Behind the Scenes of Transamerica’s Data Transformation
Vanessa Gonzalez, Senior Director of Data and Analytics for ML & AI talks about leading Transamerica’s Data Transformation program. The biggest challenges and important success factors for large-scale transform
Vanessa Gonzalez is the Sr. Director of Data Science and Innovation at Businessolver where she leads the Computational Linguistics, Machine Learning Engineering, Data Science, BI Analytics, and BI Engineering teams. She is experienced in leading data transformations, performing analytical and management functions that contribute to the goals and growth objectives of organizations and divisions.
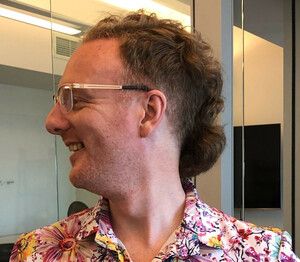
Richie helps individuals and organizations get better at using data and AI. He's been a data scientist since before it was called data science, and has written two books and created many DataCamp courses on the subject. He is a host of the DataFramed podcast, and runs DataCamp's webinar program.
Key Quotes
Customers want to be able to see the data when they need it, and they want to have a better digital assets and interactions with us. That's where they see the results of our transformation. They will not know why, but suddenly the website works faster, or that calls are being routed in a better way, and the truth is that they really don't need to know exactly where or how the data is is going from point A to point B and why it's taking longer or shorter times in order to still experience the benefits. By going through data transformation and implementing machine learning and AI models, what we really do is improve our customer service and by doing that we are able to grow our business and keep our clients happy.
Organizations should have thorough requirements at the very beginning of the data transformation process to ensure nothing is missed. You have to make sure that you don't skip any pieces when you're putting your requirements together. For example, if you have a project where you're bringing data from many different places, if you forget a couple of pieces, then they won’t be there when you need them, and it will be a lot more difficult and complex to bring those pieces in. Easier when you plan ahead and map out exactly what pieces and transformations you will need and how you will take each item from point A all the way through where it will live moving forward. This is actually a lot easier than bringing 80% of what you need, but forgetting 20% and having to figure out as you go. It is wise to spend the time before you start moving any data to really lay out clear requirements.
Key Takeaways
Data Transformation is a team effort that requires organization-wide support and a collaborative process in order to be successful.
The ability to translate complex technical ideas to internal stakeholders in simple and quickly understandable terms is a vital skill data scientists should continually nurture.
Just as technology and processes are constantly evolving and growing, it’s important for data scientists to cultivate a learner’s mindset, always exploring how to embrace and adapt to new changes.
Transcript
Richie Cotton: Welcome to Data Framed. I'm Richie, and today we're talking about data transformation programs. Whenever I speak to data camp's customers, one of the most common conversations goes like this. Hey, we know we need to get better at working with data, and our C-Suite has finally figured this out too. So now we're gonna do a data transformation program, but it's kind of hard and I'm not sure exactly what we need to do.
So at DataCamp, we spend a lot of time coaching organizations through the details of who needs what data skills in order to modernize their data. I thought Robin have to tell every organization one a time. Let's just hear the story of someone who's been through that transformation process and tell her war stories.
Today is Vanessa Gonzales, the senior director of Data and Analytics for machine learning and artificial intelligence at Transamerica, as well as helping Transamerica through their data transformation program. Vanessa is also a senior data manager, so I'm expecting some great leadership insights.
Hi Vanessa. Thank you for joining me today. I'm very excited to chat about what you've been getting up to at Transamerica. So first of all, maybe you can just give us a bit of context about what does Transamerica.
Vanessa Gonzalez: Hi, Richie. Thank you so much for having. So Transamerica, it's a financial institution. We do a retirement, we do employee benefits. When you hear about a company that, for example, when you start working there and they offer you a 401k and they offer you some benefits that you can choos... See more
And then also Transamerica on the other side of the, of the coin, can sell some products and annuities benefits directly to customers. So we do a little bit of. We're really well known for the retirement side of it, but also we're getting into a lot of of other products like employee benefits and insurance.
Richie Cotton: Wonderful. And so your job title is Digital and analytics for ML and AI. So maybe you can just explain a bit more about what your team does.
Vanessa Gonzalez: So I have a team of data scientists, and also I have a business system and analyst in my team, and I work very closely with engineers, with architects. But really what we do is that we. Figure out how can we help our business there? It's very exciting and there's a lot of different topics, and there are a lot of different ways how we do it.
