How Data Science Can Sustain Small Businesses with Kendra Vant, Executive GM Data & AI Products at Xero
Dr Kendra Vant, Executive General Manager of Data & AI Products at Xero, is an industry leader in building data-driven products that harness AI and machine learning to solve complex problems for the small-business economy. Working across Australia, Asia and the US, Kendra has led data and technology teams at companies such as Seek, Telstra, Deloitte and now Xero where she leads the company's global efforts using emerging practices and technologies to help small businesses and their advisors benefit from the power of data and insights. Starting with doctoral research in experimental quantum physics at MIT and a stint building quantum computers at Los Alamos National Laboratory, Kendra has made a career of solving hard problems and pushing the boundaries of what's possible.
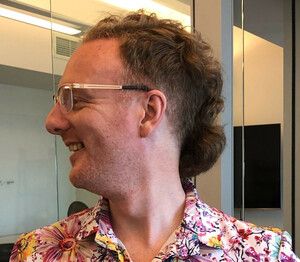
Richie helps individuals and organizations get better at using data and AI. He's been a data scientist since before it was called data science, and has written two books and created many DataCamp courses on the subject. He is a host of the DataFramed podcast, and runs DataCamp's webinar program.
Key Quotes
I think that it is true now and it will only become more true over the next 50 years that being comfortable and confident with data, with what it means, with how we treat it well, being able to spot when someone is lying with statistics, being able to understand how to use statistics, to understand more about it is only going to become a more important part of the working life of every person listening to this podcast.
I'm really excited about helping people have the confidence to do things, making use of all of the tools that are emerging and maybe using this tipping point with techy beauty about language models becoming part of the vernacular to reinvigorate your confidence, reinvigorate your thirst for learning and say, I've got 40 years working ahead of me, 50, 60 years.
We're all going to be working for real long time, right? Because we're all going to live to 100. We're already working through our ages. How do I build into my sort of my patterns, my habits, a thirst for learning, a thirst for reeducating myself and reinvigorating my career prospects? And how do I reach out and use those incredible online resources to become more confident use of data?
I'm passionate about that because I think it really will assist millions of people to become confident, literate and economically productive. And I'm also excited about it because it feels like now is a time when people are being exposed to a much greater extent than they have been. And so it's a good time to make that mind shift and to say, okay, I'm going to jump on the bandwagon and I'm really going to learn.
Key Takeaways
As small businesses grow, they must be aware of their increasing responsibilities as employers. Data can help in ensuring compliance and understanding obligations, especially in areas like payroll.
Identify patterns with data - Even without extensive data expertise, small businesses can extract valuable insights from their records, such as recognizing seasonal ordering trends or time lags, to optimize operations.
For enterprise organizations building B2B products, AI should be viewed as a tool to improve customer experiences, not just as a standalone technology. Integrating algorithm and product teams can lead to a more seamless and intuitive user journey.
Transcript
Richie Cotton: Hiring a single data scientist is often out of the question. That means that there's a completely different set of problems and solutions that small businesses face when trying to get better at working with data. Today's guest is Kendra Vand, the executive general manager of data and AI products at Xero.
She's responsible for developing data and AI features. for Xero's accounting platform for small businesses. Kendra was previously a data leader at Deloitte and Telstra and gives advice about data to the Australian government as a member of the National Data Advisory Council. I'm keen to hear Kendra's insights into how small businesses can make better use of data and artificial intelligence. Hi, Kendra. Thanks for joining us on the show today.
Kendra Vant: Hi, it is a real pleasure to be here, Richie.
Richie Cotton: We spend a lot of time on the show talking about enterprise data, and so I'm quite excited to hear about the opposite, to talk about what goes on in small businesses. So I guess just to begin with can you tell me what are the main use cases of data in small businesses?
Kendra Vant: Oh my goodness, that is a wide ranging question to get started with. So Xero's original premise is to be the backbone of the back office small business. So we offer the general ledger in the cloud. So effectively, it doesn't matter what kind of small business you're running, in almost every jurisdiction, you need to pay tax.
And hopefully you will be profitable. And so to do... See more
Inventory, the financing, all of the things that perhaps you didn't go into small business to do, but if you don't do it well, you won't stay in business for very long.
Richie Cotton: So it seems like the place to get started running business really is worrying about what's the financial data and what's happening with that and making sure that you are doing your payroll and your taxes correctly.
Kendra Vant: It's sort of one of those things that you want to spend as little time on as possible, but as much time as necessary. Because it's not why you went into business unless you happen to be an accountant or a bookkeeper. But it is really, really important to make sure your business runs well. And probably a little different from country to country, but where I am today recording in Australia, of businesses in Australia are small businesses.
So it is an enormous part of every economy.
