Fighting for Algorithmic Justice with Dr. Joy Buolamwini, Artist-in-Chief and President of The Algorithmic Justice League
Dr. Joy Buolamwini is an AI researcher, artist, and advocate. She founded the Algorithmic Justice League to create a world with more equitable and accountable technology. Her TED Featured Talk on algorithmic bias has over 1.5 million views. Her MIT thesis methodology uncovered large racial and gender bias in AI services from companies like Microsoft, IBM, and Amazon. Her research has been covered in over 40 countries, and as a renowned international speaker she has championed the need for algorithmic justice at the World Economic Forum and the United Nations. She serves on the Global Tech Panel convened by the vice president of European Commission to advise world leaders and technology executives on ways to reduce the harms of A.I.
As a creative science communicator, she has written op-eds on the impact of artificial intelligence for publications like TIME Magazine and New York Times. Her spoken word visual audit "AI, Ain't I A Woman?" which shows AI failures on the faces of iconic women like Oprah Winfrey, Michelle Obama, and Serena Williams as well as the Coded Gaze short have been part of exhibitions ranging from the Museum of Fine Arts, Boston to the Barbican Centre, UK. A Rhodes Scholar and Fulbright Fellow, Joy has been named to notable lists including Bloomberg 50, Tech Review 35 under 35, , Forbes Top 50 Women in Tech (youngest), and Forbes 30 under 30. She holds two masters degrees from Oxford University and MIT; and a bachelor's degree in Computer Science from the Georgia Institute of Technology. Fortune Magazine named her to their 2019 list of world's greatest leaders describing her as "the conscience of the A.I. Revolution."
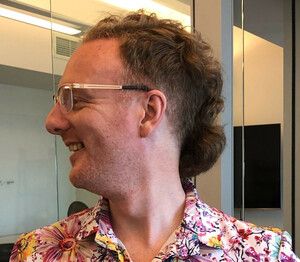
Richie helps individuals and organizations get better at using data and AI. He's been a data scientist since before it was called data science, and has written two books and created many DataCamp courses on the subject. He is a host of the DataFramed podcast, and runs DataCamp's webinar program.
Key Quotes
I decided to even focus on gender classification is I had done a Ted feature talk and I decided to take my profile image and run it through online demos from different companies with their facial analysis systems. So some didn't detect my face at all, like the white mask fail. And then others that did detect my face, they were labeling me male which I am not. And so that's when I said, huh, maybe there's something to explore here on the gender aspect of things and looking at gender and skin types. So that's how I ended up going to do this research that then became known as the Gender Shades Project.
For me, it wasn't so much that, you know, I feel square and not square, you know, that kind of thing. It was more so being my full self. And I am a poet, I'm an artist, and that has always been the case, regardless of what I was doing with computer science or within data. And so for me, I would encourage people not to feel like you have to hide parts of who you are with the work that you do. So for me, poetic expression and, you know, the art installations and things like that, they're true to who I am and ways I want to express myself.
Key Takeaways
Focus on the Entire AI Lifecycle: Beyond the end product, consider the design, development, deployment, oversight, and redress processes. Implement robust feedback mechanisms and incident management processes.
Ensure that individuals know when AI systems are being used in decisions that affect them. They should have the right to challenge AI-driven decisions and be informed about the systems influencing those decisions.
Recognize the potential for bias in AI systems, especially when datasets are not diverse. Ensure that the training population fits the intended user population.
Transcript
Richie Cotton: Okay, welcome to DataFramed. This is Richie. With the adoption of AI ramping up in every industry, everywhere in the world, there's a paramount need to ensure that AI is ethical. This is particularly the case with computer vision AI for recognizing faces. There are so many use cases for this technology. From facial detection to improve the autofocus when you take a selfie to facial recognition that allows automated border control checks, to facial identification to find criminals from security footage. While the widespread use of this technology brings many benefits, there are also a lot of ethical issues to deal with, particularly in security and policing applications. That's a polite way of saying that there's a lot that can go badly wrong. In particular, there are issues to address when the technology fails. And this is where the story begins for today's guest. Joy Buolamwini was an MIT researcher building a computer vision product When she discovered that facial detection AI didn't work on her dark skin, this kicked off a fascinating journey about bias in computer vision AI that led to a Netflix documentary, Coded Bias, work on the United States AI Bill of Rights, and the foundation of the Algorithmic Justice League.
Joy joins me today to tell her story and discuss her book, Unmasking AI. I'm hoping to learn about some of the ethical issues in computer vision and how to deal with them.
Hi, Joy. Welcome to the show.
Joy Buolamwini: Thank you so much for having me.
Richie Cotto... See more
Joy Buolamwini: Yes, so I got started looking at questions of algorithmic bias through a graduate student project I was working on. So I was a master's student at MIT. I took a class called science fabrication and the idea was read science fiction and build something fantastical as long as it could be delivered in six weeks.
