How AI is Changing Cybersecurity with Brian Murphy, CEO of ReliaQuest
Brian Murphy is a founder, CEO, entrepreneur and investor. He founded and leads ReliaQuest, the force multiplier of security operations and one of the largest and fastest-growing companies in the global cybersecurity market. ReliaQuest increases visibility, reduces complexity, and manages risk with its cloud-native security operations platform, GreyMatter. Murphy grew ReliaQuest from a boot-strapped startup to a high-growth unicorn with a valuation of over $1 billion, more than 1,000 team members, and more than $350 million in growth equity with firms such as FTV Capital and KKR Growth.
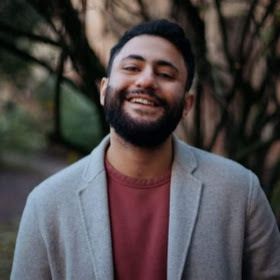
Adel is a Data Science educator, speaker, and Evangelist at DataCamp where he has released various courses and live training on data analysis, machine learning, and data engineering. He is passionate about spreading data skills and data literacy throughout organizations and the intersection of technology and society. He has an MSc in Data Science and Business Analytics. In his free time, you can find him hanging out with his cat Louis.
Key Quotes
Generative AI is definitely lowering the bar, lowering the skill set that you need to be bad. You can already today go out on the dark web and buy username, passwords, buy attacks, buy ransomware scripts. If you wanna be a bad actor in cybersecurity, these tools can be used to get you into that industry and learn.
I don't focus on the fear, uncertainty, and doubt of cybersecurity. I think there's a lot of opportunity in cybersecurity. I think bad actors using generative AI is no different than them using other tools for bad things. They've been doing it. There's always been this kind of cat and mouse game since the beginning of the digital world, right? And so it's why we exist in the first place, to stand on that wall and help customers make security possible. I think it's never just one thing that someone does that can hurt you. It's always a combination of things, which is what we're good at. So I look at this opportunity to leverage AI to make us more multiple as an industry, to help us see around corners, to help us aggregate data at a high speed and at levels that we weren't able to previously that gives us a leg up.
And I feel great about the capability. It's a useful tool to help make security possible. It helps us increase visibility, get access to data in different data types and different table fields and different table and put like next to like so we can make accurate decisions with accurate security information. So I'm more bullish on it than I am fearful of it.
Key Takeaways
While generative AI can significantly enhance cybersecurity defenses by automating mundane tasks and providing insights, it also lowers the barrier for cybercriminals, making threats like phishing attacks more sophisticated.
AI is being used to remove repetitive tasks from the workload of security professionals, allowing them to focus on more strategic, creative, and impactful aspects of cybersecurity.
Utilize AI tools to identify and address potential threats before they become issues, transforming security teams from a defensive to an offensive posture.
Links From The Show
Transcript
Adel Nehme: Hello everyone. Welcome to DataFramed. I'm Adel, Data Evangelist and Educator at Data Camp, and if you're new here, DataFramed is a weekly podcast in which we explore how individuals and organizations can succeed with data and AI. One of the things that became apparent over the last two years with the rise of generative AI is that the ability to create fake yet convincing text and images. This will put tons of pressure on cybersecurity teams. We've already seen numerous high profile attempts, whether successful or failed, at leveraging AI to do sophisticated phishing and social engineering. But that said, generative AI also has tons of potential in accelerating cybersecurity efforts.
Enter Brian Murphy. Brian Murphy is the founder and CEO of ReliaQuest, one of the largest and fastest growing companies in the global cybersecurity market today. Murphy led ReliaQuest from a bootstrap startup to a high growth unicorn with a valuation of over 1 billion to over 1000 team members, and they serve companies across the world.
In our conversation today, we delve into how generative AI can pose extra cybersecurity risks for organizations, how to effectively combat against these risks, how cybersecurity efforts can be accelerated with generative AI, And a lot more. If you enjoyed this episode, make sure to let us know in the comments, on social, or more.
And now, on today's episode.
Brian Murphy, it's great to have you on the show.