But what we do is that we, we use machine learning. We use AI to create more value to our business. We help them solve problems, and we make sure that by doing that they can do their work better. And also they, we can be better with our customers and, and get them to have better service.
Richie Cotton: Are there any particular business problems that your team's been working?
Vanessa Gonzalez: Yeah, so we work in many different things, and that's the fun part of our job is that it's never the same. So if you ask me today and you ask me a year from now, the projects are gonna be completely different. But to give you some ideas of what we do, we focus on four different areas. So everything that we do is to increase retention for, for customers or to create growth.
So really grow our business or improve customers service. So it could be from the call center or it could be on how we do processes and how we automate certain things. Like reduce the, the time that you wait on the phone, for example, or, or if your call is routed, is routed to the right place. And we also try to decrease costs for our business.
So depending who we're working with, we're gonna be doing different things. Everything we do is gonna have a machine learning model that is gonna drive these predictions that help our business, and then we integrate them into our systems that we already have. Let's say one example is if, if we want our advisors to know who would be a more probable person to be retained.
We help them by giving them a prediction of, of these, and then they can call this person and, and, and talk to them and figure out how can we help them with the, with what the issues or problems they may be having. So that's the type of thing that we do. And we do a lot of other models as well for prioritizing.
So for example, if we wanna know which claims may be fraudulent, we can see, okay, these top 10 are the ones that look like more like fraud. So we can do models for that as well.
Team Structure at Transamerica
Richie Cotton: That's really fascinating. So you mentioned that you have some data scientists and your team data architects and engineers. So perhaps you can tell me how all these people work together. How are your teams structured?
Vanessa Gonzalez: Sure. So my direct team were more data scientists and business analysts, but we work very closely with the data engineering team, with a third architecture team, with a, a BI team. So the, the way we do it is that, as we always say, that, Machine learning is a team sport, so you need to collaborate with all these teams to make it work.
So you're gonna have three pieces for every model that you're gonna build or every solution that you're gonna build. You're gonna have the piece where you bring the data in, and then we, we need the architects there and the engineers to bring that data into cloud, make it available for us to access it.
Then we have the data scientists in my team, they're gonna be developing those models. They're gonna bring the data. Manipulate the data. They're gonna be working with it, training the models, developing them. Once they're ready for deployment, then we need to tie to work with the DevOps team to make sure of how we're gonna deploy the solution.
We need to bring that model from development all the way to. Promote environments all the way to production. And then there's another piece to it. We need to integrate the results of these models or the output of these models into solutions or applications. So could be Salesforce, it could be just a table in Redshift on the cloud.
It could be other solutions like Call Miner that we use also for the Kohl Center. So depending when, where we want the output to be. Then we're gonna have to work with them and that we were, we need engineers again, DevOps and the architect team to the architecture team to help us out. So that's where we, how we interact.
So we may not have everybody in the same team, but we have to work with all these teams to make it happen. And of course, the business, that's the, the most important piece of, or, or the most important team, because we're really trying to, Have them explain what they're dealing with, what problems they're having, and they help us through the process also to get feedback of, of what the results that we're giving them.
And then we tune our models and then we're able to, to do a little bit more there.
Richie Cotton: it really is like a lot of different teams involved just to get answers to these data problems. It's not just data science working in isolation. I like that
Vanessa Gonzalez: Exactly. So when you think about a data scientist, if you think that they're gonna be working just to hiding in a room, doing their thing, Well, not really. They need to have a lot of communication with other teams. They need to have a lot of collaboration. So for a da, a good data scientist is gonna be somebody that, that loves to collaborate, that loves to work in a team environment.
If not, they're not gonna be able to develop the same quality models that you could if you integrate with all these teams.
Recommended Skills for Data Scientists
Richie Cotton: I think that's, that's really useful advice there. Do need those communication skills. Actually, maybe just a continuing on that thing. Are there any particular skills like communication or are these other kind of softer skills that you think are important for data scientists?
Vanessa Gonzalez: Yeah, definitely. So one skill that it's not easy to find, and it is very, very important, is not just knowing how to communicate, but also knowing how to translate. The very technical to the more everyday type of work because you're gonna have to be working with B with business people that have never seen a model or they don't know how it works.
So you need to be able to have that communication going back and forth and understanding what they want to tell you, but also being able to share what you're finding and what you want to tell them in the same language. That translation, it seems that it's easy, but it's not that easy. Sometimes you have to explain a model that is very, very complicated in a very easy way, and sometimes the business has to explain their processes that maybe for them very obvious to data scientists that they have never been exposed to them, so it's not as obvious as that one would think.