Richie Cotton: I hadn't realized it was, it was so important that I suppose you ever anything about it, there are an awful lot of small businesses around. And I like the idea that it's something that you want to spend sort of just enough time on because sometimes you get overexcited about data here. We're like, oh yeah, everyone should do more, more things with data, but often if you're a small business owner, maybe it's not where you intended to be spending your time.
But we'll get into that maybe in more detail in a moment. But first of all, have you seen any success stories from small businesses making better use of data?
Kendra Vant: So I, one reason I love working at Xero is I love The fact that many people I run into in the street aren't users of our software. So I can quite genuinely say that people say, Oh, where do you work? And I say, I work at Xero. And your hairdresser, your plumber, your little coffee shop, they genuinely say, Oh, I love Xero.
And the reason is what we were just touching on. I love Xero because it makes my life easy. So I would say there's a general high tone of I like it. Because it reduces the time I need to spend on those things. If you then dig deeper into success stories, for me, probably if I think about some of the conversations I've had, some of the perhaps slightly larger small businesses, if that makes sense, who have been running for a little while, so now that perhaps they have a couple of years worth of inventory or a few years worth of repeat orders, they actually find Even the ones who perhaps aren't data savvy by nature when you sit down with them and say, well, do you know a wealth of information that now sits in the records that you've been keeping with Xero?
They actually have gone through and discovered things like order time lags or customers who order in a seasonal pattern and then have been actually able to reshape their ordering or their staffing to meet that demand. So yeah, when people are perhaps given a little bit of a nudge. For the folks whose businesses aren't data oriented, but you give them a little bit of a nudge and you say, think about what actually now sits in the records that you have.
Usually what you hear people say is, I can be more efficient. I can serve my customers better. Or I can save money, all of which are wonderful things if you're a small business owner.
Richie Cotton: Absolutely. I love the idea that just being able to surface things like seasonality or order quantities can make a huge difference to someone's livelihood.
Kendra Vant: And it's the thing, right? We think about it for big businesses, particularly, I'm sure many of the folk listening to this podcast who are data practitioners, and they think about this all the time. And many of them will work in large businesses, optimizing for millions of customers. Now we have millions of customers ourselves at Xero, but our customers are small businesses.
They don't tend to, but they can take those ideas. of looking for patterns in enormous amounts of data and look for patterns in their smaller amounts of data and it's still really, really valuable?
Richie Cotton: Absolutely. Something that sort of strikes me is if you've got one or two business owners who are doing everything, Then you're not going to have the resource to have a data specialist to help you look at all this stuff. So how can you make effective use of data when you have this limit to your resources?
Kendra Vant: It's a really good question and I think we would try to answer that in two ways. One is to encourage people that when they use a product such as Xero their data is actually quite tidy and quite neat. And many people listening to this podcast will be very aware that the large amount of time spent for a data practitioner is needing up your data.
So for folk who are using a cloud software provider for doing their accounting, actually a lot of that neatness has occurred. So that, if you're using multiple banks, you'll have pulled it into a single bank, et cetera, et cetera. The other side of it is we are always, as a company, striving to make this more simple for our customers.
So we build products. That do that and deliver them through zero. So for me, for my machine learning team, my AI products team, we have two mantras that we build towards. One, remove toil, use the data and the patterns from the data to make small businesses life easy. And two, Delight with insight. If we can pull patterns that say, hey Richie, you seem to come in every Thursday at eight o'clock in the evening and these are things you do, so we're going to queue them up for you and suggest to you that this one we've already taken care of, this one we have a suggestion for, and this one, oh, this supplier plays late.
So we suggest that you go and nudge that supplier so you can make payroll next week. That's going to make your life easier. So that would be, I'd say, the two sides of it. One, the data is tidy for those human beings who would like to delve into it, to find their seasonality patterns and their big customers.
And two, all good SaaS software providers should be working on that data to pre provide the value that you can get from it.
Richie Cotton: So, that first idea around making sure your data is tidy is one of those things that's easier said than done. So do you have any tips for how you can go about tidier or easier to analyze as a small business owner?
Kendra Vant: I think the universal answer to everything, is that where you put your attention is paramount. If you would like to be able to derive value from anything, including data, you need to pay attention to it when you are generating it. So if you are a small business owner saying, I'm sure there'd be something in there, you sort of need to begin with the end in mind.
If we go for a, a well known phrase, what I mean by that is you need to label it correctly. You need to categorize it consistently. You need to, as you go through your general ledger categorization, be consistent in the saying, this is office supplies, or this is, and that sounds boring, and it sounds mundane, but it's actually, as, everyone listening here will know, it's the bedrock of being able to do anything useful.
Because what are you trying to do when you look at data? You're either probably trying to eyeball it yourself for patterns, You can't find patterns if you haven't added them, or you're trying to feed the data to a machine. And as we all know, machines are really, really good at picking up whatever is actually there, and if what is there is noise.
