Federico Bettuzzi is a Data Scientist at Chelsea FC, one of the top football clubs in the English Premier League. As a specialist in match analytics, Federico works with Chelsea’s first team to inform tactical decision-making during matches.
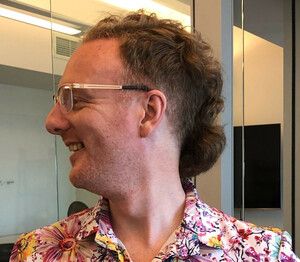
Richie helps individuals and organizations get better at using data and AI. He's been a data scientist since before it was called data science, and has written two books and created many DataCamp courses on the subject. He is a host of the DataFramed podcast, and runs DataCamp's webinar program.
Key Quotes
The big analysis project we did on set piece and open-play cross-optimization brought to life a lot of match data we were analyzing. We managed to make our analysis operational and made it work on an ongoing basis as an automated process that has led to tangible success on the pitch, especially as coaches are actively using it to make decisions in training and designing the best tactical setup for matches. I'm saying it has been successful on the pitch because, in both the 20-21 and 21-22 seasons, we have seen a dramatic improvement in set-piece performance and open-play cross performance from both a defensive point of view and an offensive point of view.
We have two main sources of data. First is the event data, which is the data of every single event that happens during a game. So, every pass, every shot, every bull carry, a clearance interception, and even red cards and yellow cards from the referee. It’s any event that is relevant in a game of football. The other source of data we get, which has higher potential, is tracking data, which provides information about players, ball positioning, and speed throughout the game at 25 frames per second. So, essentially, for each second, you get 25 observations for the ball and for each player, which amounts to roughly 3 million rows per game.
Key Takeaways
Managers have a key role role in determining which data is prioritized and how that data is used
Chelsea’s data team uses two main sources: event data, which is every relevant action taken in a game, and tracking data, which provides information about players and ball positioning.
Chelsea’s data team chooses long-term projects based on what will result in regular usage by the team and how much time they have to ensure that the project is both effective and fully-functional when it is put in place.
Transcript
Richie: Hi Federico. Thank you for joining us today. Just to begin with, I'd like to find out a little bit more about what you do at Chelsea. What does being a data scientist at Chelsea?
Frederico Bettuzzi: Hi, Richie. Nice to meet you today. Let's say then data science at Chelsea is probably not just at Chelsea probably overall in football is a bit of a niche right now. It's starting to develop quite massively, but it's still at an early stage. And let's say that being a data scientist at Chelsea is, is fun.
First of all, I would say, because especially if you are, a European football or a soccer fan is quite fun because you basically combine, at least in my case, two passions into one, which is the sporting passion and the data passion all in one. So it's really the best of both words in some way. It depends.
Most of the times it is active. Some other times it is a bit more quiet. It's a really fast paced world where you really need to be prepared for sudden changes, especially when like there's a managerial change or when there are a few things to uncover, which maybe come up from people at the top or from the manager himself.
So let's say that overall it's an experience, which is definitely, yeah, not usually if I have to put it in very high level words.
Ric... See more
Frederico Bettuzzi” The variety of problems is really huge because of course, it's also really dependent on what people ask on a daily basis. But let's say that overall in my role, since I'm working in a department, which is called match analysis, so it's basically the department which focuses on analyzing the performance of the men's first team and the opposition teams.
So basically what happens is that we focus primarily on making sure to provide metrics and analytics at the player level and team level for the first team, which can be of any use to make sure that on the pitch when the match day is on, the manager is fully prepared to make sure to know the strength and weaknesses of the opponent, players, and team as a whole, as well as.
Our own strengths and weaknesses in various aspects of the gameplay. So that's basically the high level view of what we provide.
Richie Cotton: So it's looking at like individual player performance and I guess whole team performance as well.
Frederico Bettuzzi: Yeah, it's a combination of both because of course, you are interested in knowing how specifically players perform in certain aspects. But on the other end, you are also interested in knowing how your team behaves, especially when it comes to certain tactical decisions to face certain situations.
Richie Cotton: So I'm curious to know a bit more about how do you define like what good player performance is?
Frederico Bettuzzi: That's a big question and probably one of the biggest questions in soccer overall or football overall. Cause basically it's really down to what's your own view of. A player's performance or a player being better than another player, for example. Of course, when it comes to soccer, what we look at is primarily scoring more goals than the opposition.