Brian Murphy: Great to be on the show. Thanks f... See more
Adel Nehme: So you are the CEO of ReliaQuest, one of the fastest growing companies in the cybersecurity space today. I'm really excited to talk to you about the opportunities and threats generative AI poses for cybersecurity. So maybe to first set the stage, as you were seeing the capabilities of generative AI tools and technology emerge over the past few years, when was the first moment you realized, generative AI is going to be a game changer for cybersecurity and what was going through your mind when you first started playing with the technology, whether chatGPT or other tools like that.
Brian Murphy: I think, you know, a lot of things have been building to this point, I think a lot of things have been building from just automation, right? Using code to automate and removing. We've always looked for ways to remove mundane tasks out of security operations. Let's let our teams are talented security teams and our customers work on the most creative project.
So it started with automation and machine learning and kind of the early days of AI. And the interesting thing about, ChatGPT a lot of the LLMs and some of the more advanced AI is you're able to connect what would normally take a human to rewrite or to put into a standard language. And now you can speed up that time for a security professional to do the most accretive thing. And so for us, it's been fun to watch this progression. And you just started to see glimpses, but I'll say the most exciting thing is the willingness of large enterprises to leverage a I where I think five years ago, there was just a lot of fear and uncertainty around it. And some of the more public facing, more consumer facing products that have led the industry to be more open to it. I think the willingness has been the most exciting part.
Adel Nehme: Yeah, and you mentioned that I think generative AI definitely put AI on the map for numerous organizations today, especially enterprises who were hesitant on doing that. And you mention as well here, the workflows of security teams, right? I want to unpack that in a bit more detail just to give listeners, a bit of a lay of the land of what it means to be a cybersecurity professional.
I think many listeners today are more familiar with AI or generative AI. But today's episode, we're going to go into the cybersecurity angle of generative AI in a lot of ways. So maybe give us a primer on the challenges cybersecurity teams face in their day to day environment and the type of threats organization face from a cybersecurity standpoint.
Brian Murphy: It's a great question, and it's something definitely great to drill down on to demystify cyber security a little bit. I think we can all appreciate that data is everywhere. It's on the phone that you carry around the applications you log into your desktops, your servers, cloud environments, multi cloud environments.
And so especially for large enterprises and small startups. Data sits everywhere and there is important, whether it's, personal identification information or intellectual property for companies, health care information, all of these things that we want to protect, things that we wouldn't want out there.
No different than, you wouldn't want your physical assets out in the opens while you lock your car doors. And so we want to lock all those data doors. But when data is everywhere, that becomes very challenging. And so when you're trying to secure that and trying to be that security guard to walk around the perimeter of everywhere that the data sits and make sure it's okay, very hard to do that without automation.
And so the reason AI and automation is so important is it allows us to remove the things that take way too much time and security, all the noise, all the data that's either normal or the data that has, is not accretive to security and remove it. So we can focus on. The most relevant data, right? So we call it removing the things that take a lot of time and the things that that don't require a trained and skilled security professional to do and get them out of the way. High time, low brain activity, mundane tasks, get them out of the way so our security teams can go respond to actual things and make sure that nobody's breaking into those data virtual buildings, so to speak.
Adel Nehme: Okay, that's really great. And maybe kind of expanding here on the type of threats organizations face, maybe walk us through the different the lay of the land of the different types of threats organizations face when their data is at risk. How is that data at risk in a bit more detail? Maybe.
Brian Murphy: We're the biggest things is still for profit, right? Cybercrime is a for profit industry. There's a lot of money in it. If I can get you to click on an email, I can do a ransomware attack. It's a relatively low tech attack and get somebody to click on a link and If I can just do that enough times and I find somebody that gives me access to information that I can turn and sell, username, passwords, unique identification information, healthcare IDs, anything that that would be valuable that I could turn around and sell for profit, or whatever.
I click on it. It's ransomware. I'm encrypting a part of your business, keeping you from running and operating and asking for you to pay a reference and I'll give you the keys to unlock the code. So there's the cyber crime. There's nation state activity, right? I want to know what's happening in another country.