They will pick up the noise. So I would suggest that paying attention to making sure that you are generating the data and the labels around the data, i. e. the categories and the annotations that you put in a consistent fashion, will pay dividends in allowing you to then pull information out of that data.
Richie Cotton: I like the idea that I'm just doing really simple things like Categorizing your data consistently is going to pay off in the future. So something that might seem boring is really going to benefit your business. I think as you mentioned, a lot of people when they go into business, they don't necessarily care about data.
They might be a bit hesitant about using data. So if I'm a plumber or a cook or something, then I'm not necessarily going to think, Oh, data is something I should be spending my time on. What data skills do small business owners need?
Kendra Vant: think the first one everyone needs is just a little bit more confidence. you can get that by sitting down and working with your bookkeeper. So I think... Accounting is a really interesting space because some of our primary users are actually folks who are really number savvy. Accountants and bookkeepers are actually really numerically literate, number savvy people.
Now, they might have some concerns around the technology side of things, but if they, they are really good at dealing with data because they have done it all their lives. So one thing I'd say to small business owners is that advisor you have. that bookkeeper that you work with, that accountant who looks after your taxes at the end of the year.
If you approach it with curiosity, they're actually a fantastic resource to helping you become more comfortable with data. And they'd probably be quite delighted if you asked them, because you know, when you do poor coding on the general ledger side of your input, they're the ones who sort that out and make sure that actually at tax time, everything flows through really smoothly.
So that would be one suggestion would be lean on the business partners that you have today. And then the other one. would be to put down your preconceptions about the fact that it is difficult or the fact that you need specific skill to do it. And there are lots of great online resources if you want to read about it.
But essentially, yeah, approach it with a child's mind and say, I believe I can learn about this information start from there. One bug there for me is I think our education systems universally across the world kind of turn people off. Doing quantitative things. We don't, unfortunately, teach math particularly well.
We teach statistics. If possible, even worse. And people tend to leave formal education with a misconception about the fact that it is A, hard, B, complicated, and C, not for them. And if you can put down A, B, and C and start again and say, well hang on. everything I read in the papers says that machines are taking over the world, that data literacy is going to become more important.
How about I give it another crack and try again with my newly enhanced googling or chat GPT skills? Give it another go would actually be my biggest recommendation.
Richie Cotton: That's fantastic because it is so easy. Just. Say, Oh, this isn't for me. This is a hard thing and give up before you even tried anything, I think I do like that idea that you just. Got to give it a go and see so trying to be more concrete about this. I mean, so we talk a lot about the idea of data driven decision making that is a small business owner.
You're gonna have to make a lot of decisions yourself. So how can you bring data into that process of making decisions about your business?
Kendra Vant: So I can't escape plug for our products. So that is what our products do. So let me give you two examples which you certainly could do yourself or if you are a user of zero, you can make use of the products that we offer. So one of them is forecasting your cash flow. So A very, very, very common reason that small businesses go out of business is because of cash flow problems.
And I don't know what it's like in every jurisdiction that we operate in, but certainly in Australia we have challenges with big businesses paying small businesses slowly. So it's really common to sit on a float. And be like, Oh, you know what? I'll just pay my invoices a little late. I'll keep that money in the bank and I'll use that as a float to run my own business.
And Big Business is engaged in that practice, unfortunately. So cashflow or the lack thereof, people not paying invoices on time is a huge reason that small businesses. Go out of business, or can't make payroll. So what is the first thing that you can do to assist a business with that? Apart from trying to influence government policy to make big businesses pay on time.
What you can do is give people information about payments. So we have built and continue to extend and work out a cash flow forecasting app, which looks at the sequence of payments into your accounts over the last 30, 60, 90 days. And forecast forward what your level of cash flow intend to be. Now, I know that people can and do do this themselves.
I know that because I was at a cocktail party on a frigate. True story. And was talking about the fact that I worked at Xero. And one of the gentlemen there who was a port designer said to me, Oh, short term cash flow forecasting. He said, I used to do that myself in a spreadsheet. And now I use your app, which I was delighted, but so you can do it yourself.
But what it effectively says is in 90 days, given the pattern of transactions and the pattern of payment coming into your account, this is the cash flow balance we expect to see in your account. And that's transformative because it allows you to say, I can't make payroll unless I dig in and understand that one supplier who has a habit of paying 14 days beyond the due date.
Okay. That's an action that I can take. I can bring that supplier and say, mate, I need you to pay me on time. I don't set my payment terms, for amusement value. I set my payment terms so that I can make cashflow and payroll and pay my teams. So cashflow forecasting is one great way to use data to inform how you run your business.