This is the final goal in the end. Sorry for the use of the words, the goal, but let's say from another perspective. Also looking specifically just at scoring more goals than the opposition is quite limited in some ways because of course, since we're talking about a very low-scoring game as opposed to other sports like could be baseball or basketball, of course, there are so many other aspects of the gameplay, which could be so crucial actually for individual player performance and team performance that might not be totally related to gold scoring or not considering a for example, if I have to put a, like a very simple example, like looking at defensive midfielders, looking at their performance in terms of getting the ball out of the opposition, like recovering the ball quickly in their own A, or even making sure to do proper presses in the opposition of those are kind of things which are maybe not specifically related to winning games.
Especially if you look at some kind of correlation measures, it's very difficult to find a direct correlation. Winning or scoring goals and recovering the ball. Dangerous areas of the pitch. But then when you look at a more fine-grained kind of analysis, you can start to connect the dots and see basically that recovering the ball in certain positions can lead to sequences of possession, which can then lead to creating a good chance of scoring a goal.
Being some kind of threat to the opposition. So that's one of the examples, for example, of things that are not strictly related to winning or scoring a goal, but in an indirect way, they're crucial as well.
Richie Cotton: That is really interesting. So I like your sort of comparison with basketball where it's like, okay, when you're scoring a hundred points, like the score for the team is like a pretty strong indicator of like how well the team performed. But soccer, like it quite often ends up in a. They'll, they'll draw and it's like, well, it wasn't like the team did nothing the whole 90 minutes, they actually added value somehow.
So it seems like for strikers or like for the forwards, it's like kind of clear like how you measure the performance. Like do they actually score goals or take shots and things like this, But for defenders it's a bit more fuzzy. So maybe can you give an example of like, do you have a sense of how you go about measuring how they ended value to the.
Frederico Bettuzzi: Yes. Let's say that probably in terms of defensive players, like one example is the one I provided before, but other examples could be, for example, when you look at. Certain specific gameplay situations, let's say when players need to defend open play crosses or set pieces, situations like corners, like in this case, when you are facing these situations from an opposition perspective, of course you need to find a way to try and minimize the amount of goals you concede from this situation or minimize the amount.
Threats you actually concede. So in these scenarios, for example, making sure to measure performance around our defenders, effective in winning jewels, for example, winning I area jewels, or making sure that like to measure performance around clearing the ball out when there's a cross inside the box. Or when it comes also to looking at more specific kind of information around spatial information of defenders, for example.
You wanna make sure. Depending on the situation, the players are positioned in an optimal way, where optimal, of course, is a very fuzzy word in some way, but optimal is always down to what we consider to be optimal and also what is effective, let's say, for the coaches which study this particular situation, set up, this particular situation on the pitch to know what to do.
Basical. In training, for example, when they want to make sure that knowing the characteristics of an opponent in terms of where the players tend to attack in certain kind of situation or how they tend to move, you know, which kind of positions you have to keep and which kind of movements you might expect.
Richie Cotton: It feels like midfield is maybe. Even like a harder thing to analyze. Cause it's like you don't have the obvious, Well, I'm either gonna score something or I'm gonna stop someone scoring. But it's sort of somewhere in between. So can you talk at all about midfield? What do you do there?
Frederico Bettuzzi: Yeah, you are definitely right about that. Cause first of all, the midfielders are probably the most right kind of roles you can have because of course you. As set up with three midfielders or two midfielders, or even four midfielders in some occasion. But of course the kind of role they play in the game can be very different.
Some midfielders are primarily defensive midfielders. So often their role is stopping the position from advancing like. Being like good pressors or making sure to intercept dangerous balls. Other midfielders have more of an attacking role, so perhaps midfielders that starts wide. And then they are ball carriers that like to carry the ball towards the opposition box for a shot or potentially to try and play a pass, which.
Breaks the lines, the defensive lines for examples and other midfielders prefer like to run without a ball. So doing off ball runs, which can then be in some way targeted by other players, which are more into playmaker kind of role. So there's these different sides. So of core, depending on the. Midfielder you are looking at, you might look at different metrics for, let's say attacky midfielders.
You might potentially still look at some kind of defensive metrics because of course, attacky midfielders are probably also amongst the first players. That puts the first line of pressure in the opposition half or in the opposition third, when they are closer to the opposition box. But you wanna also look at more some kind of creative stats, such as how creative that player is in terms of playing.
Potentially dangerous passes where the dity, the danger of a pass can actually be measured by using some, in this case, some kind of model, some kind of machine learning model, which can actually be used to predict, let's say, how difficult that pass can be or how potentially threatening that pass can be.
