.
Richie Cotton: The other thing you mentioned around customer experience was personalization. And it sounds like this is an area where generative AI might be able to help. Can you just talk me through what sort of things you're doing to personalize the shopping experience?
Swati Kirti: I think generative AI is definitely playing a very important and crucial role. And I don't know if the viewers know, but Walmart was one of the first retailers to actually AI search capability on the U. S. website a few months back. And through that, we are able to now offer intent or context based searches for our customers under certain categories.
So, yes, think that's very much in line with how personalization will work over time. Like, it more and more personalization will happen through intent rather than through, very curated, very specific customer journeys. And generative AI is enabling us to do that at scale. It's helping us find different types of use cases around how to not only support customers in what they're looking for to find the right products, but then also offer them, recommendations or offer them different kinds of complimentary products that users might be looking for, but they're They might not really be thinking very actively about it.
So, with Generative AI, we're able to surface those recommendations and again, enrich their personalization journey as well.
Richie Cotton: Okay, yeah, I can certainly imagine that as the number of products you have grows to, like, hundreds of thousands or millions, then search comes really, really hard, and that's where you need all the sort of technological assistance.
Swati Kirti: try out our Generative AI search on app and you can find a lot of things on camping, on birthday parties, and whatnot without doing a lot of search and, you know, searching. It's going through pages after pages. I think like that.
Richie Cotton: Okay, yeah, that's certainly a miserable shopping experience where you, like, The thing you want is buried on page five. All right. So, the other thing you mentioned right at the start was that you make use of data for the supply chain. I have to say, obviously you're dealing with a lot of physical goods and the logistics got to be pretty mind boggling here.
Yeah. Can you talk me through how data and AI are used on the supply chain side?
Swati Kirti: I think supply chain is one area where we are really using AI and data in the best way possible to, for a couple of use cases, to give an example like, of course, inventory planning and management is an important one, and demand forecasting is a very important component. So, how do we use different kinds of systems signals to kind of understand the internal and external factors to then make plans on our, how our inventory should look like, where it should be placed.
and the entire supply chain that goes around enabling that right from last mile to middle mile, first mile. So all that is definitely a place where data and, how it flows through at an item level at a geographical level plays a very important role. The other thing I would say is also on and I where Walmart is playing a very important role is how we are helping to optimize the supply chain through how we are packing our trailers, how we are using the most optimal route to get from place one to be et cetera.
And actually Walmart just A few days back, again, launched a product called Optimizer, which is basically a SaaS based product, which any business can use now to optimize their middle mile for best route planning. So think those are some of the use cases where Walmart is really doubling down to have the right product at the right time, but then also do it in a way which is optimal in terms of our supply chain strategies.
Richie Cotton: That's really interesting. I suppose I was thinking of supply chains, mostly about like, well, how do you do warehouses, right? But actually just. Figuring out what order do you put the boxes in the truck in order to pack more of them in. That's going to have a huge impact. So, uh, yeah, lots of subtleties there.
Swati Kirti: and warehouses also important one. I mean that's like, like I said, there are many different use cases where everything is part of the puzzle. Like everything needs to be done right. to get the right experience for our customers.
Richie Cotton: okay. So if you've got lots of different components here I imagine you've got like lots of different data sources all over the place in different parts of this. Does that give you like a data integration challenge? Like how do you manage all the different steps of this together? Um, yeah. Yes data is challenging. And I think working for international business where we are not only supporting one market, but many different markets such as Canada and Mexico. There are challenges around, how data resides in different sources, how it's generated and then how it's captured.
Swati Kirti: But then over time, we are building robust data lake, Strategies where we are able to bring most of the data, if not all into our data lakes, and we are able to then build a solutions on top of it. Now this is all done in a very very scientific way in terms of not just ensuring the data is the integrity of the data, but then how we follow all the all the policies around data, how we keep it very transparent and explainable at all times.
Richie Cotton: Okay, so a few things to manage there, like who has access to data, is it high quality, and is it following policy? A lot of governance issues there, I'm sure. So the other aspect of supply chain that I always find fascinating is around forecasting demand. It seems like a lot of the optimization comes from just having the right number of any given products in your warehouse or in the right place at the right time.
Can you talk Be through like what sort of tools or techniques you use to make product forecasts.
Swati Kirti: Yes, there are different types of first of all, there are different aspects where we do these analysis and planning. So there's definitely the warehouse component of it, like what's the inbound and outbound from a warehouse. So because that helps us understand how much Goods will flow from FCs or a DC how we need to plan it on a given day and then where it will go.