You know, The United States and its allies get attacked a lot. I want to know what's happening. What are they building? How are they thinking? I want to try and deal intellectual property that maybe helps us advance our systems a little bit faster. And then thirdly, and it's not as large as the, as the crime and as large as the nation state activity, but hacktivism, right?
I don't agree with something that's happening out there in the world and I'm going to pull my capability with others and we're going to take that thing down. And there's been some, just large, well known attacks over time. So. Those are generally the three large buckets and I'm generalizing that.
But what they're looking for is just information to either discredit information to hurt your brand information they can sell or a way to hold you hostage or something they can turn and use to make them better. And that's, that's really the gist. Generative
Adel Nehme: That's a really great lay of the land. And, we're going to talk about the ways generative AI can definitely accelerate the ability of cybersecurity teams to respond to these threats. But I'd be remiss while we're talking about threats not to talk about the ways generative AI as well can accelerate these threats, right?
I think early in the release of Chachapati, we've seen a lot of examples of, the potential misuse of generative AI to accelerate something like phishing emails, for example, give us a walkthrough of where you think generative AI can accelerate the threats organizations face here from a cybersecurity standpoint.
Brian Murphy: AI is definitely lowering the bar, lowering the skill set that you need to be bad. You can already today go out on the dark web and buy username, passwords, buy attacks, buy ransomware scripts. what generative AI allows you to do is then turn around and use that to create some of the ransomware emails so that they're more accurate.
We've all gotten those emails that doesn't look right. There's a few things misspelled. The words aren't used correctly. Well, using this. Chat GTP capability where it's just closer, right? And it just looks, it's really hard to tell it's getting easier and you send those out tens of thousands of the time and you're going to get somebody to click on it.
And so we are already seeing evidence. IBM recently did a study where they used AI to generate phishing emails and the AI generated phishing emails were much more effective. Than ones that, they had seen used in the past. And so it's just an example of one of the many threats where It's not as difficult to get in. If you want to be a bad actor in cybersecurity, these tools can be used to, to get you into that industry and learn.
Adel Nehme: Okay, that's really, really great way of putting it. One additional risk that I've seen is that, you know, with the risk of auto generated code and a lot of times, that could leave vulnerabilities a normal human would be able to spot or think about or prevent in their code generation process.
Do you want to maybe comment about that particular risk of using generative AI in the enterprise about, code generation and how it could leave vulnerabilities at certain points in time in your code base as an organization?
Brian Murphy: Yeah, I think anytime you're depending on, some third party, whether it's an outsource service or or it's an AI model to create something that's critical to your organization, you can't just fully rely that the AI models, right? You still have to go through your testing protocols, your security protocols, right?
We can't just outsource that because it's not. Perfect. We know these things are are trained and they're learning and anything that's learning can be fallible. And so I think that there are opportunities where maybe it misses a line of code or it misses important logging capability in the code, something that the security team would need access to.
All of the things that we want to make sure are embedded by our work. human dev teams, we want to test to make sure that it's being done in our AI models. And I think it's just about using the same protocols and not having blind faith that this just magic wand is going to create all the code that I need.
I think it's going to help you. It's going to help you go faster, but you're going to need to test and validate.
Adel Nehme: Yeah, I couldn't agree more. We're talking about the threats here. I think it's important to switch gears, and this segues really well to discuss the generative AI opportunity in cybersecurity. In many ways, cybersecurity teams must fight fire with fire here to defend against these generative AI powered threats.
So maybe to set the stage, you mentioned this earlier that, you know, in the early days, of traditional AI and machine learning. is already being used to tackle cyber security challenges. So, maybe before we talk about how generative AI specifically accelerates cyber security efforts, walk us through how traditional machine learning is used in cyber security today.
Brian Murphy: Yeah, I mean, traditional machine learning has been used for years. I mean, it's basically a statistical analysis. It tries to make predictions based on data attributes or trends, right? So I'm seeing a trend, go look here, or this trend is more like a trend that I've already identified as something I don't need to look, right?
And so these traditional ML models, they've been around for some time in cybersecurity to detect threats and or anomalous behavior, right? And that's, really using that type of ability has been something we've been working with for a long time.