So that communication skill, definitely important.
Richie Cotton: Do you have any success stories where that's been done really well in your organization? Or any maybe some disaster stories where it hasn't worked so well?
Vanessa Gonzalez: No, definitely. So for data scientists, you know how we always say, Oh, well this is the recall and this is the precision of of our models. Well, that doesn't go very far with the business because they don't know what recall is or what precision is. Or if anyone we're talking about accuracy or an F score, what are we talking about?
So I have a data scientist in my team that he's awesome on. That communication with them. So he's able to say, instead of using really the data science terms, he's able to tell to the business. And in this case it was a, a model that had to do with natural language processing. And we were talking about how many, like, how the model was identifying topics on a call and there are transcription.
So he was able to really explain to the business on. How accurate was the model? By using some easier terms, like, like saying okay, of every a hundred calls the model will be able to tell us 20 times correctly what the topic is and then it would not be so sure in another 20 ti 20 times. But in five times they was well, so they were, he was really able to explain.
What we were trying to say with the results of the model or the, like, the metrics of the model in a, in a way for the business to comprehend and say, oh, 82% of the times guessing the topic is good for us. It's even better than what we get from our own people doing it. So we're very happy with that number.
And then we were went from, that conversation went from there. So that would be a time that really worked out well. We have tried in another times when we go and just give the metrics and. Get a lot of silence in the room. So that's when you know that you have to explain in a different way for everybody in the room to understand what we're trying to say.
And y'all, they're not supposed to know machine learning. So we have to be able to say, what value are we gonna add by doing it in the terms and in the way that they're more. So that's always an interesting conversation, but you learn it and you get good at it, and by practicing and paying attention, you can really get that translation really to a good place.
Richie Cotton: That does seem so important. I think like one of the points you're making there is just, if the business people don't understand what you're talking about, then it's gonna have no impact across the rest of the organization.
Vanessa Gonzalez: Exactly.
Data Transformation Program at Transamerica
Richie Cotton: All right, Wonderful. So you've been part of a, a big data transformation program at Transamerica.
Perhaps you can just tell me a little bit about what the goals of this data transformation program.
Vanessa Gonzalez: Yeah, definitely. So when we talked about data, we need to, as time goes by, we need just like a lot of data, but we need to access it in a, in an easier way. We need. Quick access to it. We need to be able to find the data in one place and we need to make sure or know that that data that we're gonna be using for whatever we're gonna be using it for, that it's accurate, that it's complete, that it's timely.
So Transamerica, we we're a company that has been around for many, many years more, I think more than a hundred. And it has been also, it has been formed from acquisitions and it has grown in many ways. It has been restructured many. So we have many sources of data and we need to make sure that we can access all the data that we have.
Also, think about a company like ours that we do retirement. If you have somebody that starts their 401K when they're in their thirties, They may not start using it until 30 years later. So you have customers that have been with us for 30 years or for 35 years, and that means that we have to keep all the data, all the transactions they have done in their plans through that time or in their, how, what if they have been maybe married and then divorced and then they had kids.
And all the beneficiaries for them have changed through time. So there's a lot of data. So what we are doing with the data transformation is really moving all our data from on premise servers and to on cloud, and we are trying to modernize ourselves to make sure that we have all the data in one place, that all that data is curated, that it's accessible, that it's really.
Well monitored for security as well. We wanna keep our customers protected. We don't want their data just floating everywhere, so we have to make sure that we do all these things. So by doing the data transformation and the digital transformation, it allows us to be a lot better, more careful, and user data in a better way.
As we move our data into cloud. We also make sure that the quality of it is there, that we're looking at how we're using. That if we have the same, uh, somebody's record in seven places, that we know that those seven records of that person are the same person. So we're doing mastering and identity resolution there, and most of all, we are trying to have the data available and insecure for our customers.
So that's just some of the examples of why we're doing the data transformation. But as you can imagine, it's a huge project and it's a very exciting one for.
Richie Cotton: Absolutely. I mean, I think about the data. We at Data Camp and the company's been around for, well, almost 10 years at this point. We already have data from so many different sources in so many different places, so, What you were talking about where someone's got a life insurance policy or retirement policy and you've gotta manage the data integrity for 30 years before they even start using it, then that does seem like a huge challenge.
So can you talk to me a bit about like, where have you got started with this programs At the beginning, you have data in all different places and you're trying to curate the data. So what, what was the first step with.