In order to get to a certain position. So creative stats, defensive stats are the kind of stats you wanna look primarily for midfielders, potentially Also scoring stats if you wanna consider very offensive midfielders, such as a number 10, playing behind lone striker, for example.
Richie Cotton: So it seems like there are a lot of d. That you can sort of calculate, like for each of the different roles. I'm kind of curious like what happens after you sort of crunch the numbers. So if you discover like one player is playing particularly well or particularly badly, what sort of happens in the club?
Frederico Bettuzzi: That's actually the part in which probably I'm more behind. The sort of scenes in this kind of work, cuz of course I'm responsible for doing all the, let's say, maybe not so exciting work in terms that you are not facing, let's say the players directly when it comes to communicating. Then the kind of results, I'm more behind the scenes, but when it comes to actually the final end product of the outcome of the analysis, basically what happens is that usually the coaches.
So the manager staff and the manager themselves actually have usually meetings with the players on the day or two days after the match where they analyze the game with both videos. The analysis we come up with to actually trying to spot the positive things and the negative things of. The previous game, essentially.
For example, in the game we had on the weekend against, right. And there were lots of negative things to look at. Of course, without going to much into the detail by uh, just looking at the score line. Of course we had a few things to look at. But yeah, at the end of the day, you always have, of course, where to be a bit cautious about how to communicate these things to the players because it's always about some kind of trade off.
Of course, you know, you don't want. Be too harsh with them if they've done something bad, but on the other end, you wanna make sure that they underst. Where they can improve, but also highlight what they've done well in the game cause. So from there you can actually take it and also use the training, kind of setup, the training session to actually train the players in those kind of aspects.
Especially when it comes like in set pieces, you can train players to kick set pieces more effectively or to learn certain patterns of play, for example.
Richie Cotton: I can certainly imagine that like after you. Game having to like tell all these sort of players who've been hire from billions of dollars, actually the statistics show that you will rubbish today. That's gotta be like a difficult conversation. So maybe it's best it comes from the coach rather than yourself.
Frederico Bettuzzi: Yeah. Yeah, absolutely.
Richie Cotton: I'm kind of curious as to like how the sort of communication flow works. I know like English football in particular is like traditionally had like kind of anti-intellectual reputation and so I dunno how well like analyst communication comes across. So can you maybe talk a bit about how you explain the results of your models and your statistics?
Frederico Bettuzzi: I wasn't aware of that kind of aspect about.
Richie Cotton: I mean, I think this is more like a 20th century thing, but
Frederico Bettuzzi: Yeah. No, that's good to know. I mean, I would say that, of course, different managers have different views and ways of working, especially with our kind of role, which is very analytical. But in general, I would say that probably we are way more into direct connection with his assistants. Their assistants. So the assistant coaches rather than the manager himself.
Usually the manager himself actually is a bit more, I wouldn't say on, on his own, but he's more direct with the players and of course with these closest stuff. And we are more in connection with the coaches, the stuff which is actually helping the. The workflow is very direct with them because actually we usually meet them daily on a daily basis.
We meet with them on random occasions during the day, so it's not like a set meeting every day like 9:00 AM meeting. It's more like they come into the office, we say a lot to each other, and then we just start talking about. What we want in terms of we wanna see this, this, and that today when it comes to the daily kind of operations.
Because at the end of the day with the coaches, we are more into the daily kind of metrics or daily kind of work. Whereas when we talk about a longer view, so a longer term kind of planning for the work we do on a longer. It's more down to us, specifically us as a department, planning things beforehand, planning things which still gone alongside daily things.
And sometimes you start them, then you leave them aside because you need to do something else which is more urgent. Then you pick them up again. So that's the sort of thing we do with the coaches and bombs ourselves as.
Richie Cotton: You've just had a change of manager quite recently. And so does that affect, like do you get new directives on things that you need to be looking into? Uh, different kinds of analytics, like depending on the manager.
Frederico Bettuzzi: Yeah. Yeah, actually there's actually, I've been with three managers since I've been at Chelsea, so I could see three different ways of working and yeah, I would say that all the three of them were quite different amongst each other. I would say probably the first manager I've been with, which is ARD and the current one are quite similar probably.
Whereas TOIL and these assistants were quite different because they had a very clear view of what they wanted to see, so they have very clear view of what they wanted us to do. So let's say that we knew very well what to do, but we were in some way also constrained to do what they wanted, which is a good thing.
Because they had very clear ideas. But still, let's say that in terms of working for longer term kind of project, it was a bit more complicated because they knew very well what they wanted. So we were full-time working for them, basically. Whereas now there's a bit more of. Let's say freedom because, so I mean, they're very open to what we provide to them.