Adel Nehme: That's really great. And when you mention here, for example, generative AI being able to automate a lot of the mundane tasks that, cyber security teams have, Maybe give us an example of what type of tasks here are going to be automated or augmented and how can generative AI help cyber security teams, find the needle in the proverbially speaking here.
Brian Murphy: good example is earlier this year, we launched our phishing analyzer tool. And what it does is it analyzes the text and email you've probably gotten, training before when you get an email that you think is phishing, forward it to phishing at whatever you need to forward it to, and somebody in security is going to look at that.
Well, that takes a ton of time, and it's not very interesting work for someone in security just to be checking an email box. So we can use these models and our phishing analyzer essentially just analyzes the text of the email to determine is it a phishing attempt? Is it not a phishing attempt? we can auto isolate.
We got, there's so many things that we can do based on the behaviors that we've studied for the past decade. And that's why having. Data is so important in AI as having, the data to train the behavior of the model, right? And so fishing analyzer is an example. Another example where we're using ML models is our, customers get thousands of alerts per day from a security perspective.
It gets really noisy. And so, The challenge in security is figuring out what's noise and then what needs to be investigated further. Like what's noise? What can I ignore? And then where do I really need to focus my time? Because time is limited. so as alerts fire, our ML model is able to compare that alert to past alerts.
To see if there's similar trends, to see if there's maybe something that we've learned that we know every time we don't need to worry about. Can we trace it back to the same issue? Is it a duplicate thing that's happened? So if you just weed out duplicates, weed out noise that allows your security team to focus on things where there might be something right.
And so they can free up time and visibility for the things that really matter.
Adel Nehme: And, you mentioned here the security team working, hand in hand in a lot of ways with these AI tools and generative AI tools and their daily workflows. And I think. We often talk about the difference between automation and augmentation when leveraging generative AI tooling at work.
And I think cybersecurity teams are a great example of how generative AI can augment the current workflows of a particular team. Maybe how do you view the skills of cybersecurity teams evolving as generative AI tools become more and more embedded in their workflows? What do you think are the primary skills they need to learn?
And where do you think what needs to change in the, in the make skills makeup of a cyber security team to become? effective in using AI.
Brian Murphy: I think the dependency, if you look at, prior to automation and really we're still in the middle of this transition now of. A security professional was really required to learn how to do use specific tools. It was really, you would talk to people in security and they would talk about their expertise around a certain type of technology, not even a category, but like a specific tool.
And that's not really security. We want people thinking about The data thinking about where that data came from thing about the meaning of that day to the organization why it's important in helping the organization make. Accurate business decisions with accurate security information not managing a tool not running a technology right we want it we us interpreting the security information to help make business decisions with it and so i think what will happen is it will actually help.
Evolve the careers of our surface cyber professionals faster. If you talk to most people in cyber security, they don't like the mundane tasks. They don't like managing the tool. They want to be doing the offensive stuff, hunting in people's environment, and they want to be advising the business. They want to be in front of their, their company's mergers and acquisitions team and helping to vet the company they're looking at acquiring based on their security protocols to see how hard it is or isn't going to be to roll them in the daily operations.
I mean, there's so much value. And the data that a security team gets access to, they could really be better advisors to the business. And I is going to make us require less of our time to be spent managing a tool and making sure it works. It's like having a sports car and it's just sitting on cinder blocks in your garage leaking oil.
That's no fun. I'd rather be out driving the sports car. And so we want them to drive the car instead of constantly working on it in the garage.
Adel Nehme: And you mentioned here that transition of how AI will enable cybersecurity teams to go from defensive to offensive, right? Maybe as well, walk us through what offensive looks like here. What does it mean for a cybersecurity team to become proactive about cybersecurity rather than react to threats?
Brian Murphy: Well, proactive is, we see something come in. We see an alert fire that looks interesting. Well, man, it's really valuable to say, has this happened before? Is this happening in another environment? We bought a digital risk platform last year. So we have this amazing external view of what's happening out there and outside of our data outside of our customers.
Data is something happening external that makes this more creative. And so When we can kick off searches or a, zero day event happens, or one of the large prolific attacks happen, it usually would sometimes take security teams days or weeks to figure out if their systems are implicated by a patch or an upgrade or some type of exposure.