Vanessa Gonzalez: So the first step in that was started even before I started in Transamerica, that we started thinking about, okay, what do we need to do to be a more modern. To get our, uh, to keep our, our data safe, to put it in one place and in the right place. So that like the, the first thing is, is making the decision of this is what we want.
This is important to us. This is gonna be part of our strategy. Then from there, then we start to thinking of, okay. How are we gonna do this? Because it's huge. It's a huge project. It's not something that you can get done in a day, and it is not something that we can say, Okay, everybody stop everything they're doing.
We're gonna wait for one year or two years while we do it, and then we continue business. We have to keep the business going. Right? So you have to keep those both things happening at the same time. And that's also tricky, so, So the second piece, like first you start the strategy, you start thinking about how you're gonna do it, and then the first step to do it was, Creating that architecture, that foundation, that, uh, like the little boxes where you're gonna put this stuff, right?
So you have to figure out what is gonna be your architecture in, in cloud? How are you gonna, how are you gonna do it? Are you gonna bring applications? Are you gonna bring just the data? Are you gonna bring both? In our case, we are doing both. We're bringing, like, the idea is to have in a, maybe in a year or so, we're gonna have everything in cloud, maybe in, in, in between one and two years.
So we have already brought a lot of applications into cloud. Now we're bringing data. We have about 25%, I would say, of our data is already in cloud where we're gonna bring a lot of data this year. We have so much that you have to start thinking as you bring in, Okay, what am I gonna clean up? What am I gonna bring the data from one server and then just shut the server off?
But then how many processes are affected? By moving that data. So just think about reporting. If you move the data from point A to point B, every report that was using data from point A have to be refactored to point B. So it's a a lot of pieces happening at the same time, and you have to prioritize then what comes first, what comes later, and the sequence of how you're bringing in the data and the applications and everything else.
So the first step is, Getting that architecture ready, getting that, that place to start moving things in, making sure that you have the security that you need. How are you gonna give access to that data, to the applications? Like you really start thinking about that architecture. So our architecture team did have an amazing job about thinking about it, getting a lot of knowledge on.
Making sure that the way that they're setting the architecture is gonna work for our company. Because every company is very different. So we cannot just say, Oh, maybe Sony did it this way. We should do it the same way. We have to come up with an architecture that works for us and that is gonna work for our customers and for, for the agents that we work with and the companies that we work with.
So there's a lot of different moving pieces. Once that is, Then you start bringing things in and you start thinking about, okay, how do I bring them in for how long I keep them both, Or in which cases I just move them? How do I test it? How do I give access to these new pieces? And then once we have all that, then you start to have to think about how do I turn off the old and the legacy stuff and just keep the new one. So that's more or less how, how we're planning AB and how we're going about it and how we're doing.
Prioritizing Data by Value
Richie Cotton: So you mentioned talking about like prioritization cuz you need to decide on what order you are shifting your data into the cloud. I'm wondering how do you prioritize. That high value data first, cuz that's the most important or the low value data cuz it's less risky? Or do you do it by team? Or how? How do you think about this? How do you prioritize?
Vanessa Gonzalez: So that's a great question. So how we have been doing it is that we are, at the same time that we're doing our data transformation, we're also doing a transformation to be a better company. We're doing a lot of initiatives we're working on to be better, to sell more, to treat our customers better. So all of those new initiatives, what we're doing is that we're think.
These initiatives are gonna need, uh, data for, uh, one example is we're making our website better. Well, the website needs these type of data, all these pieces, so let's bring those pieces to the cloud. So when we create this new website, It's gonna use data from cloud instead of using data from premise. So we prioritize by the data needed for the new stuff that we're bringing in.
We are doing it all with data from cloud. And then we start thinking about what is the data that we use the most that is used in most systems, in most cases, that it's like very really important to us to report on our. That's a data that comes in as well. So we are bringing in what on the first group of initiatives that we had, we saw what data we needed.
Then we saw, okay, well what's the busiest database that we're using the most? Our retirement database? We brought that in, and then this next set of, for the next couple of years, we're looking at, okay, what are the initiatives that we're gonna be working on in the next couple? What data do they need? What data do we don't have yet in cloud that we're gonna need?
And then we bring that in. And really the data that is used the least, or by the least systems list people, these programs, that's the one that comes at the end. In a perfect world, we want everything in in cloud, and that's where we're heading. But some things are gonna take a little bit. And we have to be okay.
It's a journey. It's not gonna happen in the day. So you have to be patient and you have to keep going and keep at it, to make it happen.