So basically we have more kind of freedom in working on our things in some way and provide them with things that can in some way stimulate their intellect and stimulate a conversation amongst us. So let's say that. That's a good thing Also as well, I would say that I found myself well with all the three of them because in some way they were all different.
But at the end of the day, that hasn't really affected us in terms of our daily workload. I mean, We always had something to do, which was relevant for the club and for the team as well. Something that allowed us to actually play a significant role also in a few situations. So I would say that that's something actually I can complain in terms of the overall relationships with the managers and their staff so far at least.
Richie Cotton: That's good. Mute
Frederico Bettuzzi: Yeah, that's very.
Richie Cotton: So you talked a bit about the difference between the sort of short term projects and the longer term goals that you have. Can you just maybe, uh, give some examples of each and what the difference.
Frederico Bettuzzi: Let's say that when we talk about planning the long term kind of things, these are usually things that probably are discussed in the summer break. So basically when all the championships are over, so in the month, around June and July, which are very quiet months for us, cause of course the team is basically never there.
We have a bit more time to basical. Plan the things we wanna work on, which decide ourselves alongside the season, which are a bit more, also research and development kind of things, which can actually be used also on a daily basis, but can be worked on a longer term view. So we can actually say, Okay, we give you six, seven months to work on this.
Piece of work where you can take your own time and developing things which are actually working nicely. We are sure they are a hundred percent nailed down. We have no rush about doing that on a very short term, like within one month. We're sure that when they are in place, The club can use them on a regular basis and making sure it is effective when it is in place.
So that's the kind of long term view. One example of these, actually back in the days when actually the pandemic kicked in, so we're talking around March, April, 2020, there was the big work we did around the set pieces, the big work about around set pieces, which I can maybe discuss a bit. And when we talk short term, it's very, very short term.
So it could be short term of, with a term of one week, for example, or even less than one week because it really depends on, on what the manager or the staff come wants in terms of, okay, we want to see this in within a few days, or as soon as possible at least, or sometimes. Find myself working on pieces of work, which literally last one day.
So I work on one piece of work, which is ready in one day. The next day is already operational, or maybe it's not operational, but there's already something to work on. And then to make it operational, let's say on a bigger scale. And with this sort of automation you wanna. See also on a game day basis, that's something that maybe takes a bit more time.
I found myself working on very short term things like working on, like making sure the metrics were provided live during games, some simple metrics which we wanted to see live in game to make it operational. It took a bit of time because we needed also the help of an external developer, which was more into making sure.
The scripts could run on an automated basis externally in a platform, but to make sure that they actually just, the metrics were available in game on a sort of live scenario, which wasn't really fancy, but was still working. That was basically done with in a matter of days.
Richie Cotton: That sounds first, I'd love to know a little bit more about what these sort of metrics you provide live during a gamer.
Frederico Bettuzzi: I mean these live metrics, actually this is, this was a very short term piece of project, which basically started and actually was nearly concluded with the former manager. So we took, and this stuff, they wanted to. A few metrics, which were very high level, simple metrics, both at team level and player level, but they were detailed enough for them to actually see them on a sort of mobile app, which they could open and refresh whenever they wanted, and they could see the progress of this metrics during the game.
For example, Very simple metric, which. Very well used now is, for example, being able to see XG expected goals live in games, both at player level and team level for a specific game. So basically you could see by refreshing this app, how the sort of cumulative XG was at that point in game for. Our team and our pulling team, but also specifically for each player.
And the nice thing was also that you could see this metric also specifically for certain gameplay situations. So you could split it also amongst open play situations and set PC situations like corners, for example. That's an example of a metric.
Richie Cotton: So this Xg, this expected goals, this is a sort of measure of like what the statistical model would predict the number of goals to be. Cuz that's often like quite different from the actual number of goals. Right?
Frederico Bettuzzi: Yeah, absolutely.
Richie Cotton: Could you just talk a bit more about like how this sort of thing's calculated?
Frederico Bettuzzi: The expected goal is usually going up a bit more technical into the kind of model, which usually it entails. The model itself is a classification model. Each line of your data is a shot from any game, so you, you collect. A significant number of shots throughout a big number of games slash seasons, and you have a few characteristics about these shots, which are your features of your model.
So it could be the shot location, the sort of situation, whether it is open play or set play. Another characteristic could be the angle you have towards goal, or the number of opponents you have between the shot and the goal. So a few features that describe the shot and this feature I use to predict whether the shot ends in a goal or not.