So I wanted to explore the idea of shape shifting. And given that six weeks wasn't enough time to really change the laws of physics or find some innovations around that, I thought instead of shifting my physical shape, maybe I could transform my reflection in a mirror. And so that's what led to this Aspire Mirror project, which essentially would.
Act like a video filter you might see on a social media app, but instead of it adding a filter on top of a video feed, it would add a mask through the mirror. And so I got this to work. I was really excited, but it was almost like when you go to a theme park and you have to put your head in just the right place to have the effect look correct.
And so I thought it would be interesting if the actual filter could follow me in the mirror. So I got a webcam, downloaded some software for face tracking, and this is where the story takes a little bit of a turn. And so I got generic face tracking software. I thought, you know, I have a face, it's face tracking, but it wasn't detecting my face that consistently until I literally put on a white mask.
And it detected the white mask almost immediately. So I started asking questions. Was it the lighting conditions? Was it, you know, what was going on here? Was there something more? Or was it just something about my specific face? And so that's how I went down this whole journey that now has an organization, a book, a Emmy nominated film and all of these things.
But it started with an art project and asking questions.
Richie Cotton: That's a pretty amazing story. And it seems like, with the Aspire mirror, the fact that it couldn't detect darker skin faces is annoying, but not a huge problem, but it just seemed that in general. This problem with facial recognition in general can have some serious consequences.
So can you maybe talk me through, like, what some of those bigger problems are? A
Joy Buolamwini: Yeah, so it's one thing not to have my face detected for my art installation, as annoying as that is not the higher stake situation. I really was not thinking I would focus too much on issues of facial recognition technologies, whether it's gender classification or age estimation, or what we see more with mass surveillance, which is facial identification, one to many, searching.
But in 2016, around the time I was exploring these types of questions, Georgetown Law released a report called the Perpetual Lineup Report, and it showed one in two adults in the U. S. had their face in a facial recognition database, face data set. that could be searched by law enforcement without warrant using algorithms that hadn't been audited or tested for accuracy.
And so when I saw that police departments were starting to actually adopt these types of tools, that's when I knew it was more than my annoyance at an art project, but that there were people whose actual lives and lived experiences were going to be impacted in important ways. And sadly, we have had, and we continue to have, false arrests linked to facial recognition misidentification, the very thing I was warning against in 2016.
In 2020, you had Robert Williams arrested in front of his two young daughters due to a false facial recognition match in the city of Detroit. 2023, you had Portia Woodruff arrested just a few months ago while she was... eight months pregnant. She reported having contractions while she was sitting in the holding cell.
So this experience of, oh, my face is not being detected, what's going on, really opened the door to much more severe uses of the technology with dire consequences. And you can go a step further now that you have systems, let's say, autonomous weapon systems, or think about drones, cameras. guns and facial recognition technology.
So the stakes can literally be life threatening But you also see facial recognition being used in ways that can shape people's opportunities. So some hiring firms are adopting facial analysis as part of the interview. Process. And so biases that are part of that system can actually preclude you from getting a job, and you have facial recognition systems being used by schools.
Some are adopting it for a proctoring. We saw an increase of this, particularly During uh, the pandemic there was a student in the Netherlands who actually brought a case against her university because she had to get all kinds of extra lighting and etc just to be recognized by systems. And then you have other systems that will flag students as cheating.
Even though they're not, there are all kinds of reasons why a face might not be detected, or why a student might not be looking directly at the camera, and it might be due to disabilities. And so there's this issue of ableism, there's issues of racism, there are also issues of sexism as well, not just when it comes to facial recognition technologies, but the AI techniques.
And tools that power many AI systems, even going back to the school context again, you have large language models, as I'm sure many are familiar with now the LLMs being used and generating all kinds of texts. And so some educators and teachers want to try to detect if this system has been used. There's studies now showing that.
Students with English as a second language are being falsely flagged as having used an AI generated system. And to me, this is an example of a context collapse, which I talk a little bit about in Unmasking AI. I use the example of a Canadian startup, and they were doing a system that would listen to somebody's speak and based on that make a determination if that person had Alzheimer's.
They trained it on Canadians who spoke English as a first language, but then when they were running the system on Canadians who spoke French as their first language, they were being flagged as having Alzheimer's or being at risk for it, even though that wasn't necessarily the case. So the reason that their English didn't sound like the training set.
was a different kind of signal. So it's really important that we think about context and context collapse. So being trained in one system with one set of assumptions, and then that being ported over to another area, sometimes with good intentions, right? They were trying to help people, but not with the full appreciation of the differences.
Richie Cotton: lot of impacts there. So, I mean, I think even going back to the original example you said, where there's people being falsely arrested, even if it gets resolved, that's still causing some trauma and problems for the family, at least in the short term. And then, yeah, being misdiagnosed with Alzheimer's has also got to be...
Pretty traumatic as well, I'm sure. So, lots of consequences there. And I'd love to talk about those more in a moment. But before we get to that, let's flash forward to now when you've got your new book out. Unmasking AI.