Richie Cotton: That's a very good point. I've noticed that, well, basically everywhere I've worked, management tends to have like, like a short amount of patience for these really long technical projects unless they see some kind of benefit early on. So is there anywhere where you think you've had like an easy win or you've been able to demonstrate some value from this data transformation program kind of part way through, rather than having to wait till the end?
Vanessa Gonzalez: Yeah, no. So we have some incremental value on the way. You're completely right. You have to show some value added because if not, it's, it's just like putting a lot of money into it, and then you don't see any results. That never goes well. So what we're doing is that as we're building this foundation for us of bringing this data in, we're starting, like we have already a couple of machine learning models that we just.
Just use, like all our data is already in cloud. There's other, other initiatives that have happened, like we had a, did some customer mastering and that data, it's already in cloud. The mastering that we produced, there's a couple of other big initiatives that they were related with, with our, our, our website and interactions with the customers, and that was all the data needed for that was also in cloud.
So we've had some early. But we keep going as we go and have some more wins on the way. So the idea is that as we are creating all these initiatives, that's why we prioritize that way so we can start getting value added by having this data already in the cloud.
Richie Cotton: And so with these sort of big technical projects, it can sometimes feel like it's a sort of backend thing that's a bit removed from the customers. I'm just wondering what's the impact on your customers been so far?
Vanessa Gonzalez: So our customers, like they don't need to know or they don't should, They should not care about where we have our data. What they want is having. Good data, right? They have, they wanna have it at on time. They wanna be able to see the data when they need it, and they, they want to have a better, better digital assets or interactions with us, right?
So that's where they have been seeing the results on, on what we're doing. They will not know why, but suddenly the website works faster. Or for example, suddenly the calls are being routed in a better. and they really don't need to know. Exactly where, how the data is, is going from point A to point B and why it's taking longer or shorter times.
But they see the benefit there. So as I was saying at the very beginning, by what we do, and by going through data transformation, by having applications of machine learning and ai, what we do is really. Improve our customer service and then doing that, then we also are able to grow our business and also keep our clients and they keep them happy, right?
And, and reduce cost for us so we can. Pass that along as well. So it's all, all good. . You see, there's no downside other than that. It takes time and a lot of work. I think it's a great thing when companies go through these data transformations, they're, I hear again and again, everybody's doing it. It's kind of like something that we have to do at this point.
We can just stay in, wait, right? We have to do everything we can to be in a better place, and that's what we're.
Richie Cotton: Absolutely. So I'm curious on what the time scale is beyond. It's a long time, like so when did this program start and when do you think you'll be done?
Vanessa Gonzalez: I think it started a couple years ago and we're hoping that it's gonna be done in a couple years, so I'm thinking it's gonna take about four years or, or so there's some pieces that started that they're like, they're starting as we go and then they will end up later, but, I think that's more or less the timeframe from beginning to end.
So it's very cool transformation. I think 19 is when it started and then should be done by the end of, of 2023 or half of 2024. somewhere there.
Richie Cotton: We never know if everything goes to plan the end of 2023, more realistically, a bit later. Okay. I'd like to talk a little bit about the, uh, the technologies using, so obviously you're, you're adopting some cloud tools. Has your technology stack changed at all beyond that as part of this transformation?
Vanessa Gonzalez: Yeah, definitely. So we were using some cloud already a couple years ago. But not, not that much. So we were developing our models for machine learning and, and we were using tools like Domino and we were using Hadoop and Bitbucket. Right now we're, we move to aws, so that's a, the cloud technology that we're using.
We're working under SageMaker environment for machine learning development. So we're using now SageMaker and we're using Redshift and, and S3 buckets. That, those, those pieces there. But we also were using bid pocket, so our tool stack it. Change a little bit. The idea is that as we move more data into cloud, it's gonna be a lot easier for us to run the models that we're running and more and more running them in real time.
Well, now we do batch, we batch brands. So it, it has changed. We had to, to develop a new infrastructure for us because as you can imagine, like every company also has to look into their security and what it, what works and whatnot. So you have to do a mix of what is already out. And then you put your own guardrails and follow those good practices that you have for your company.
So we integrated those and we're super excited because we finished our, our platform and now we're developing there. And more and more we're gonna be able to be more efficient in, in my group. So it's, it's all a really ex very exciting times.
Richie Cotton: Wonderful. And uh, so cuz this is such a huge effort, which sort of other teams have been involved in this beyond just the, your sort of analytics and machine learning teams?