Well, what we can do now with offensive in the right way to deploy and how we think about, we should know that in minutes, we should know that in 30 40 minutes and we can now advise our boards were not at risk or, advise our customer base. We're not at risk. And so, you know, when you think about all sense of security, It's looking for things before they become a problem, right?
And looking for little hints of things, the footprints outside your window, knowing that someone's been trying to get in that window. And I think there's just so much power and the, the security teams of large enterprise are some of the most talented in the world and leveraging automation.
To use their talents for the most creative function, the best that they can be using it is that's the, that's the gold standard.
Adel Nehme: and we're talking about here how security teams can go from defensive to offensive. We're talking about the threats generative AI poses from a cybersecurity standpoint, but also the opportunities. And I think a lot of leaders today and a lot of boards understand that investing in cybersecurity a stable stakes, right?
They've seen the rising threats from generative AI. they have more and more data, as you mentioned, that gets potentially at risk. And I think many leaders today are struggling to understand where to get started, So maybe what are key questions leaders should be asking themselves as they start building their generative AI strategy for security?
Brian Murphy: I think it's important to establish. The comfort level, what are we comfortable doing with AI? And what are we not comfortable doing? And that doesn't mean we're not going to get more comfortable over time. But I think you need a starting point. so why are we comfortable? And so it could be using generative AI and marketing.
We see a lot of those tools happening right now. But for from a cyber security perspective here at ReliQuest, the way we think about is we are comfortable Using AI to eliminate the things we know we don't need every time in a certain investigation like that, that is something that will do no harm in the environment.
It frees up a lot of time. So for us, we start with how do we make security operations more efficient? So what can we remove out of the equation every time using AI? And then things that were not comfortable, personally, that last mile is so important, that last piece of an investigation, you want someone to look at and make sure that makes sense.
Make sure there's not something going on like an acquisition or, like something that maybe that model wouldn't know about, right? We want to apply. And augment the security analyst, not replace the security analyst. And so I do think we'll continue to have more confidence along the way and use it for more.
But I think it really is important for an organization to baseline what are we comfortable with today. ideal world where we would like to get? And then how do we need to educate ourselves to move from one to the next?
Adel Nehme: And when you mention here educate yourself to move from one to the next, what do you think are steps here to reach, that point of evolution as you start integrating AI more and more into your workflows?
Brian Murphy: I think you really need to lean on industry partners. I think we have gotten as an industry, especially in cyber security, there's information ISAC groups, information sharing groups and communities. We need to really leverage the collective knowledge, especially on, you know, there's good actors and bad actors and bad actors share better than good actors.
So we've gotten a lot better at it on the on the good side of the equation. We need to share those experiences and, and learn from each other. I think that the different AI based companies have done a good job of sharing blog posts. I know we put a lot of content out so I think it's a collective effort and that's where chat GPT, when you have just board members downloading it and playing with it, that can be scary, but it's also great.
It's raising awareness of the power. It's making people more open to it. So I think the overall being willingness to share what works and what doesn't will be great.
Adel Nehme: Yeah, I couldn't agree more here. Now, one big question I think leaders have when it comes to generative AI, you know, even for Whether they're building products or not, I think this is even more foundational for cybersecurity purposes, is whether they should buy or build their own large language models, be your advice here for anyone evaluating that trade off, especially for cybersecurity purposes? And what data should organizations be looking at when training these large language models?
Brian Murphy: Well, it's, it's a lot. I think most organizations have faced this question of buy or build before and whether it's their own internal apps could be their, app in the app store, right? It could be their mainframe technology or whatever they're running on. And so I think they need to use the same systems that they use then to determine to buy the build.
is it speed you're looking for? Might be better to buy earlier days and then work towards it. Building your own. if it's your data and that data is so secretive and so important to your business, well, you want to make sure you're not sharing that out into a public forum, right?
And so I think they really need to go through. Do I have the right skill sets? Do I have the people that can build it? And when do I need to start using this? What's the opportunity cost of it taking me a year longer to build my own than using one now? And so, think you do have to be careful of privacy concerns, especially if you're in a domain with a lot of privacy issues.