So, can you just tell us a bit about, like, what the book's about and who it's for?
Joy Buolamwini: Yes, the book is for anyone who's caught in between fear and fascination about what the future of AI looks like and wants to be part of the conversation. So I say if you have a face, you have a place in the conversation about technologies that are shaping our lives. And we know that artificial intelligence systems are being adopted in many areas.
So the book is really my journey from an idealistic immigrant. Very much a tech geek, building my robots, excited to be at MIT, enamored with the possibilities of technology, and then in my journey learning that this technology I was so excited about can actually perpetuate so many societal ills. And then finding ways to combat that, whether it's working with people on the front lines, working with people at companies, developers themselves, policymakers, lawmakers.
So it's also a book of hope with all of these things we can do to actually build technologies that work well for all of us, not just the privileged few. So we can realize the promises of AI. And you get a lot of behind the scenes of what it's like to be a grad student at MIT Media Lab, or what it was like to be at Davos, talking to all of these world leaders and realizing I'd come a long way from my art project or.
Testifying in the halls of Congress. I even talk about my meeting with President Biden and a handful of other AI experts. It's really inviting you in, and along the way you meet many characters who are well known within the AI space as well. So my hope for the book is that people feel inspired.
And they feel that they can be part of creating better technologies, and they also know that they don't have to accept AI harms. We can push back. We've done it successfully in the past, and we'll continue to do it in the future. So it's an invitation to be part of the conversation and to get a little bit of a behind the scenes peek of what it takes to make AI and change AI.
Richie Cotton: It is a fascinating read. And I have to say, my favorite bit of the book was about you writing your thesis and how things gradually spiraled and you were, like, finding challenges to overcome and things like that. So I guess, could we go back to the start of when you were writing your thesis, like, and you found, okay, we've got this problem with computer vision and facial recognition.
So where did you begin with that?
Joy Buolamwini: So after I had the issue of coding in a white mask to have my face detected, it became the focus of my master's thesis work. And so I wanted to see again if it was just my face, if it was just the lighting conditions, or if there might actually be some systematic bias on the base of skin type, and also on the base of somebody's gender.
And The way I decided to even focus on gender classification is I had done a TED featured talk and I decided to take my profile image and run it through online demos from different companies with their facial analysis systems. So some didn't detect my face at all, like the white mass fail, and then others that did detect my face, they were labeling me male.
which I am not. And so that's when I said, huh, maybe there's something to explore here on the gender aspect of things and looking at gender and skin type. So that's how I ended up going to do this research that then became known as the Gender Shades Project. So that was my MIT master's thesis work. And then once I finished the master's, I published it with my good friend and long time collaborator, Dr.
Timnit Gebru. So, the Gender Shades paper that most people know is the 2008 published paper. The original work is based on my 2017 master's thesis. And if you go to GS, ajl. org. All the various variations, datasets, all, all the things you could imagine to know about the project are there for your listeners who might be curious in learning a bit more or reading the various research papers that came from that work.
Richie Cotton: Absolutely. Like, I feel that this is a very important paper, but one of my favorite or one of the bits I found most interesting about the research was the data collection side of things. It sounded like a lot of the problems with these models originally were that they've been trained on mostly white male faces, and so you went about collecting your own data set.
Can you talk about, like, what happened there? I know a I don't you had a few challenges in terms of getting a good data set.
Joy Buolamwini: The big thing for me was really not is it easy to collect face data because it can be easy to collect face data, but can I collect face data that is more diverse than what had been collected in the past? So when I was doing the research and I wanted to test how well different systems guessed the gender of a face, my first thing was looking at existing face data sets.
And when I saw the existing face data sets, to your point, many were what I like to call pale male data sets, right? So largely male, largely lighter skinned individuals. So it became clear to me why even though I was having challenges using these systems, the papers and the research that was published suggested that the systems were getting better and better.
And they were to a point, but that knowledge was limited to the people who were in the data set. And so the existing data sets were actually giving us a false sense of progress. So we had misleading measures. So because of this, after saying, okay, let's see what's out there, then I had to go create my own data set.
So it's one thing to critique other people's data sets, right, point out what's wrong. It's another thing when you actually have to figure out how to do it yourself. And one of the big things that I ran into that became a constant point of tension was consent. And so at the time that I created the pilot parliament's benchmark, it was possible for me to go on the websites of different parliament members and scrape face data.
And To try to have a more balanced gender set for this particular data set, I actually went to the UN Women's website to get a list of the top nations in the world by their representation of women in power. I looked at the U. S. More around the hundreds place, so it wasn't even close, but the countries that did do fairly well were certain African nations and also some Nordic nations as well.
So I ended up getting a data set of parliament members from Finland uh, Sweden, which I thought was interesting, as well as Iceland, and then in the African context, I had Senegal, Rwanda, and South Africa. There were countries from the Caribbean and other places that were also in the top 10, but the reason I decided to focus on the parliament members from African nations and European nations was because I wasn't just looking at gender, I was also looking at skin type.