Another one that. I know is just so valuable to the small business economy. So again, I'm going to, talk through it is at the other end when we are pulling information out of everyone's bank accounts and into the general ledger, there is an incredibly tedious process that all bookkeepers and small business owners need to do, which is called coding your transactions to the general ledger.
And that's where we're talking about before providing high quality data. It's saying. Oh, yeah. That was when I took client X out for the coffee. Oh, oh yeah. That's when I had to make the emergency run. Cause I'd actually run out of envelopes and I went into the office works and purchase some things and putting those against the correct categories.
Now, again, that's the place where you as a human, if you are tidy and organized Consistent in your coding can speed yourself up, but it's also where machines can do a great job because that's where you can source the wisdom of crowds. You, as a small business owner, intimately understand your own purchasing habits.
We, as a SaaS software provider, don't, but we do have the benefit of millions of customers transaction habits and hundreds of thousands of bookkeepers categorization habits. So we can, and have, and it is now available in our software, create. Machine learning driven predictions of that category that just, that's right, that transaction line that just flowed through from your bank account.
We believe it belongs here. Would you like to accept that? Yes, and an amazing toil reduction for small business owners because it is a lot quicker to review what a machine has suggested to you, you might want to accept. Accept it 90% of the time and go, oh, silly machine, you got it wrong 10% of the time.
Then to actually have to access your own memory every single transaction and click all the buttons that allow you to categorize. So those would be two places I think you can use data to speed up your day to day.
Richie Cotton: So you mentioned that zero, you have millions of small businesses and you can see patterns across the data that an individual small business couldn't see. Can you give me an example of some of these patterns that appear
Kendra Vant: Yeah, this is actually another really, really interesting one because it's actually quite tricky to build. But when we released it. Folks were just in. So we have a product in a number of our, the number of countries we work in called Hubdoc. And Hubdoc allows people to upload their financial documents and to have those financial documents automatically processed and added to their accounting software.
And we have for a. Machine learning driven pathway so that when you upload your financial document, be that a bill, an invoice it goes through OCR, Optical Character Recognition, it comes out as an electronic version of itself, and we then go through and pick out the key fields. So we can pick out what currency is that in, what date was it, who was the merchant, and what is the invoice total, for instance, let's say.
Now, one of the most annoying things, and I'm not an accountant, so people will have to forgive me if I get this slightly wrong, but I'm fairly sure I am correct, is when what you think is an invoice comes through. But it is actually a credit note. So rather than somebody charging you money, they're actually giving you money back because you've overpaid a previous invoice or something like that.
Now as you can imagine, if you have a document come through and you code it as an invoice, when it's actually a credit note. In your backend, you actually end up with a double up problem because it was money that was supposed to and it goes up on the other. So say it was 25, 000, you'll actually get a 50, 000 offset of incorrectness and where the money is supposed to be because you took negative 25, 000 and put in a positive 25, 000, 50, 000.
So it is the bugbear of small business owners, accountants, and bookkeepers everywhere when somebody miscodes a credit note because you have to go picking through. Reconciled General Ledger, understanding how on earth you have a 50, 000 incorrect level in your bank account versus your Reconciled Ledger.
Now, what we are able to do by looking at millions and millions and millions of financial documents was build something called, okay, this is, our great naming here. Credit note detection. But it does exactly what it sounds like it does. It's not a simple problem as your listeners will understand because it's anomaly detection.
And anomaly detection is hard because for every, credit note there are, I'm going to get this slightly wrong, but hundreds if not thousands of invoices. So the documents look very, very similar. Some people will stamp credit notes across them and other people won't. They look very, very similar.
And one percent ish or a fraction of a percent ish are actually credit notes. So it's a hard thing to do well. It's also actually a hard thing for humans to pick up because we're really, really good at getting into the mode and just going, yep, click, click, click. But that is an example of where we can use the transactions of millions of people to pick up the subtle patterns that indicate this isn't an invoice, it's a credit note and actually alert humans in the flow.
So that's something I really like too is actually the way we do it. It's very easy for humans to fall into the mode of I'm just clicking a button. And we need to be reminded or you probably need to pay a little more attention before you click this button. And it's one of my sort of hobby horses is I get frustrated when I hear people think that an AI product is the algorithm.
It's the sexy part. It's the part that gets talked about. It's the part that gets published. It's the part that gives people patents. But it's only part of a really functional AI product. And when I'm talking to folk about how to build really functional AI products, I'm always reminding them that it's a systems problem.
And it's the end to end flow including the human and in this case a credit note detection the piece of the flow That actually we thought quite hard about which is this is an impactful thing because if we get it wrong It's really annoying and if the human doesn't notice It's really annoying and ends up in the wrong place.
So we actually subtly, but in a way that, to a human stands out because it is different, redesigned the interface at the point where we say, Hey, human, we're fairly sure that this is in fact not an invoice, a credit note. But we suggest that you now pause and take a little time to think. Our decision.