That being said, most enterprise LLMs are coming with capabilities to control for that now. But it's something important to consider. So no different than back when we were just starting to write code, you know, in the mid nineties and, putting out public facing websites and storefronts, it's the same decision making processes.
Let's use our experience from those things to impact how fast we move on these things.
Adel Nehme: And, when advising leaders on what their first steps should be as they're, building out their generative AI and cybersecurity strategy, and as they evaluate these trade offs, what do you think is the first step here?
Brian Murphy: I always begin in any business issue, AI or others, what problem am I solving for? Like, why are we having this conversation? It's not that I, I want to be involved in ai, let's, let's start with the business problem I'm solving for and work with other partners like legal finance to understand both what the building of that is trying to accomplish, as well as any legal or regulatory constraints you may have.
Like, don't go down the road of building something when you haven't checked to verify that it doesn't. Trip. If you're in the financial services realm, there's a lot of just things that you have to be careful of there. So you want to check with your security teams. You want to make sure that it's well tested before we deploy it.
So, just think there for me, it starts with what problem we solving for and then making sure you have the right people around the table to help decide.
Adel Nehme: And, when we're talking here about, applying generative I think there's also a cybersecurity angle to building generative AI apps and tools, Many organizations today are racing to build their own set of generative AI tools, products, experiences for their stakeholders, right?
And they need to train it on their own data. Whether that's fine tuning an existing open source model or or, you know, training a model from scratch on your own existing data. What are the security implications of training generative AI models around your own data? And what are the type of threats organizations face here when they deploy their own models into production?
Things stuff like prompt attacking the training data, walk us through here this new family of threats organizations face as they build their own AI models.
Brian Murphy: Well, security risks with AI and ML are not unlike other security risks. For example, the prompt injections, right? They happen because of a malicious insider. It's the same threat as anything else involving a malicious insider. It's just another application. And so you really have to look at the controls that you use to build other long standing applications and processes in your organization.
And, and don't assume because AI is new and exciting and interesting that it's all that different. if it can be used for good, it can be used for evil. So it's just another application we need to treat it that way.
Adel Nehme: And what are steps here that you advise organizations that they need to do to secure their AI pipelines and secure their data pipelines to avoid threats here.
Brian Murphy: Well, third party risk is a big concern. Whose model are you using? What pipeline are you using? What are they doing to secure their application? A lot of these AI companies are high growth, fast, newly emergent technology companies. What have they been doing along the way? To secure the information.
So first, we have to get comfortable with the people that were dependent on. Second, we have to look at what data are we putting into it? Are we exposing ourself by putting data that makes us more at risk into these models? So how are we using it? What problem are we solving for? So maybe restrict it?
Maybe it is a marketing function that isn't going to get to the crown jewels of the data, the organization. And let's, let's go slow, slowly expand just like you would with other applications. It's the education of teams that just because the AI says it, the AI model says it doesn't mean we should always do it.
You've seen that in some of the law firms that have used it early on. Check the case law, check the outputs, right? So I think Be professionally skeptical is what i like to tell everybody and all things digital and security be professionally skeptical of everything you're looking at.
Adel Nehme: You mentioned here is that, you know, check your partners, right? Like, work with your industry partners in AI, whether these, proprietary or foundation models or these open source foundation models. Maybe how would you grade the current security levels of AI models today? Given the industry is moving very quickly, what's, from a cybersecurity perspective what's your view on the security of these models as they're being integrated in, systems, workflows and products?
Brian Murphy: i think difficult challenge for some of the providers and some of the third parties and AI is they don't understand what's important for your security right there building a one to many application so they're not building. This application to secure your specific use case. And what I would really think about is zoom out is when you talk to these providers and third parties.
about their models, be very specific with your use case in that model, right? And I would be very careful about the data that you put into the early models. Test it with data that they're not going to get you in trouble, and see how it works. And so I think it's an open and honest https: otter.
ai Again, there's no such thing as being 100 percent secure. Some of the models that we've looked at, they're making their best effort to build in checks and balances to build in every time. it's a sharing community. So every time they hear of something that hacks, they find a solution for it.