And to look at skin type, then brought in a whole other classification system. How do you classify people's skin types? What are scales that exist that I can already use? And one that existed was the Fitzpatrick skin type classification system. And so that one was a, at first it was four classes. So, three different ways to burn if you're white.
and then the rest of the world because it is a scale about the skin's response to UV radiation, sunlight. It was later expanded to be six point scale, but the issue with that was the majority of the world would be represented in the last three categories. So it was still skewed, but nonetheless it was a starting place.
The middle of that scale was very difficult To try to categorize people in based on how they were described for what skin types would fit that, particularly when you're looking at people from the Caribbean, or I would say the majority of the world. So I wanted to try to choose people who would be on closer to the extreme ends.
of the Fitzpatrick skin type cell. So that's where you go from Iceland to Senegal, right? And those are still nations, right? So within South Africa, you still, of course, had white parliament members given apartheid and also given the colonial history. But that was a really interesting artifact of the research because sometimes One might argue that, okay, the differences we're seeing aren't just because of skin type or gender.
It might be the photo quality. There might be other artifacts that are leading to the differences we're seeing when we're running the experiments. So it was actually really helpful to have South Africa where you had a different representation of the population and see that we still had those same issues even on a data set coming from the same place.
Richie Cotton: I have to say, it is fascinating. It's one of those things with data collection where you think, Oh, it's going to be easy. I'll just, scrape or something. I'll get millions of photos immediately. And then you realize that actually there are a ton more issues that you have to deal with.
And particularly like with classifying data, it becomes hard. So, I found it very interesting just the way you went through all those different steps just to figure thing figure out how to get something an ethical data set that
Joy Buolamwini: Yes, and there's still questions about how ethical the data set I collected for pilot parliament's benchmark is. I don't think I would be able to create that data set with the laws that exist today. For example, you have GDPR, the General Data Protection Regulation that came out to protect people in the EU.
It was not in effect at the time that I was collecting the biometric data. of EU citizens, so these issues still remain when it comes to data collection.
Richie Cotton: Do you have any advice in general about what people need to worry about when they're collecting data on people?
Joy Buolamwini: I think one is thinking through what informed consent looks like. And so that was one of the challenges I had as I was collecting the pilot parliament's benchmark. I did not reach out to 1, 270 individuals to ask for their permission. We used the fact that they were public figures as a bit of a proxy to say, okay, they are not necessarily private individuals in the same way.
But even that is a, a bit of a shaky justification. And I remember talking to other older, practitioners about some of these issues and they were looking at me like, get on with it. This is, this is how we do computer vision. We scrape the data sets, it's data that's available and the current thinking is if it's out there, then you can use it.
But we're seeing even now with all of the lawsuits that are happening, that data isn't just for the taking. Right. And so we're seeing this with stability, AI and the. Getty images, a lawsuit. We're also seeing it with authors, right? Who are going up against companies that have built large language models that they suspect have been trained on their copyrighted books.
And so to create data sets and then use those data sets to make products. that you're profiting from without permission or without compensation is likely going to continue to lead to more litigation. So I would advise anybody who's building AI systems and you want to build an ethical AI pipeline to really look at the data provenance.
Of whatever systems you are thinking of incorporating and this is really important too because there's so many Models out there now where you might take it and fine tune it without necessarily thinking about the source of the data for those models And in some cases, even if you wanted the source for the data for those models, it wasn't well documented.
So that's another thing that people can do if they are thinking through, how can we be better? Document the data sources. Document the classification systems you're using and why you're using those classification systems, as well as the limitations. So I really think ideas like, Data sheets for data sets and model cards for models are so important because it forces us to look at the systems we're creating both our aspirations for it, but also the limitations and that can help with that notion of context collapse.
So if we know, okay, this is who it was trained on. Maybe it's a AI model meant for health care, but it was trained mainly on data sets of Caucasian men. We can say this can be helpful in this use case. or on this population, but we have to be very careful so we don't end up sending what I call parachutes with holes.
Good intentions, right? But did we check?
Richie Cotton: That does seem incredibly important, just having that level of documentation so people can understand is this model suitable for use or not? One other aspect of your research was around auditing existing computer vision products. Can you just tell me a bit more about how you went about doing that?
Joy Buolamwini: Yes, so after I realized that I wanted to focus on gender classification systems, then the question was, well, which ones do I test? For my research, when I did it for my master's, I tested an open source model as well as commercial models from a number of tech companies. So for the first study, we had IBM, we had Microsoft and we had face plus plus based in China.
And then for the second study, we included clarify and also Amazon. And part of choosing those targets, the initial targets for the first study. was which companies had demos available that were easily accessible. And so for me, that was a signal of confidence in your product. For them, it could have also been a way of collecting more data to train the product, right?
So that was one of the ways of selecting. And I also thought it would be important to select companies that had name recognition in the U. S. Because if I showed that, oh, these issues are happening with companies with immense resources, much more than I had as a graduate student, right? That people would pay attention.