And so that just that subtlety and redesign the interface and making that pop up to the person in a way that is noticeable was a really important part of building a really useful AI product. And we rolled credit note detection out. Gosh, time flies. So, it's probably longer ago than I think it is now, several months ago.
And kid you not, people love it to pieces. It was one of the most requested product features, and when we rolled it out, people were in raptures that we have now, an anomaly detection system that allows them to avoid accidentally coding a credit note as an invoice.
Richie Cotton: that's fascinating. And I love the point that the AI is just a tool and actually the thing you're trying to improve is the customer experience. So you really have to think about the user interface and things like that. So, do you have any advice on how things like the design and product teams can work with AI and understand the nuances here?
Because sometimes you have. AI and data teams separately from the, the product teams and maybe the communication between them can be quite tricky. it seems like you did a lot of wrangling of the, these different teams. So, I was curious as to your advice here.
Kendra Vant: Yeah, it's a really good question, and I have really strong advice. Don't. Don't have separate algorithm and product teams. It is insane. It adds way too much overhead, and you'll end up building things that each side of that equation thinks are good. And your customers think I'm not so good if we are like with our language an AI product, which to my mind, a hundred percent is the end to end complete customer experience, including the human computer interface is no different from any other product.
You wouldn't. arbitrarily split up another product and say, you know what, you do the button and you do this bit, you do that bit. In my lived experience, AI products are harder to build. They're harder to build because we're not as used to them, because they are complex and subtle, and because you are hard coding in a way that is almost impossible to really pull apart.
You are deeply wiring together. A flow and large amount of data that your algorithm was trained on. you know, a long time ago Google wrote a paper called the High Interest Credit Card of Technical Debt, machine learning, the High Interest Credit Card of Technical Debt. And it's because of that absolutely insoluble, tight coupling.
Between the data that your algorithm was trained on and the end to end flow that your AI product has. They're harder to build, they're more expensive to maintain. You want to start off with a team setup that is as optimized for that as possible. So just don't separate them, in my opinion, if you want to build something successful.
The other thing I would say is... This is tricky because in my experience, AI product build is a lot more iterative than other types of product build. I've yet to find a team who can sit down at the beginning of a challenge and say, Yep, I can absolutely guarantee for you that with the data available to us today, we will be able to build a product that is acceptable to the human users within three months.
Maybe there are teams out there who can do it, who are iterating on a product that they have already built, but I think it's incredibly unusual. So you need to allow for more iteration time. what I would say though is, perhaps there is not full time work for a designer throughout that entire process, but Wobetide, the team that thinks they don't need a designer, involve right at the beginning of the conversation.
So get your cross functional skilled team together at the beginning of the conversation. Get them to know each other, get them to appreciate the other person's skills. Don't skip that step. Don't skip the step that says at the end, we need to have an end to end usable product that humans don't have an uncanny valley reaction to, that doesn't trip them up in their workflow, that doesn't provide something that is so subtle that nobody ever noticed it happened.
They're expensive to build. They're slow to build. We sure as heck don't want to get 95% of the way there. And then discover that we have an unworkable human computer interaction. So, take my advice, get those folk involved right at the very beginning.
Richie Cotton: That seems like great advice to get all the people you need together at the start. And I think you, you mentioned that almost no one will tell you that they can do this sort of thing in one go. Probably the people who do tell you that they can do it in one go, they're, they're people that you don't want working on this sort of project, I think.
Kendra Vant: There are almost certainly folk who simply haven't done it before. Or what I see disconcertingly too much of now, and it'll ebb and flow, because we're humans and we'll come and, we get over things. But there has been, at least in my head, at least twice, two waves of this, and I think we're in another wave right now, is very enthusiastic software engineers.
Who believe that as long as they can call an API, they understand how to do machine learning. And we've all gotten very excited in the last few months about the amazing accessibility of really competent large language models. And, oh my goodness, the number of people I have heard Say to me, oh, we can just call the API.
Can you now? Good luck with that. Well, the beautiful Forbes type article that was circulating with all the boards in Australia, and I'm sure all the boards in the U. S. as well. The cost of software engineering is going to go to zero in the next 10 years. Is it now? Software engineering is only writing code, is it?
Is that right? So I would say, yeah, absolutely. Folk who, who tell you that this is going to be very quick now, because all I'm going to need to do is stitch together multiple calls to chat GPT using LangChain, have never actually tried to build a functional AI product. So avoid them, bite the plague. Or if you really need their skillset.
Embrace their enthusiasm, but make sure that they work in lockstep with folks scientific training who understand how to build experiments and test the outcomes of those experiments and user interface experts and product managers who really understand what the customer is after because it's very easy to think you are building for the problem that you're trying to solve, but actually build to solve a subtly But importantly different one.