Vanessa Gonzalez: So the data transformation has been a huge effort that the whole company has been evolved. You have from the, our leadership on the business side and on the IT side, our CTO has been instrumental on this. And if you, if you think about like all the teams, With it that they're needed. You need the production teams.
You need the strategy teams. You need the DevOps teams like architecture, engineering, like there's a lot of teams that need to work on this data transformation. Some are gonna work on the how we make the infrastructure. Others are gonna work on how we bring the data, the data. Governance data quality and data science teams are important here.
The business and then business analytics teams are important as well because they have to set up requirements of what they need in this environment to be able to do BI and have reporting. Uh, the business they need to be really involved in supporting because all the processes that now they get their data from, from like servers, OnPrem, now they're gonna be getting their data from cloud and that opens a lot of possib.
But also a lot of challenges on paying, like making sure that they're on board so they can tell us exactly like, Oh, this process is getting its data from this place. Make, let's make sure that the, that when we move to cloud, we can keep doing this process and we pointed to the right place. So that's the, the beauty and the challenge of a data transformation is that you need everyone and you cannot just do it on your own and in silos because then it does not work.
So it's a lot of coordination, a lot of collaboration, and a lot of, of, of compromises, right, That you have to make as well. You have to, to start really thinking about what others need and not what you need to make this work, and then figure out something in between. So it's a lot of different teams working on it, but definitely worth.
Richie Cotton: Okay, so all this sort of collaboration between lots of teams, I know it. Often really, really hard stuff. So I'm wondering how do you manage all these teams having to communicate with each other and collaborate?
Vanessa Gonzalez: So you said some processes, you said the leadership has to be aligned, so. It starts there with the leadership really being on board, having our CEO and having our CIO and our CTO all thinking in the same way and thinking where we wanna go. That's one piece. The other piece is that when you start getting more tactical on how we get things done, We have tons of meetings between several teams.
So for example, for figuring out what data we're gonna bring in, and I'm, I'm working very closely to that one. I organize a meeting when I, where I invite architecture, I invite engineering and I invite the business, the program management office, and also our data and analytics team. So that way we understand, okay, what are the requirements of data?
From these business owners of, of these processes. And then what are the, what is the data that's already in place? So we talk to architecture, engineering, and what are, how are we gonna bring it in? So we have to talk to them as well. And then governance really helps us out on, okay, how are we gonna govern this data?
How are we gonna cure, curate? What are we gonna be looking at when we're thinking quality? And what is gonna be the right source? It's not just bringing the data and dump it there. You. Figure out if you are, if you wanna say like the name, Where do we bring name from this database, From this database, from this database.
Which one is the right name? So we have to do some mastering there. So there's a lot of collaboration between these teams. And what we do is that we, we meet regularly and we break it in pieces, right? They say, How do you eat an elephant? A bite at a time. Well, how do you do a data transformation? A few data items at a time.
you just start like putting in little, little pieces and moving those pieces and making sure that everything that you do follows that, that same purpose and you're doing it in the same way, so it's easier to get to where you wanna go.
Richie Cotton: I imagine with a program. Something must have gone wrong somewhere. So I'm wondering what have you found that has been challenging, or is there anything that you wish you'd known at the start?
Vanessa Gonzalez: So I think that's something that is very challenging and, and we have learned in the way, is that you need really good requirements at the very beginning of everything. You have to make sure. You don't skip any, any pieces, uh, when you're putting your requirements together. So for example, if you're gonna have a project where you're gonna bring data from many different other places, if you forget a couple of pieces, At the time that you meet them and they're not there, it's a lot harder to bring those pieces in.
It's a lot easier when you plan ahead and you say, Okay, these are the pieces I need. These are the transformations I need to do, and this is where gonna take it from point A to point B to point C, and this is the final place where it's gonna leave. I'm gonna curate it this way. It's a lot easier than bringing 80% of it.
And this like, The 20%. Oh, and we need this other piece and it's not as sufficient. So that is one thing that I think is challenging and it really makes sense to spend the time before you start moving data to really have those clear requirements. That's one piece. Another like challenge is that you have to keep doing what you're doing and making room for new.
So you have to be making sure that you are. Doing the, your everyday job, right? And at the same time, you have to put a lot of emphasis on the new stuff so that that means more work and mean means a lot more effort. Totally worth it, but you have to be careful of how, how you do it. So you don't not do your BAU work and business as usual, and at the same time, you're building something new.
And then at what point you move from the old to the new. You have to really test well if you don't test well. And can you imagine. You don't have the old and the new doesn't work, that would be really, really bad. So that's something that I think that we all learn the, the hard way at some point when we think we're gonna go into production on something and it doesn't work as we thought it was gonna be because we missed a couple of pieces.