And I also wanted to choose a company based in China, given the access to data sets that some Chinese companies are provided with access from the Chinese government. And also, that the research had shown, especially for facial recognition systems systems developed in Asia work better on Asian faces, systems developed in the Western part of the world work better on Western faces, etc.
So I also wanted to see if those dynamics would be at play. So that's how I selected the initial targets. And then for round Deborah Raji who you'll see in the film Coded Bias, and it's also in the book, she reached out to me and she wanted to know if there was some way she could do some research with me because she was also interested in issues of algorithmic bias, and she happened to have interned at Clarify, so that's how that became one of the companies we explored, and Amazon, at that time, Was selling facial recognition to law enforcement and there had been a number of letters signed by many people Asking the company to stop doing this and so that became a reason to then include amazon.
So amazon unlike IBM and Microsoft at the time. You couldn't just go on the website and test it out. You actually had to sign up, etc. But we, we did all the things. So we paid to find out what we learned, right? Which is when we tested these systems with the now more diverse pilot parliaments data set that they all showed gender bias and they all showed skin type bias, which then maps on to racial bias as well.
Richie Cotton: Absolutely. It's. Shocking that this was a problem in all of the products that you tried, that there was this discrepancy in performance between different skin types and between between genders.
Joy Buolamwini: I will say I was I was most surprised with Amazon's results because we tested a year after. So there had already been a new cycle around the results from IBM and Microsoft both had released new models, et cetera. So for me, it almost seemed like failing a test where the answers had already been.
Shared in a manner. So that was actually really surprising. I thought that the second test we did, they would have a bit of a head start and so that they were where their competitors were in a little bit worse. the year prior when we tested it was really surprising to me. The other thing that was really important about that research was that we didn't just look at skin type and we didn't just look at gender, we looked at the intersection of skin type and gender.
So that's when we found that all of these systems work the worst on Black women, right? Women with dark, so women like me, you know, and I thought that was interesting because when we looked at the various models, sometimes the performance on, let's say, darker skin males. would be better than the performance on lighter skinned females or vice versa.
And the best performance in our tests was always on lighter skinned males. But I point out those differences to say that you can't use the performance of one specific model to then extrapolate about the performance of all other models. You actually have to do the specific test and the specific intersectional.
analysis for each model that you're making.
Richie Cotton: Yeah, that's a great point about testing is you think, Oh, well, I've tested one thing and maybe it applies to something else, but often it doesn't. So you got to be really, really careful about what what exactly the results of your tests are. I have to say, I did try a couple of these sort of face detection tools in this last week just to see whether they worked on me.
And for Everyone in the audience who is audio only, I'm close to the theoretical limit of how white a person can be. And so it did correctly guess my skin color, they guessed my gender. The ones that showed age, they all added about a decade on to my age, but it was very rude.
But that's a, that's a, less of a sort of a,
Joy Buolamwini: Well, I will, I will, well, I will say I, in addition to these tests that we talked about, the actual research tests, we also did other fun exploratory tests. So we did one with the women of Wakanda from the Black Panther film series. And because you mentioned age, we did Angela Bassett, who played Queen Ramanda.
And it went the opposite, so I think the IBM one said she was around 25, that age bucket, so it gave her decades less.
Richie Cotton: Okay. That's more flattering.
Joy Buolamwini: I was like, you know what, maybe not all algorithmic bias is that bad, but it depends on the context.
Richie Cotton: Nice. I'd like to talk a bit about what you describe your research as being socio technical research, which goes beyond just the sort of technological solutions. Can you just talk a bit about what that means?
Joy Buolamwini: Absolutely. I think a response I've often seen to my research when people see, okay, there's skewed data sets and there are these disparities between groups, then the immediate solution is let's build more inclusive data sets. And if you stop there, that's very much thinking about it from a technical perspective.
Perspective. A social technical perspective would say, not only are we thinking about accuracy, but we're thinking about how these systems are being used. Because let's say we did have highly accurate facial recognition, which we don't have. Flawless facial recognition does not exist. Hypothetically, if we had that, we still have tools for state surveillance and state control.
Accurate systems can be abused. So when we're talking about technologies like various AI systems that are being deployed, it's not just a question of performance metrics. Those are important, right? If the system doesn't work in the first place, you probably shouldn't use it. But also we have to ask, are these the right systems to be building and how are they being used?
So When I look at the context of face surveillance, I don't want the face to be the final frontier of privacy, where you gotta go out covered everywhere, you're going. And I think that the socio technical lens requires us to ask more questions about society, more questions about power, who has it, who doesn't, who benefits from these systems, who suffered the burdens.
And I realized that my computer science training didn't really equip me. for those types of explorations. Sure, I could go scrape some websites and build a data set and run the scripts to test out various APIs and, do what I needed to do in R, but when it came to asking more of the societal level questions, that's when I found that the work of social scientists.
The work of anti discrimination law and so many other spaces were necessary to do this work properly.