I
Richie Cotton: And I'm sure we've got a few chief finance officers listening, thinking, Oh yeah, cost of software engineering, go down to zero. That sounds brilliant, but it might be might be wishful thinking on a related note. So, you said that these sort of new generative AI tools, the large language models well, they're difficult to build products for, but are they useful to small businesses?
Kendra Vant: think at the moment what we see is that they are difficult to replace humans with and you have to think really carefully about doing that. They certainly seem to be very, very attractive for augmenting humans with. So I'll just give you a couple of the for instances that I've heard from small business owners.
One of them is writing boilerplate or what I call breaking the white piece of paper problem. Who hasn't sat down in front of a proposal they need to write or a letter they need to send and gone, I'll know it when I see it, but how does that go again? Particularly, you haven't had your coffee on a Monday morning.
I know a large number of small business owners who are It's their go to. They have it open next to Google, I'm sorry, I'm talking to chat GPT, the interface, OpenAI's interface, they have it open next to Google and they use it to generate the first draft of communications. And I do stress the first draft.
Folk got extremely excited about it back in the before times, January, February. And thought that it was going to be a drag and drop replacement for a lot of the things they did. The impression I get talking to folk now is. It's like having an intern that, they'll get it to 90%, but you're always going to need to take that extra 10% yourself.
So where I see small business owners using it is largely in doing desk research. If some folk just really prefer the back and forth that you get from a chat bot than that you get from a search engine. Creating first drafts, boilerplates, break the white sheet of paper for written customer communication.
Now, I would say that they're also pretty careful to note now. Perhaps they weren't four months ago, but they are now. Yeah, but you do want to read it before you use it. Because it will get it 90% of the way there, perhaps even 95% of the way there. But as we're seeing play out in the courts now, as the human who uses it, you're the one that's responsible for it.
So that would be my, just my note of caution to some small business owners is, it's still you, it's still your business. And I would always read very carefully anything that came out of a generative large language model, because it's not sentient. It does not know what it is doing. It cannot give you a confidence level for how confident it is that what it has said is the right answer to your question.
It is a sequence generating machine, and it is generating you a statistically likely sequence. To the sequence that you put into it. So since you were mentioning plumbing business earlier, then we're on boiler plate now, I think AI is great for writing boilerplate, not good for fixing boilers.
at the moment, certainly true.
Richie Cotton: That would be a good invention though. AI that can fix your boiler. All right. So, getting back to using data and small businesses, are there any important business metrics that you think are important as a small business?
Kendra Vant: I am not an accountant, so I should put a very, a very large warning of amateur opinion. It really depends on your business, Richie. And this is something that I would simply say, having had conversations with many, many, many small business owners the ones that they all come back to is cashflow, incredibly important cost of goods sold.
Incredibly important. There's a lot of subtleties around payroll and understanding particularly awards rates. So, extremely important to understand your obligations as an employer. And that's something that, again, a lot of small business owners don't go into business to be employers. But then as their business thrives and they become really successful they suddenly discover that there are lots of people relying on them.
So having a really careful understanding of making sure that you are doing everything that you need to as an employer is probably another place where I'd say paying really close and careful attention to that data is really important.
Richie Cotton: Okay. So it does seem like, a lot of this really is around like, the finances of things and also around the compliance stuff like tax and regulations and making sure you're, you're doing the right thing there.
Kendra Vant: So I think it's a case of get the basics right, right? So I think one of the reasons that small business is an extremely difficult area to, if you think about companies like CERO, like financial institutions I've worked in financial institutions for many, many years, not recently, but for many, many years, and small business is really, really hard.
Anyone who works in a large bank will tell you, yes, it's really hard. What is the reason for that? The reason is because small businesses are incredibly varied. Like, as much as we all as humans would like to think we're unique, we're kind of not. Like, consumer patterns, consumer patterns are reasonably easy to tap into and reasonably easy to fingerprint.
We spend fairly similarly. It is not difficult for a bank that has a significant amount of your banking to figure out quite a lot about your spending habits and to figure out quite a lot about your household composition from the spending habits. There are just Two things. One, although there are an enormous number of small businesses, there are a lot less small businesses than there are spending households.
So we have a small data problem, and within that somewhat smaller pool of small businesses, there is a far greater dynamic versatility of the types of small businesses that you have. that's why I think that it's, difficult to say this is the killer app for a specific vertical. Because there are, there are killer applications for data for all the different specific verticals of small business, but they are myriad.
So what's the common thing that all small businesses have in common so that it's easy for, in a general podcast or a general conversation to zero in on? It is the thing that everybody has to do. Everybody has to make payroll. Everybody has to pay their taxes. Everybody has to understand that they have paid their bills, that their suppliers are paying their bills.