So, it will go to a store or another fulfillment center or maybe directly to a customer's home. So, there are different channels that we have to work through to ensure that we have again, the right inventory to support all them, but then also have a good understanding of when the goods needs to go out so that we are able to replenish in stores or, even ship it from directly from our X season DCs.
So different kinds of forecasting techniques are being used here to ensure. again, a lot of different complexities, but then mostly different kinds of forecasting techniques to figure out how we can best forecast demand of a given item in a window of time. There are many internal signals being used where we can analyze things like past trends, et cetera.
But then many external factors are also brought in to enrich our modeling, the AI part of it, where we can build algorithms which are very attuned to these signals and are able to do a better job at forecasting.
Richie Cotton: Okay. And what happens when things go wrong? So I think like must be unanticipated events happening all the time. So, how do you deal with this? Cause you can't predict the future.
Swati Kirti: Right, right. Yeah, I mean, I think I think covid was a big example of that. Like you cannot predict these things and things can go wrong. And definitely that's why we have a lot of very smart people looking at this data and, making these decisions and putting in their feedback into the systems in a very data driven way.
So we have a kind of a reinforcement learning going on where we learn from you. didn't go as planned and to basically make it more aligned, more att to to the demand, which should actually be captured
Richie Cotton: Okay. So, when you talk about reinforcement learning, are you talking about the machine learning technique or is this like just, you have human processes.
Swati Kirti: as well. Yes, of course there are, you know, like some very, like I said, very business savvy people who understand the market. They understand what went wrong, what, why, course. Inputs are also necessary for us to understand and mimic some of those behaviors, which they from their experience are able to identify and share.
But we now need to have a way to implement it in our models. So we take those qualitative feedback as well, and of course, there is a like a feedback loop, which is more of a pipeline that's built to capture that feedback, and then our models are fine tuned or retrained on those as
Richie Cotton: Okay, so you're taking whatever real world or business events happen and then you're feeding those back in to improve your models
Swati Kirti: well. Wherever possible, if, we know, like, like a holiday is coming in, and we know something will will be sold more than. Time then yeah, we can definitely use that kind of signals to enrich our forecasting signals.
Richie Cotton: curious how much of the work around data you do is sort of generic and how much is specific to retail. So are there any challenges around working with data and AI that are really retail specific?
Swati Kirti: Good question. I don't think anything we do do is generic, to be honest, because we're , we're always solving for the next big problem and and I think all are geared towards, providing that, holistic and frictionless experience. So I don't think I'll categorize anything as generic.
If you're asking more in terms of what I would categorize as like highly sophisticated algorithm versus not so much. If, That's what your intent was then think We try to match the solution to the type of problem. Like if a problem can be solved by something analytics, then we don't need a deep learning Algorithms to be built to go and solve just that because we want to but yeah There are challenges where we really need to go deep.
We really need to build more sophisticated algorithms so that Because that problem is needs that. So we definitely have that kind of approach. It's not about over engineering anything. It's about finding the optimal way to solve for a problem using what we have. So, that's the approach I would say we, we take again, nothing generic because everything itself is a big problem.
And something that at one solving at Walmart scale is a Another challenge to go and work to work towards.
Richie Cotton: Just to follow up on that what helps you scale things? I know you do it like everything has to be gigantic scale. Are there any sort of tips or tricks you have for scaling your work with data? for scaling, we definitely have seen that learning more about machine learning techniques, machine learning engineering in itself becoming more and more important where we, it's not just about building the right algorithms, but then how do we take it and roll it out in production, which can then, you know, make it more efficient.
Swati Kirti: Serve millions and millions of customers in a way that is providing the best experience in terms of being real time or near real time but then also helps us manage our resources like compute resources and our in the amount of effort that goes into supporting these capabilities So it's definitely a challenging at the scale.
But then I think machine learning engineering is becoming more and more pertinent as we go roll out more of these air solutions.
Richie Cotton: I think one of the biggest complaints I've heard from people working at large companies is it takes longer to do things and makes it harder to stay cutting edge. So, since Walmart's still about as big as it gets have you got any advice for how people at larger companies can stay up to date with their technology, with their data processes and capabilities?
Swati Kirti: Yeah, I don't know if you saw that, but I think it was yesterday or the day before yesterday. Walmart was named as the fifth most innovative company in the world. And we came and this is in comparing ourselves to Google or Amazon, Microsoft, et cetera. So, I think it very much tells you that even at scale being very relevant in terms of the next step.