Richie Cotton: It's a very different skill set for sure. And was there anything that surprised you as you moved from like the sort of pure engineering technology background to doing the social sciences work? Like, what did you find weirdest?
Joy Buolamwini: Yes, I think what was really important for me to understand was to make robust technical systems, which you might be calling pure engineering, actually requires a deep understanding of the social aspect of things. talked about that part of the book where I'm exploring different classification systems and I'm learning about, you know, various ethnic enumeration schemes from around the world and I'm looking at the Australian Bureau of Statistics and all of the various ethnicities they outline that I'm learning about visible.
Minorities in Canada. So by the time I'd gone through all of that and I'd seen how often the U. S. census had changed categories, there was no doubt in my mind how constructed these categories are and how much they reflect the historical, social, and cultural context of a time and a place. And I think it's really important to keep that in mind.
We're building AI systems that are involving humans. Language itself is ibi with so much of our biases, right? The ways in which we describe certain things. Policeman, What are we inferring with the use of that terminology, mankind versus humankind. So there are so many things that are embedded in the ways that we speak and what is viewed as valuable that.
we might think is normal, but was actually constructed. And so being aware of that then allows us to actually build better technical systems because technical systems are never divorced from us.
Richie Cotton: Absolutely. I'm sure it is very easy to get into the idea of thinking that, oh, well, there's a standard here, so it must be like some kind of golden standard. And actually there is all that sort of historical or cultural context around it.
Joy Buolamwini: Right, and the question of who gets to determine what the standard is. So if I say, okay, in order to win this game, you need to have curly hair like mine, right? That's already going to exclude you, you know, right? So who gets to set the norm, who gets to set the standard, and who is punished when you try to challenge it.
But, I think for me, the biggest thing I learned was to question the status quo. And I couldn't just accept that because these papers from highly respected institutions and highly respected researchers said, this is so. That was necessarily the case. And I had to believe my own lived experience, even if the data didn't yet exist or hadn't been documented or peer reviewed, published for what I was expecting.
And then taking the time to go and investigate and figure out what was going on. So I think a big part is staying curious and staying humble and not accepting the status quo or the gold standard as the end all be all.
Richie Cotton: Just while we're on the subject of socio technical research, I think one thing that happened throughout your book is like at the start, you're like, okay, well, bad computer vision that doesn't work is a problem. And then by the end, you're like, well, actually, there are problems with computer vision that's too good, particularly when you have like face detection that works too well.
So can you talk about that sort of double edged sword of when the AI is like bad or too good?
Joy Buolamwini: Yes, so this is why that socio technical analysis is really important. So we started earlier with the conversation about Robert Williams being falsely arrested and Portia Woodruff being falsely arrested eight months pregnant, right? So that's an example of misidentification. And so you can say, okay, technical problem, technical fix.
Let's make these systems more accurate. Then you might hear of a stadium using facial recognition to keep, this is a true story, to keep certain people out of the stadium, And so you can have somebody who's targeting you using facial recognition systems. Clare VUI has great billions of face photos, and one of the examples they had was a use case where somebody's reflection in a mirror.
was captured by somebody else, I think, taking a selfie in a gym. And that's how they were picked up. So now it's not even images you're a part of that you consented to being a part of, but showing up in some other space and then being targeted. And then I think you have examples where some companies or some researchers will claim that they can infer your sexual orientation from your face.
Data. They, some will say they can infer if you're a poker ace or you're terrorist just from your face data. So that kind of information isn't even something you can reliably detect from a face. But if people say they have these capabilities and then it's used, right? You still have many countries in the world that will put people to death.
for engaging in same sex relations and so forth. So there are systems that are problematic when they don't work. There are systems that are problematic when they do work. And there are systems that are fabled to work on things that cannot even be done by computers reliably, but are still nonetheless dangerous because they can be used to target and persecute people.
Richie Cotton: It seems there are some really tricky sort of ethical issues here as well as like the pure technical issues. And yeah, with life changing consequences for the people involved. So, I'd like to talk a bit about like possible solutions and maybe if we start with like For people who aren't particularly technical what do you think everyone needs to know about using AI systems?
Joy Buolamwini: I think it's really important for people to have a choice. And one thing we can demand is that we know when AI systems are being used. So, for example, when I talk about the coded gaze, like the male gaze or the white gaze, which is about who has the power to shape technology and who puts their preferences their priorities and also their prejudices.
So, for example, if AI is used in a hiring, Algorithm or A. I. Is used to determine a loan or mortgage decision or A. I. Is used as part of a teacher evaluation. People should actually have a right to do process to know what systems were made and to be able to challenge it. You can't challenge the power. You don't see.
You can't challenge the decision that wasn't made known to you. So I think that first thing is demanding that we know where a I. System being used as just a baseline. So you have somewhere to start. The other thing is actually having affirmative consent so that you get to decide if you engage in this and there are meaningful alternatives, if that is not what you want to engage with.