So there's a reason, I think, beyond the fact that I work for an accounting company, there's a reason for the fact that we all come back to the lifeblood of small business data being around finances, because it is the common thread that all small businesses have to do well. Then, of course, depending on whether you are an inventory based small business, a services based small business, or any other of those myriad different ones, there will be pockets within each of those verticals where the data is incredibly rich and you can do a lot of other things with it.
Yeah,
Richie Cotton: I find it fascinating. The idea that small businesses are incredibly varied and maybe more so than, than large businesses, perhaps. Given that you've got millions of customers, like what happens if you do patent recognition on this? How do you, if you do a cluster analysis on small businesses, what are the groups there?
Kendra Vant: it's not so much that small businesses are more diverse than big businesses, it's that you, like if you're thinking about a bank, a bank can afford to employ a human to look after the accounts of a small business. That's worthwhile. You can't afford to employ a human to look after the accounts of one small business if you're a bank.
So that's why you need data to do more for you. But it's extremely diverse. What you're talking about, I think, leads inevitably to the holy grail of accounting, which is small business benchmarking. Lots of people work on small business benchmarking. We're certainly very interested in it. let me talk about one piece of it, perhaps.
One thing that, when you, you have to start thinking about quite hard when you start getting into this is What's a similarity metric? How do you come up with a robust similarity metric to describe whether Richie's small business is similar to Kendra's small business? And therefore, if we understand that they are similar, how can we say, Oh, well, Richie's small business appears to be more profitable, even though it is similar to Kendra's, therefore, how can we tap into what he is doing better than she is?
There's an enormous amount of data cleanup to be done. If we go back to the conversation we were having at the very beginning of the hour when you were trying to get into something as esoteric as similarity measures in something as non standard and multi dimensional as small businesses, there is an enormous amount of work to be done to define, on what dimensions of similarity are we interested in?
is there a simple answer to your question? No, there is no simple answer to your question. Is it an area of great interest to almost everybody in the small business economy? Absolutely there is. And it's actually where perhaps some of the most fascinating data challenges and machine learning challenges go on.
Because you do get into some fairly fundamental concepts. About we probably want to adopt something fairly similar to the concept of vector embeddings, but now instead of vector embeddings into a phase space of the English language. You're talking about vector embeddings into the phase space of a business similarity dimensional universe.
What does that even look like? It is a really complicated and honestly really live question that a lot of people are pursuing. Even when we get there, I think the answer will still be just as the multidimensional phase space of the English language is incredibly sparse in patches, You'll find the same thing, I think, in small businesses that will clusters emerge.
Yes, clusters will emerge. They will be associated with business verticals, but there'll be
an enormous amount of subtlety between those things You know a lawn mowing business perhaps running out of Queensland, Australia and a lawn mowing business running out of, Surrey. So yeah, I think enormous amounts to be learned there. People very, very busy doing so. Probably one of the most challenging questions to answer.
In a practical way, could an AI products team amuse itself in that space for a very long time? Yes, I'm sure they could. Can we give a definite guarantee that in six months time we can build a really tangible product? Much trickier, because small business owners and their accountants can't agree on what dimensions is important to benchmark small businesses.
And when humans don't agree, you're going to have a really interesting and challenging time building an AI system can provide an answer because two sets of humans will say, I don't like your AI system because it doesn't, it doesn't align with what I believe the most important similarity metrics
Richie Cotton: That's a really great answer. I seem to have stumbled into a very deep research problem. Perhaps this is something our listeners can spend some time thinking about. It sounds like there's space for some incredible research wins there, maybe even some business wins if you can figure this sort of stuff out.
I want to go back to a point you made earlier about, well, sometimes small businesses thrive and with a growing roster of staff, what's the point in a small business lifecycle where you say, okay, now we can start employing some data professionals ourselves.
Kendra Vant: are. Only a very small sub segment of small businesses will ever find that useful, right? You can run an incredibly productive small business doing graphic design. You probably never find a place in which it's useful to to employ data professionals.
So let me just touch on a couple of spaces where I know that people have done so and it is useful.
I think one would be the sort of the high volume dropshipping type applications, so people who run thriving businesses which are fairly low staff where they are fulfilling online orders. So I think that those spaces you can get to quite high revenue generation, perhaps not profit because it's goods in goods out, but quite high revenue generation with fairly large volumes of sales rolling through and those are spaces where I think that I know some, small but successful business in that space have found it useful to bring in folk who can look for the patterns in that because yeah.
Those, a lot of those businesses are volume gains. so they lend themselves to what data can do really well at scale, which is shave percentage points off of cost and therefore, just streamline that. So that's one where I'd say is, I think that there's certainly those sorts of high volume sales businesses can make use of, can find it valuable to have people maybe not on permanent staff, maybe on contract to do patent analysis for them of their sales.