And of course, at the end of the day, the characteristics such as the location of the shot is probably one of the most explanatory feature because of course, the location of the shot gives you also information about how distant you are compared to the goal. So the distance from goal, of course, plays a big role because the closer you are to.
The more likely you are to score in most of cases. And basically at the end of the day, it shot as a probability of ending to goal attached. So a number that ranges from zero to one, and by summing all these probabilities, Alongside a game, for example, you get the cumulative xg, the cumulative expected goals throughout a game.
So your total number of goals you should have scored in a game roughly based on the model. You can compare it along a big number of games, or even in the same game, you can compare it alongside the actual number of goals you scored. Both at player level and team level and see if you have either overperformed, which means you have scored more than you should or underperformed.
You have scored less than you should.
Richie Cotton: So this is really like a measure of, well, some shots are easy to score, some goal three, easy to score, so you. Try and optimize for getting to those. That's interesting. So does it feed into strategy then? So you feel like, well, okay, there's no point in just like trying to aim for taking long shots and actually we should work towards some kind of tactics where we can get in closer to the goal.
Frederico Bettuzzi: Actually, yes they are. They're actually use, I use a lot strategically, especially depending on the situation you want to analyze. Because for example, you want to use expected goals as some measure of threat. So rather than using expected goals as a measure of what's the probability. For me, scoring a goal, it's more used as a metric to say we wanna move the ball into more threatening positions, and we wanna make sure also, when it comes to playing certain situations, such as playing across or playing a set piece like a corner, you wanna make sure that.
When you play the ball, both the crosser and the players are actually positioning themselves within the box. You wanna make sure that they actually position themselves in such a way that we can maximize our global expected goal from the situation. So looking at things like. What's the situation that allows us, what's the combination of features, of combination of steps that allows us to maximize our chance of shooting and maximize the chance of having a high expected goal shot?
So basically combining the chance of shooting. So getting the quantity in it, but also the quality. Cause there can be situation in which you might have more shots on average, over a longer sort. Time horizon on over a high number of data. You could see that on average you can shoot more in certain situation, but the average expected goal of those shots might not be so high, whereas there might be other situations in which you have fewer shots.
So it's more difficult to get a shot, but once you get this shot, the chance of getting this into goal is higher. So that's probably a perfect example when you. Out swing corners versus in swing corners, for example. So out swing corners, like corners, that player kicks and tends to go further away from the goal.
Whereas in swing corners are the ones that tends to go closer to the goals. For example, in out swing corners, you tend to see more shots, but we have lower XG on. Whereas in in swing corner, so the corners the goal towards the goal. It's more difficult to shoot, but the shot tends to be higher in XG because actually the ball actually also gets closer to the goal.
So that's also another thing to consider. If you get the ball closer to the goal, you are threatening crazy, but it might be more difficult to shoot because there's also more density of players.
Richie Cotton: That's really interesting. So there's a sort of trade off there, like the thing where if you're just counting like how many shots do you take, then you'd probably favor going for the Outswing corners. But actually you are gonna have more chance of scoring if you go for the Inswing corner, even though you're not gonna get so many shots.
Frederico Bettuzzi: Exactly, and especially it can be this average, these numbers score will be different from team to team. So that's also where the. Individual opponent analysis comes in when it comes to deciding what's the best way to try and maximize our chance of scoring at the end of the day.
Richie Cotton: I'm curious, how do short corners compare to these when you just knock.
Frederico Bettuzzi: The short corners in another differ is another interesting point because short corners, for example, if you look at the global kind of output you get from short corners, they seem not to be very effective. On average, it's very difficult to get a shot from a short corner. If you compare it of course to the standard in swing or outswing corners for an obvious reason at the end of the day, because with in swing and outswing corners, you are getting the ball into the box straight away for free in some way, so you don't have the additional step of moving the ball further away and trying to find another spot to get the delivery into the box.
Because often also short corners don't really. End up into the box, they become some kind of build up phase. So the short corner becomes at some point an open play situation. So that's also another thing to consider that actually short corners in most cases, end up being an open play situation. And so anything that comes from that situation.
He's also classified as an open and play kind of output and not a set play at some point. So that's also another thing to consider. But there are some teams that are actually better than others in playing short corners and getting something out of it. Although at the end of the day, there's not really much data for short corners as opposed to swinging and outing because it's not something that teams on average tend to apply very often during a.
Richie Cotton: So it seems like corners are a pretty well studied like part of soccer analysis. How about other set piece? Is What about like penalties or free kicks?