So that's going to naturally happen. I have seen the response of those companies be quick and be deliberate when they do hear something that's not working. So that's great. But that being said, they're not going to be 100%. So you really have to be accountable for what you're exposing your data to and know that you cannot rely on a third party to be 100%.
Adel Nehme: Okay. And, you know, we talked about this with another guest on DataFrame. In a lot of ways, the AI space is like the Wild West at the moment. A lot of these foundation models are going out. A lot of these open source models are going out. And, the regulatory landscape is going to catch up and is catching up in many ways.
Maybe walk us through from a regulatory perspective, how you view the privacy and cybersecurity conversation evolving over the next 12 months. What are you hopeful for? What are you most worried about here?
Brian Murphy: Well, I think As we understand the regulatory as those that pass the laws that are on the staff that are in the committees that are researching things, the more they understand, the more complex this issue is going to get I don't think there's, there's one policy to rule them all here.
And I do think you have to separate regulatory and compliance. From security and privacy, those are generally two separate things. And so if you're secure, you're more than likely compliant. If you're compliant and you get some assessment done, that doesn't mean you're secure. And so I think as companies wanting to leverage AI and wanting to use these things, focus on securing it.
Don't focus on what government regulation you think is coming work within the regulations that exist today and know that if you can your security team can honestly say that you are doing everything you can to secure that information in that model and you can build a case for that more than likely.
You're going to comply with whatever regulation comes out. And, and so that's generally the way I think about compliance is focus on securing your data and your compliance will come. If you just focus on being compliant, you may be leaving yourself way open for a breach or something that's going to hurt your brand.
Adel Nehme: Okay. And then, taking a step back as well and thinking about the cyber security industry overall, maybe what are you most worried about about in the next 12 months regarding AI accelerating cyber threats? And what are you most excited about about AI accelerating cybersecurity?
Brian Murphy: I'm not worried as much about too many things. I don't focus on the fear, uncertainty, and doubt of cybersecurity. I think bad actors using generative AI is no different than them using other tools for bad things. They've been doing it. There's always been this kind of cat and mouse game since the beginning the digital world, right?
And so it's why we exist in the first place is to stand on that wall and help customers make security possible. I think it's it's never just one thing that someone does that can hurt you. It's always a combination of things, which is what we're good at. So I. I look at there's opportunity to leverage AI to make us more multiple as an industry to help us see around corners to help us aggregate data at a high speed and at levels that we weren't able to previously that gives us a leg up.
And I feel great about the capability. It's a useful tool to help make security possible. It helps us increase visibility, get access to data. That in different data types and, different table fields and different table and put like next to like so we can make accurate decisions with accurate security information.
So I'm more bullish on it than I am fearful of it, but that's just me being an entrepreneur's general personality.
Adel Nehme: Yeah, no, we appreciate bullish takes here on the podcast. There's a lot of doom and gloom. Uh, So it's always good to have a positive take. Now, Brian, as we close out our episode here, do you have any final call to action or closing notes to share with listeners before we wrap up today's episode?
Brian Murphy: I point listeners to be curious, use this stuff. We publish a lot of great insights to how we're using these tools as well as trends in the threat landscape in our blog, which if people want to fear uncertainty, doubt there's people, there's plenty of that there with all the bad stuff. And so you can go to rely quest dot com.
If you're interested in learning more, it's a great place to start. I think it's a fascinating opportunity. I think it's a job builder, a job creator, an opportunity creator, and I'm just excited to see what people do with it over time.
Adel Nehme: Great. Thank you, Brian, for coming on DataFrame.
Brian Murphy: Hey, really appreciate you having me. Thank you very much.
You’re invited! Join us for Radar: AI Edition
DataCamp Team
2 min
The Art of Prompt Engineering with Alex Banks, Founder and Educator, Sunday Signal
The Future of Programming with Kyle Daigle, COO at GitHub
A Comprehensive Guide to Working with the Mistral Large Model
Josep Ferrer
12 min
Serving an LLM Application as an API Endpoint using FastAPI in Python
How to Improve RAG Performance: 5 Key Techniques with Examples
Eugenia Anello