Technology can advance as possible. And with generative AI search, I think Walmart was one of the first in the market to do that. So, I would say that is definitely hard, but I think Good and focused intention of, being the best in technology in retail we are able to do that and we are able to bring in the best in class technology to Walmart and then build solutions which are Best in class for retail.
So don't see that that to be a challenge where I am and in my team.
Richie Cotton: Now, I'd love to talk a little bit about skills. So, within your team or across Walmart more in general what are the most in demand data and AI skills?
Swati Kirti: the most important skills I would say is definitely those for data scientists and machine learning engineers. So skills like data analysis, statistical analysis, predictive modeling good coding experience with Technologies like Python or et cetera, are definitely very relevant. I would also say like there is a lot of, these no code or low code ai tools that are coming up in the market and a lot of things can be done using those tools, but I still feel like having.
Very solid coding background, critical thinking, analysis experience will go a long way, just because it can enable you to build a more, many more bespoke solutions, which these tools might not be able to do that. So I think those are some of the skill sets I would definitely. They are very critical.
Richie Cotton: you mentioned a few different sort of tools and techniques. I guess because you've got so many people working with data at Walmart there must be a sort of variety of things going from like one team to another. How do you like synchronize skills and synchronize like tools and things like from one team to another?
Is there any attempt to do that?
Swati Kirti: Absolutely. We do have like every Organization have their hiring, standards like, for this kind of role I want We will need this kind of a skill set or this kind of a profile of a candidate. So those kind of I would say normalizations happen, especially like if I'm meeting a team, I'm meeting multiple teams, but the skill sets needed in different teams might be different.
People who might be building search and personalization solutions might need a different skill set versus somebody who might be focused on building more demand planning solutions. So those kind of variations do exist. But at the same time, having a A good benchmark of, what are transitional skills versus what are very specific skills for the job helps us normalize it across different teams and different organizations.
Richie Cotton: Are there any skills that data professionals need like, to work in such a large organization? Like, I imagine it's a very different vibe to working at a startup.
Swati Kirti: Yeah, yeah, would say, you know, like I mentioned, those two tools like Python and learning algorithms, predictive analytics, those are definitely some of those I'll put them as more transitional skills, because you know how to Work on them, then you can pretty much apply them to a lot of different solutions.
But then I think for companies like Walmart, I think a lot of critical thinking making sure you are really a problem solver you're able to communicate these complex concepts very well to a large And a very different set of audiences who may or may not speak the language of a data scientist is very important.
it's ultimately about creating value. So if you're able to articulate your work in a way that is understood by most people out there, then I think that is definitely something that an advanced Technology should work on, especially in the data science and AI space, because things get really complicated here.
So how do you make sure that you're able to represent those complexities very well to others is very critical to how you make them understand what you're doing.
Richie Cotton: It seems like, communication skills, whether you're a very important, whether you're talking to a technical audience or a non technical audience. Is there anything that you think is helpful for being able to navigate a large organization?
Swati Kirti: think just be a confident technologist. I think if you know your what you do and you have you're very clear about what your strengths are, then think it's no different than any other place. It works in a very startup mode. We are very aggressive about how we roll out new products and solutions.
So, I don't think it's going to be any different, but It depends on how self assured you are in your own technical skills.
Richie Cotton: I'm curious about if there are any innovations that are happening with data or AI in the world of retail. So, what are you most excited about right now in this area?
Swati Kirti: I'm really excited about how, course, the generative AI that I mentioned earlier, like, that was just one example, but we are doing a lot of innovation within every other aspect of retail, in different domains, in different problem areas where we are looking at different ways to again use generative AI to either provide automation, get to better insights or to just enable better associate our customer journeys in their processes, whatever they are working on.
So that's definitely one area where I'm excited, but I think I'm also excited about the fact that we're using really machine learning and I do actually solve for some problems, which I think I've been very passionate about. Like, one of my teams is really solving about how we reduce waste in our stores using AI.
So we are actually looking at waste metrics of if you walk into a Walmart store, there are a lot of things that are being produced in store, like pizzas and chicken and bakery items and whatnot. So how do we use AI to basically ensure that we are, producing the right optimal quantities at any given time.
So we're not able to just provide fresh products to our customers who are walking into the stores, but then we're able to reduce our waste because that helps us to, really work towards better environment, sustainability goals, and which are, I think, very long term and something that we need to solve for now so that we see the value in the future.
So, all innovation. might not be the most clear problem to go and solve for, but then applying AI to actually go and solve for these problems, which will make a long, term difference, but also help our customer experience. Those are the innovations we're talking about, and we do that in many other areas of the business.