Frederico Bettuzzi : With free kicks. We do basically the same kind of analysis we do for corners, so there's really nothing different. Methodologically, of course, free kicks can be kind of big variety of free kicks because depending on where the free kicks are taken, you might study them differently, like lateral free kicks, which are the kind of free kicks where.
Actually the ball is kicked from wider positions, especially in a sort of upper part of the pitch, but wider, which nearly resemble corners in some ways. Although if the ball is not high enough, actually the way defenders or players overall need to defend or attack is quite different. As you can also probably see in some videos from S as opposed to calls, for example.
Cause you see actually the ball coming towards you. So you have to. And behind towards the goal. Whereas in most cases, for corners, you don't need to run in behind because the ball is literally coming from the byline. So in that case, you don't need to run in behind, and that's another big difference between lateral free kicks and corners, for example.
Then you have other free kicks, like frontal free kicks, which are. Basically played from frontal position a bit further up on the pitch, so a bit behind. So in that case, the analysis is still different and other free kicks where free kick shots are basically, there's not much to analyze in there because even freaking shots do not happen very often in a game.
So even for that kind of analysis, it's, it is difficult to gauge something on. Bigger scale because at the end of the day there are shots so they can be classified within the short realm in some way. And penalties is actually something we don't really focus too much from an, at least from my analytical point of view.
Cause penalties are, again, quite rare events. Of course, once you get a penalty. We know the chance of scoring a penalty is extremely high. So of course you wanna make sure to get it right. You wanna make sure to have that possibility if you can. But on the other end, there probably penalties is one of those things which we probably could look at at some point, especially from a goalkeeper perspective.
If you wanna. Like feed goalkeepers with relevant information for certain players, which are actually way better than others, on averaging, kicking penalties, studying the kind of techniques, studying the kind of direction they take when they take penalties. So do they take penalties towards the left or towards the right with the right foot or left foot?
So these kind of things can actually be helpful, but in terms. Big data. It's not something that we focus too much. Also, because you can also use purely videos for that when you study a specific player, for example, because usually you might have not so many penalties to study for a specific player.
Richie Cotton: So in that case, it sees you just watch like a dozen or so just videos of them diving and then you figure out, oh, they can't dive left or whatever.
Frederico Bettuzzi: Yeah, Which is done by other people usually. But yeah, especially, yeah, if you have like over a career you might have that a player has taken 30, 40 penalties, 40 penalties already, a big number for a player over an entire career. So in that case, you can easily watch them on video 40 penalties.
Richie Cotton: I'd like to talk a little bit about like the data and how you come to sort of create all these analyses. It seems like using some video data, but beyond that, where do you get all the, the rest of your data? Of, uh, analysis. What's it look?
Frederico Bettuzzi: Basically, the data I work with are primarily coming from two main sources. One source is. The so-called event data, which are basically the data that provide in a sort of time, serious fashion, every single event that happens during a game. So every pass, every shot, every bull carry. A clearance interception, even red cards, yellow cards from the referee.
So anything foul committed, foul receive. So any sort of event which is relevant in a game of football, in a game of soccer, and the other source of data we get. Which is the, probably also the very interesting one because it has the highest potential, is the tracking data, which provides basically information around players and ball positioning, as well as speed throughout the game with a resolution of 25 frames per second.
So essentially for each second you get 25 observation for each. Player and the ball as well. And this information, which clearly amounts to some 3 million roles per game, roughly, it's 3 million roles per game at the end of the.
Richie Cotton: Pretty big data.
Frederico Bettuzzi: Yeah, especially, yeah, if you just consider one game. Yes. So basically, if you consider an entire Premier League season, let's say, you can easily exceed 1 billion rows.
So basically, when it comes to storing, of course we're talking about storing very big data, not an excessive amount of columns. So at the end of the day, the width of the data is quite limited in a good way. The number of roles. Quite explosive in some way. The use we make of this data is quite huge, quite valuable because it, with all the things, for instance, I've already mentioned, which is only a tiny part of all the things we work on, we have already used these two sources in a quite significant manner.
For example, to mention again, the big work we did around the set pieces and crosses. Overall, this is probably. Bigger project we have done overall because also it has been a project that has lasted until its final version. It has lasted probably a good six or seven months, if not probably more at least.
And this big project was literally about trying to understand how to maximize our effectiveness in all set pieces and crosses situations in terms of offensive and defensive. So basically what we did was using the event data to identify the moments in which in the game there was a set piece, like a corner or a free kick, and any open play cross as well.