So it's always good that you have that plan B of, okay, if I, before I go into production, I'm gonna test it and, and make sure that it's gonna. And then you, you keep both at for a little bit and then you, you cancel the old. So those are things that they're challenges, but they're definitely things that we have to think about.
And always think about a plan A, plan B and plan C just in case something go as planned. Because as you plan, plan for the worst and expect the best of the say or something like that, the saying goes, I'm not sure, but you better plan for everything
Richie Cotton: Okay, . Yeah. So that does seem really important, the idea of like trying to avoid introducing new bugs into. just as you tr as you move data around. So I'm curious if you have any more to say on how we go about testing things.
Vanessa Gonzalez: So, yeah, so we have a really good programs to, to test. For example, I can talk a little bit more on the machine learning side. We make sure that we test on our own environment. We have a research environment that is a pro environment because we use pro data training, but we're. In a development environment at the same time.
So we do all our testing, we check that our models are working. We make sure that the output that we're getting is what we're expecting. And once from there, then we take it to all the environment. So we go from there, we go to dev mode, then we move it to to test, and we move it, move it to model, then we move it to pro.
So in all those jobs we're. Checking and rechecking that everything works, that we're not affecting any other processes or any other pieces. Something also that we do to for testing is that our production team has a production process that you have to go through it, and as we are moving through the environments, they check their scan.
They make sure that if something breaks, how can it be fixed? And then by the time that it's in production, we're pretty comfortable that what we did is what we're expecting and that that there's not gonna be any issues. And we have always the plan B, if there's some issues, what is the way of solving those issues?
We always have that also ready to go in case something would go.
Richie Cotton: As well as having this sort of multilayer testing thing, you've got ways of like diagnosing the problems and having backup plans for like what you do when you
Vanessa Gonzalez: Yeah, so we know, okay, what happens if suddenly we lose the whole data for a day or two? Well, we have, Oh, we can use this backup, We can use this. Like there's always a plan B there of how to mitigate the issues that we may have. And depending the severity of the, of the problem or the importance, how many system will be affected?
Then we. Backup systems. If something fails, then the backup comes in. And so we make sure that we're always in a good place. So that's some something that companies do, including ours, to make sure that, that we are meeting aid, any, any issue that it may happen. Right? So that way, like if you imagine if, if companies didn't do this, then you would not be able to do anything, right?
Like suddenly your bank is down and you cannot do anything. That doesn't work for very long.
Richie Cotton: Yeah. Hard to make money when, when all these systems are down. Uh, . Okay. I'd like to talk a little bit about skills. So it seems like, cause everything. Changing quite fast. Within your team and more generally within your organization, how has that changed the skill set that you look for in your team?
Vanessa Gonzalez: On the skill set, what we're looking for is really for data scientists and people that they're willing to learn. Because things are gonna keep changing. What it was like the years ago, it was some programming language, then we changed. Then Python became like the one that we're using. But then if you are using, if you are in cloud, they need to know a little bit about how to deploy in cloud.
And then depending on, So everything changes, right? The tool stack may change again. So when I'm looking for people for my team, I'm not looking just for what they know, but. How good they are to learn how willing they are to learn, because that's the most important piece that I see for data scientists, at least for machine learning, for ai, you have to be ready for change.
We may have Salesforce right now as a crm, but who knows, maybe in two years we change to something else. So you have to be ready to think in a very open way about how can we integrate the output of our. If we change systems or if we bring a different application that we cannot, we don't even know that exists, right?
So maybe in two or three years, that completely changes. So we have to be ready for that in the skill size. I would say for my team, I'm always looking for a strong sense of statistics and math. That understanding of science of how you think. I'm gonna have a hypothesis and then I'm gonna prove it and then I'm gonna do this.
Like having a very organized mind of how you're gonna approach a problem to solve it. I think that's very important. And languages, we can learn them. New software, we can learn it, but what we, what is hard to teach is the ability to learn. And that's what I'm always looking.
Richie Cotton: Okay. Certainly that point that technology changes fast. The bits of software you're gonna be using will change every few years. That really resonates with me. But yeah, I like the idea that you always need to be willing to learn new things. So on that note, actually, when you do find that you've got a skills gap within your team, have you been training people internally or do you hire the skills like from you people outside your organization?