I do think the blueprint for an AI bill of rights that was released last. November is actually a really helpful starting point to say people should be protected from algorithmic discrimination. Your age, gender, sexual orientation abilities should not be a reason you get X coded or otherwise harmed.
from AI systems. They need to be safe and effective. They need to actually do what they claim to do. And this is an assumption that cannot be made just because a company claims this AI system does XYZ. Remember, contacts collapse. Maybe it looked good on their internal tests, but in the real world, you might have some other types of problems.
And another thing that we encourage with the Algorithmic Justice League is to share your story, share your experience. experiences. So we actually have an AI harms reporting platform where people can share what's going on, and we'll do different campaigns. So we did one with the use of facial recognition at airports.
So if you go to fly. ajl. org, you can let us know, how did it go? Did you even have the option to opt out? Did you ask? How did they respond? Did the tech work on you? Do you have concerns about this? So I think it's important we continue to document what's going on. Thank you. We continue to speak up, and I think it's going to take continued resistance to see the changes that are necessary.
Otherwise, we get this narrative that people are, accept this, people want this. People don't want harmful technology. Yes, they will want the benefits that are marketed to them, but you have to have the full picture and the full story.
Richie Cotton: Okay. And so for people who are asked to use AI at work, do you think there are any questions people should ask to say, is this good or not? Should I be doing this? Like, what, what should people be asking their managers?
Joy Buolamwini: I think they should be asking their managers if the systems that are being adopted have been audited, have they been tested to show that they're fit for purpose, and have, they've been tested to show that they're not discriminatory. So I think in general, this process of meaningful transparency and continuous oversight would mean that before an AI system is adopted, There's a opportunity for scrutiny and debate.
If it is adopted, there's an ongoing process of monitoring it to see if it's actually delivering on the benefits and to see what harms or burdens are also being produced. So that things can be adapted and changed in real time. So I do think having that base level understanding, what are we using? Why did we adopt it?
What systems came into, what systems came to bear through the procurement process? And what's in place if something goes wrong? Those are very basic things to have.
Richie Cotton: Absolutely, like understanding what are you, what are we doing here is in in general is, is a good idea. And for people who are building AI products what do you think are the most common ways in which AI can go wrong and and cause these sort of biased outcomes? Like what, what do the builders have to look out for?
Joy Buolamwini: Yes, one of the things I talk about in the book is this concept of power shadows. When I was doing my research, I was thinking, how did we end up with all of these skewed data sets? We have very smart people working on this, I am told. You know, So what is going on? And oftentimes what's happening is convenient sampling.
So in the case of face data sets, people would go for the images of public officials and oftentimes people in political power there. We see the shadow of the patriarchy who tends to hold political office and political power. So if that's what you're using as your subset, it's not so surprising if you have an overrepresentation.
of men. Similarly, when we saw that there were more lighter skinned individuals, there were several factors in place. One, there are automated face detecting systems, or face detectors that go through the many images, so that you as an individual aren't sorting through all the images online. Instead, you use a face detector to say, oh, this image has a face.
If these face detectors don't get darker skin faces, you've already limited that subset. But then you also have to ask from a cultural and social perspective, who's given airtime, who's given screen time, right? Who's more likely to have their images available online? So all of these are power shadows that come from convenient sampling.
So you have to really ask, does the training population fit the population we are intending to use this on? And do people have agency and a choice and a voice about how it's going to be used?
Richie Cotton: That's excellent. I do like that idea of like making sure that you're not doing convenient sampling and you're actually thinking probably about like the sampling strategy for your data. I think the other aspect for people who are building these systems is about being able to communicate like about accuracy and limitations to users.
And do you have any advice on how to do that better?
Joy Buolamwini: I definitely think the data sheets for data sets are helpful to say here is the data that went into the system. Here are the limitations. I think also saying Was this data copyrighted data, right? How did you acquire the data? I would like to see something like fair trade data or ethical AI pipelines so that you, as somebody using an AI system, I'd prefer to use the system that wasn't built on stolen data.
I'd prefer to use the system where they paid people more than 2 an hour to do the toxic content moderation. And so I do think that there's certainly is an interest for people to use tools where they feel good about how those tools were made in the first place. So that's an opportunity. That's
Richie Cotton: Absolutely. And I think maybe for people who are managing AI products is there any advice you can give there on how you can build in these processes or generally improve the quality of products there? What would you say to managers?
Joy Buolamwini: such a great question. I think it's so important to focus on process of the entire AI life cycle and not just products. And so when you see research like gender shades, part of it focuses right on the black box product we have at the end, which gives us a. very limited view, though it was a powerful view on what's going on on the inside as a company, right?
You can think of the design process, the development process, the deployment process, the oversight process, and an area many people don't even include as part of the AI life cycle is redress. What happens if something goes wrong? Do you have an incident management process, A way where people can let you know what's not going well, as well as what is going well.