We have throughout a game, so also open play situations like crosses are taken into account and once we identify these situations from event data, We use the trucking data to identify a reasonable window of time where we believe the specific event will fall into the trucking data. Because one big limitation of this big alignment thing is that the two sources are not.
Synchronized from a time perspective. So basically we needed to find ways, some fuzzy, very fuzzy logic to make sure that the window of time we have, we identified the tracking data was matching the event. We were identifying event data as precisely as possible, and that took a big effort from me in terms of trying to find.
The right context. So basically by using the player, for example, that was kicking the corner of the cross in the event data, I was making sure that that player was in the right context in the tracking data. So that player was actually very close to the ball in a sort of timestamp, which was reasonably closed with timestamp.
We got from event data and also trying to work out what. Optimal approximation for the ball to actually leave that player foot to actually getting delivered. So this kind of logic is the logic I've actually used to try and align with these things as best as possible. And also a big effort from my colleagues in my department to validate these things with videos.
So looking at videos of. Of course randomly sample the events because looking at thousand and thousand of events all over and all over again would've been a mess. So trying to cherry picking events to try and find out the optimal sort of validation. And there was a lot of back and forth on this, so me doing.
Sort of alignment to try and align the two sources. Then validating with video saying we could maybe refine the rule. So going back to the code and refining the rule. So there was a few times this back and forth kind of situation between me and my colleagues, which was frustrating sometimes, but at the end of the day, rewarding because we managed to do something quite big at the end of the day in terms of this big work, which also proved to be.
Quite effective on the pitch as well in the past season. In the season before as well, in terms of getting our output in terms of offensive and defensive situations. Right. Or at least getting it better.
Richie Cotton: It seems like you were saying that like the two main data sets are kind of like time series event data, and then sort of the spatial data as well, and there's like a bit of a data cleaning problem to try and get the two things aligned.
Frederico Bettuzzi: Yeah, absolutely.
Richie Cotton: Can you tell me a bit about which statistics or machine learning techniques you tend to use?
Frederico Bettuzzi: I would say the, apart from the large majority of time I spend on proper data cleaning or proper refinement of data rules, which are actually essential to make sure that we actually work. On the logic we need to actually work on to make sure we get to the final goal we need for the specific analysis.
For example, making sure the certain sequences of possession, in event data reflect what we actually see on video, which is sometimes not right because the event data don't fully reflect sometimes what you see on video because they are humanly tagged. They're tagged by people. So of course that brings in an unavoidable human error apart from the huge data cleaning.
I carry on on a daily or monthly or weekly basis on a regular basis. The machine learning techniques I use to actually more on a kind of research and development phase at this stage, which hopefully at some point will make the light of the day on an operational phase are, for example, related to. Pure analysis on tracking data.
For example, identifying patterns in the tracking data, which reflect player runs. For example, behind the defensive line, especially when you want to identify moments which you will never see in the event data. Because in the event data, you only see information around the ball, whereas in the trucking data you can.
The full spectrum of actions around specific events. For example, in the tracking data I'm working on, making sure to identify those runs, those player runs, regardless of whether they are around certain events or not. The players do. And they often do on a game, even when they are off camera sometimes, which is even more of a bonus for me to investigate this data even more because you can identify moments such as these, which are actually difficult even to quantify from a metric perspective, because actually you don't really see them on an event data feed.
And even in the tracking data, if you use them purely to align them with. Event data feed. In terms of contextual information, it is still difficult because sometimes you see things in tracking data like these runs, which are very difficult in some way to attach to a specific event. So in this case, that's where this kind of information can be very useful.
Also, from a recruitment perspective, if you wanna identify players for recruiting purposes, which are actually doing specific kind of things on the pitch, which are not so easily quantifi. So that's an example.
Richie Cotton: Things on the pitch, that means basically stuff that's happening away from the ball. Maybe like a player trying to create space or something like that.
Frederico Bettuzzi: Yeah, exactly. That's the big point around, uh, creating space with runs.
Richie Cotton: And so of all these analyses you've worked on, what's the sort of data success story you're most proud of?
Frederico Bettuzzi: I might be repetitive once again, but yeah, probably the big project we did around set pieces and crosses in terms of bringing to life, let's say probably to nearly full potential, the tracking data and event data together. It's probably something that really made us proud and not only because it was a big piece of work, which we managed to make operational and we managed to make it work on a regular basis.