Vanessa Gonzalez: So we have, we have done it both ways. Like sometimes I get people with D like skills that they bring with them. Other times I also, our team is very supportive about training to learn new skills. So one of my team members, for example, he's very passionate about natural language processing. And he had, We have provided a lot of training on that side and he has learned a lot on the job as he goes.
Right. And in other cases, one of my data scientists, a statistician, Brought a lot of knowledge on that side, on the statistics. So I think that for machine learning and AI team is very important, or at least I find it very important that, that there's different backgrounds that one of the beauties of data science that you can come from being a physicist or being a statistician or a computer scientist.
Like there's, there's like a lot of different backgrounds where how you get there. And for us it's, it's amazing when that happens because that. We, they bring different skills that they can share and teach to the team as well. So something that we do and we are very purposeful about is that we have a lot of sessions about sharing so they can help each other and learn from each other.
And to be a, have a, a. Data science successful team, you need to be able to do that because nobody's gonna come in with all the skills. There's no way that's gonna happen. And even in your own team, Nobo, not all of them. I can have all the skills. So you need to have someone that has very strong skills in one area and others that have very strong skills in other, and then they share and, and teach each other and help each other.
That's something that I, I value the most. I know with data camp that they go in and every now and then one is gonna be looking into deep learning. Another one is gonna be looking into maybe PIs park another. So depending on what they wanna learn, they're gonna, they're gonna be moving in different directions.
And it depends what they're specializing on as well at the moment. What they're gonna have to.
Richie Cotton: I love that your team's using data camp for continuous learning and, uh, improving their skills. That's wonderful. So you've talked about how your team, like they need to be good at translating technical. Problems into things that business people can understand and the importance of having a, a learning mindset and the importance of understanding statistics and hypothesis testing.
Is there anything else that you think makes people in your team success?
Vanessa Gonzalez: So I think that creativity is super important because not everything goes as we want it to go, and being, having that positive attitude about finding a solution. We don't have the chance of saying, Oh no, it cannot be done. Like we are more about how do we make it work? The data is not in the perfect place.
Well, we make it work. We have to, to adapt to this, this way of doing things because. It's gonna keep our data safe. Well, we adapt and we make it work. So in my team, for me, it's very, very important. Somebody that when they see a problem, they're creative and finding a solution and not giving up, just like figuring it out how to get.
That solution to me is, is very valuable. And it happens more than you, than one thing. You go to school and they give you the perfect data set and they say, Build this beautiful model. And it always works, right? So you're like, Oh yeah, I, I tried these five different techniques and Oh, work really beautiful.
You go to the real world and it's like, Well, how do, where do I start? The data is really, really weird. Well, all those pieces being creative, it's gonna, it's gonna get you there. So, uh, to meet creativity and positive attitude, that's really what is gonna make it happen.
Richie Cotton: Absolutely. I like that. Okay, so just finishing up, is there anything that you are really excited about in the world of machine learning and AI at the.
Vanessa Gonzalez: So I, I, I have to say that I love it all , something that it gets me really excited on this world. It's really the possibilities of making change. I love models that they, that, that when you, you create them and, and you have an output, that output is used in a way that the customer doesn't even need to know.
Or, and in this case, our, our business customers, like our business side of the house, you make their life easier in many ways with they having to worry about it. It's like automatic, that AIP piece of doing prescriptive stuff and taking decision. The go that makes, makes it, to me super exciting, exciting and being able to use real, real time data and in and running models real time.
I think that's something that, that keeps me very excited every day and I'm looking forward and working on as much as I can.
Richie Cotton: Wonderful. Yeah, so I think AI and being able to do. Uh, driven decision making automatically. That sounds fantastic. And yeah, real time analytics, also. Wonderful stuff. So, do you have any final advice for other companies trying to get started with their data transformation program?
Vanessa Gonzalez: I would say that, don't think about how complicated or how big it is, but what you're gonna get from it. So I think that my biggest piece of advice is that it's not easy and it's long and time flies when you're having fun. So just enjoy the journey and make it happen. I think that that what I would say about data transformation and for machine learning and ai, I would just.
There's so much we can do and such a big difference you can do wherever you are. It really doesn't matter in what industry you are or what type of business. There's so always a way to help people and to help everybody else to, to make their life easier that we can use. So that's like if, if that's what you care about, it's a great feel to.
Richie Cotton: Making other people's lives easier. That sounds wonderful. That's great. All right. Thank you very much for Vanessa for your time. That was really, really informative. Thank you very.
Vanessa Gonzalez: Thank you so much and thanks for having me and best of luck to everybody that they're building a career in data science and machine learning and data transformation. Super fun thing to.