Richie Cotton: So that actually sounds like a very fascinating problem, reducing waste, because it's going to help both the customer, because they're getting precious
Swati Kirti: Fresh fish, yeah. Walking, yeah.
Richie Cotton: And then it's helping Walmart, because you're wasting less money on throwing things out. I'm curious to see how you can go about, like, measuring that.
Is it like someone just going, just, weighing the trash that gets thrown out every night or something. Like what, what goes on there?
Swati Kirti: Very good question. I don't know if somebody actually measures it, but there are different ways. Like, for example we do look at our sales metrics on, okay, how much did we produce? How much did we sell? And was there a difference? Because that is an indicator if you're overproduced or underproduced, right?
Like, not under, but overproduced definitely. Like, we baked more cakes than we were able to sell. But then yes, there are different measurement techniques and there are different ways in which stores measure their throwaways. So we are able to capture that data. Now, all of it might not be attributed to just how much we produce and store.
It could be due to other things as well, right? Like, just something, not of good quality or whatever reason that could be. So we try to attribute what we can to waste due to fresh. But then we have some data as well on how much should we really produce on a given day. And we have other information like seasonal products, et cetera.
So we know that we were going to probably sell more during certain times or less because of weather or whatever. So, we do bring in those signals again as well to make it very informed forecast. So that our associates in store can use it to actually go and produce those units.
Richie Cotton: Okay. That's like, all the people who buy the leftover chocolates, like the day after Valentine's Day or the day after Easter.
Swati Kirti: Yeah, hopefully, hopefully, they find the freshest products.
Richie Cotton: Nice.
Swati Kirti: Yeah,
Richie Cotton: right. Are there any other sort of trends or things that you think are changing the world of retail?
Swati Kirti: from a technology perspective, generative AI definitely is the big one where it's really disrupting the way people are shopping. How people are looking for products. Basically, people are no more looking for a specific item. They want to have more of idea or intent converted into products that are being surfaced to them.
So I think that is definitely going to be very revolutionizing because it's about again, the customer experience, but also helping them find the most relevant product as quickly as possible. So I think that will be really disrupting. But yeah, I mean, retail is a fascinating space. And I'm having the privilege of working in different markets.
So I can, I see how Canadian customers are different than Mexican customers and then customers in South Africa. So, think it's an ever changing it's going through its own evolution and it's an exciting journey.
Richie Cotton: Okay. That actually sounds fascinating. you able to share any insights on, like, what the difference between a Canadian Walmart shopper is from a South African Walmart shopper or? Vice versa.
Swati Kirti: Yes. I think everyone is there in their own journey of Adopting technology to shape their shopping experience. So like a canadian Customer would be I would say much attuned to a u. s Customer in terms of how they are, using online apps to shop versus actually going to store or how they're using our newer offerings like pick up in store and deliver in store things like that mexican customers we operate through different, Banners over there.
So again, we need the banners. We see a lot of variations in what shoppers are really looking for. What are their preferred methods of shopping with us or interacting with Walmart and then South Africa is, and it's an old journey of having their own different banners operating different African countries.
So they'd also have their own. Differences in how personalization works for them or how they would want to get their products to them either in store or online. So, I mean, there are different kinds of uniqueness that each market has, and it's just really very fascinating to learn and to build products which serves the wider need of all these different markets.
Richie Cotton: All right, wonderful. Just to wrap up, have you got any final advice for people working in retail on how they can make better use of data and AI?
Swati Kirti: in retail, there are many ways in which we can harness data and use it, but I think it will be very important to understand how we can use data in a very ethical way, how we put governance on that data that we capture, and even if we are building in models, then let's make sure that we are very transparent in our approach.
We're very, it's very explainable on what, kind of analytics or AI we are building on top of it. Because that is really important from a customer experience perspective. And I think ultimately, retail is all about serving to our customers. So we should definitely keep that going, wherever and howsoever we are developing using data and AI.
Richie Cotton: Okay, I like that. Focus on improving the customer experience and helping your customers. That seems like a good moral. All right. Thank you very much for your time, Swati.
Swati Kirti: Thank you.
blog
What is OpenAI's GPT-4o? Launch Date, How it Works, Use Cases & More
blog
AI Ethics: An Introduction
Vidhi Chugh
9 min
podcast
The 2nd Wave of Generative AI with Sailesh Ramakrishnan & Madhu Iyer, Managing Partners at Rocketship.vc
podcast
The Venture Mindset with Ilya Strebulaev, Economist Professor at Stanford Graduate School of Business
tutorial
Phi-3 Tutorial: Hands-On With Microsoft’s Smallest AI Model
tutorial