And it's still working nowadays in terms of the automated process, in the background, but probably we are also very proud of that because of the actual success we have. On the pitch for this kind of data brought to life, especially when it comes to the coaches using it actively to actually take decisions in the training session when it comes to train players for certain situation, but also on the pitch when it comes to designing the best setup during a game.
I'm saying it has been successful on the pitch because actually when it comes. Past season. So the season 21, 22, and the season before the season 20 20, 20 21, we have seen a dramatic increase, a dramatic improvement in set pieces, performance, and also crosses open play cross performance from a defensive point of view and an offensive point of view.
So in terms of scoring goals and conceding goals, as opposed to the season before that. So the season 1920, which was a very. Problematic season in some way in terms of set pieces performance. Then in two years ago, and one year ago, also thanks to a new set piece, coach that came in enabled us to actually have a more thorough kind of analysis and a more thorough training on set pieces that enabled us to actually be very collaborative within.
To being very cooperative with him in terms of working very well alongside him because he, he really liked the kind of work we did a lot because he's quite a bit into data in terms of using data to actually take decision. And so using his expertise in terms of. Training players in set pieces and crosses from a defensive and offensive point of view.
Combined with our work we did with set pieces and crosses, which was completely independent of any manager request. So it is something we planned ourselves in some way. We managed to create this small success store in some.
Richie Cotton: That's cool then. So it seemed like analyzing set pieces and optimizing set piece performance is like a very big part of your role. So just switching to the World Cup, because the World Cup has got an important event right now, it seems like national teams and national squads are also very much focused on like trying to figure out set pieces as well.
So I'm just wondering, do you get involved in World Cup analysis?
Frederico Bettuzzi: Actually, I'm not involved at all in World Cup analysis, at least not in the terms of analyzing the games themselves, because actually since. Chelsea specifically is not involved in this competition, and it's also an international competition. So international teams are involved. It's usually something we are not involved also because to that end, we would need actually to buy data to actually get analysis.
So in that case, it's also another thing to consider. So I'm not involved in the analysis of the World Cup, at least in terms of getting data and crunching metrics as I usually do when it comes to our competition specifically. But it might be that in terms of recruiting, potentially also because I collaborate also with the recruiting side of things, we collaborate with the recruiting side of things with our data because our data are also used for recruiting in certain cases, which is going to help Definit.
Richie Cotton: So this is trying to identify good players in order they might join.
Frederico Bettuzzi: Yeah, of course the World Cup will be a, a very big opportunity for recruiting purposes. So I assume there might be some data analysis coming up. I dunno if we, if we will be to the level we have been facing with our competitions, but still we might still see something. We see. We just wait and see.
Richie Cotton: I guess finish, Who do you think is gonna win the world?
Frederico Bettuzzi: My answer might be quite obvious, but I'm guessing Brazil.
Richie Cotton: Okay. I think they are favorites of winter. You going with it? The safe choice there,
Frederico Bettuzzi: Yeah, I would say it's a very safe choice. Um, I've actually watched Brazil a few times also because my girlfriend is half Brazilian, so also I'm a bit biased about that and basically, of course she's, she will support Brazil a lot. I'm very, I'm very kind of, I'm biased on that. I'm not supporting a team also because Italy is not in the World Cup.
So , that's a big loss for us for two World Cup not being in there, which is very massively negative for us. But I think Brazil overall are really the most complete team. Especially consider that France. We have quite a few big defections, so I would say that that's the main reason Brazil seems like the more complete team in every kind of setup, defensive setup, midfield and forwards.
I think they are the most competitive team, but work Cup is good also because often you see very unexpected teams doing very well, like Croatia, four years.
Richie Cotton: All right. In that case, we've got a few weeks to wait until we find out if your. Are correct. So thank you for, uh, taking the time to chat. It's been a a real pleasure. I've learned a lot. Thank you.
Frederico Bettuzzi: Thank you very much for your time, Rich as well. It's been a pleasure.
An Introduction to Data Orchestration: Process and Benefits
Srujana Maddula
9 min
[Radar Recap] Building a Learning Culture for Analytics Functions, with Russell Johnson, Denisse Groenendaal-Lopez and Mark Stern
[Radar Recap] From Data Governance to Data Discoverability: Building Trust in Data Within Your Organization with Esther Munyi, Amy Grace, Stefaan Verhulst and Malarvizhi Veerappan
[Radar Recap] Scaling Data ROI: Driving Analytics Adoption Within Your Organization with Laura Gent Felker, Omar Khawaja and Tiffany Perkins-Munn
Using Snowflake Time Travel: A Comprehensive Guide
Bex Tuychiev
9 min