So you want to have robust feedback so you can have that ongoing monitoring as you have these systems. You also want to have something in place if people are harmed. If you're saying you want to build responsible ethical AI, that means you have to take accountability if something goes wrong.
Richie Cotton: Absolutely. I guess that accountability part is, is key that you need someone to be there going, okay, this is me in charge of making sure that it's done. Right. So I'd like to talk a bit about your work with the Algorithmic Justice League. Can you just tell me what the league is and what its mission is about?
Joy Buolamwini: Yes, the league is a merry group of activists, academics, artists, authors, people who want a world where technology works well for all of us, not just the privileged few. A lot of our work is raising awareness about the potential harms and existing harms, as well as the emerging threats of AI that are happening to who we call the X coded, people being harmed, condemned, convicted, otherwise negatively impacted by AI systems, and we do it in a way to be as accessible as possible.
You don't need a PhD from MIT to know that if a machine discriminates against you, you've been wrong. So that's a big part of what we do. We also work on collecting evidence of AI harms. That way we can hold companies accountable and it can also inform. Different ways of building products and systems and different policies that can be put in place.
Not just from an industry perspective, but also from a governmental perspective so that there's good governance of AI systems in the first place. And then we advocate for specific changes. We will say we should ban face surveillance because we know this technology is discriminatory and we don't want to have live facial recognition in public space.
Bases. And so if you become part of the algorithmic Justice League, you get to support all of that work. We support you and also the work that you're doing to try to create a better and more beneficial technologies.
Richie Cotton: And how could people get involved in this?
Joy Buolamwini: AJL. org. You go to our website and you can sign up to be part of the Algorithmic Justice League. I highly recommend checking out the film Coded Bias on Netflix, and also it's just one chapter in the book, so if you want more of the whole story, I recommend reading Unmasking AI as well.
Richie Cotton: That's wonderful. And since you mentioned coded bias, like, as well as you scientific work you've done this Netflix documentary, you've done art installations, you do poetry. I'm curious as to where do you get all these inspirations for art?
Joy Buolamwini: Well, I'm the daughter of an artist and a scientist, so my being a poet of code is really that companionship I saw with my mom and my dad seeing art and science walking hand in hand, and so that's also reflected in the work that I do, so that's why I am the poet of code, and also POC, we need more people of color in tech.
Richie Cotton: Absolutely. And I think this is maybe a form of bias that people think data people are a bit square, not very artistic. So I do like that you've been involved in the art side of things as well. Maybe going straight for Netflix documentaries a lot for a big jump for a lot of people.
But do you have any advice on how people can working in data can get started like with some artistic endeavors?
Joy Buolamwini: For me, it wasn't so much that, I feel square or not square, that kind of thing. It was more so being my full self. And I am a poet, I'm an artist, and that has always been the case regardless of what I was doing with computer science or within data. And so for Me, I would encourage people not to feel like you have to hide parts of who you are with the work that you do.
So for me, poetic expression and, the art installations and things like that, they are true to who I am and ways I want to express myself. So I was able to incorporate that into my work.
Richie Cotton: Wonderful. And I have to say, you've been crazily prolific over the last few years, with the research papers, the documentary, and all these sorts of things. Do you have any advice for the audience on work life balance? How do you cram it all in?
Joy Buolamwini: I know, I think the book is a warning to not cram too much in.
Richie Cotton: Uh, yeah, writing books is, uh, Ridiculously time consuming. So I guess yeah, well done on getting that finished.
Joy Buolamwini: I don't think I'm the best person. My area of expertise is algorithmic discrimination and bias and facial recognition technologies. Work life balance is not my area of expertise, but I did learn the hard way that I had to actually put my own health first. And that was actually probably the most important learning that I had because if you have nothing left to pour out, what's the point?
And so I do think making time to take care of what's important to you can be hard, particularly when you're working on issues that, with the AI conversation are so urgent so impactful. It can be tempting to feel like you have to give it your everything, but you actually have to save some of yourself for you.
Richie Cotton: Absolutely. Saving some of yourself for you. That's, that's great advice. Just wrap up. Do you have any final advice for people who are interested in facial recognition or in AI ethics?
Joy Buolamwini: Join the Algorithmic Justice League. That would be my call out.
Richie Cotton: All right. Everyone's got a bit of homework to do then. Okay. Excellent. Uh, thank you for coming on the show, Joy. That was brilliant.
Joy Buolamwini: Thank you so much for having me.
blog
What is Llama 3? The Experts' View on The Next Generation of Open Source LLMs
blog
Attention Mechanism in LLMs: An Intuitive Explanation
Yesha Shastri
8 min
blog
Top 13 ChatGPT Wrappers to Maximize Functionality and Efficiency
Bex Tuychiev
5 min
podcast
How Walmart Leverages Data & AI with Swati Kirti, Sr Director of Data Science at Walmart
podcast
Creating an AI-First Culture with Sanjay Srivastava, Chief Digital Strategist at Genpact
tutorial
How to Improve RAG Performance: 5 Key Techniques with Examples
Eugenia Anello