I mean, that's the one that springs to mind. there are certainly lots of verticals in the small business industry where this is important. And I, I know that because there are now more than a thousand apps in the Xero app store. So, why do I mention that? Well, from the very beginning of Xero's business our founder knew that the small business economy is incredibly varied.
And one company trying to build to the needs of all of those people is inevitably going to fail badly because we can't possibly spread ourselves that thin. And so, we have the Xero marketplace. And. Within that space, there are apps aimed at particular sub segments of the small business community. And I think that is where you will see the really data rich areas, is where you see the clusters of apps coming up with particular problems.
So, one that's just quite close to my mind, to my heart personally, is the needs of the agri tech. So I have hobby farm and it's the hobby farms where I hope to retire to one day in beautiful regional Victoria. And so I'm really, really interested in how you do broadacre farming in small scale and how you run cows and how you become anything other than a grass farmer.
And. I really love talking to the agronomists who work with the farming communities in Australia. They mine the data within Xero to do things like help inform their, massive broad acre farming, so their big farming plants. Fertilizer yields. So, they, they mine the information of how much you're spending on nitrogen based fertilizer, how much you're spending on extra stock feed, how much you're spending on herbicide.
They add that data to the information of when you are applying it, where you are applying it, at what levels you are applying it. they do an enormous amount of add on work in understanding crop yields and stock yields and all the rest of it, and then advising multi million dollar.
Family businesses on how to make their farming operations more effective. So there's another place Where data professionals certainly have a place to play. Now, those are reproducible enough that there are actually businesses within Science Zero's App Store that specifically concentrate on providing small businesses with those answers.
So that's a place where maybe it's such a useful, such a replicable need. People have actually said, well, there's a whole business model around doing that, and so I'll hire some of those data scientists, and I'll get them to focus just on the particular problem of how do I better inform farming operations.
So there's just, heaps and heaps and heaps of places within the small business economy where either It makes sense for an individual small business owner to employ one or two people who are savvy and comfortable with data or there's actually an entire business idea around bringing that data together, enriching it with data from different parts of an organization's operations and building a software solution when there's certainly employability for data scientists and data analysts and data professionals of all kinds in those spaces.
Richie Cotton: That's fascinating. I have to say, I'm always amazed at how much data there is involved in farming when it seems
Kendra Vant: Oh, my goodness.
Richie Cotton: such a low tech field, but actually there's so much there. And your other example about logistics and if you're running packages everywhere, then there's going to be a vast amounts of, data involved in that.
But it does seem like, in the small business space, your point is that a lot of the data usage is going to come through software rather than someone just messing about with Python or whatever. It is going to be encapsulated in a platform rather than being just numbers crunched by an individual.
Kendra Vant: And I think that's just a scale thing. There are very few small businesses who can afford to buy. One thing we get a lot chatting with small business owners and accountants is one reason we use SASS platforms is because then we can have Kendra as our chief data officer and Susie, my colleague, as our chief security officer.
Which is effectively true. Right now, as a small business owner, I can't afford Kendra and Susie is my chief data officer and my chief security officer and know what I want to because it's not my core business. But why? One reason I'm comfortable with paying a subscription model for a cloud platform provider.
Is the cause of the expertise I effectively hire through that and small business owners actually think like that quite a lot more. I think than really large people who work in really large businesses do they're like, yeah, I'm effectively buying a tiny fractional CDO and tiny fractional size. So I think that small businesses, huge generality.
There's an amazing number of different kinds of small businesses, but. They are quite plugged into the app ecosystem and that concept. So I think, yeah, if you're interested in the space and you're interested and you're a data professional who wants to work in that space, one way is, of course, finding a thriving small business that is trying to become a not small business.
And therefore might have the space to bring you in as a dedicated professional. But another way is just to look at all the places in the ecosystem of small business where a lot of data flows. And either, if you're entrepreneurial, start your own small business around the small business data flow. Or find someone who also finds that a really fascinating problem and is trying to solve it and get on board.
Richie Cotton: Fantastic. I like the idea of starting a small business, help small businesses, just small businesses all the way down. Before we wrap up is there anything you're working on at the moment that you're excited about?
Kendra Vant: I think one thing I have perennially been excited about and it's only growing at the moment with the, hype cycle, shall we say, of ChatGPT is data literacy. So, I'm the mother of three children who've finished through the education system, are off in tertiary education now, and I want them to have long and productive careers.
And I want that for everyone who's listening to this podcast. I think that it is true now, and it will only become more true over the next 50 years, that being comfortable. and confident with data, with what it means, with how we treat it well, being able to spot when someone is lying with statistics, being able to understand how to use statistics to understand more about it, is only going to become a more important part of the working life of Every person listening to this podcast.
So that's a big, big thing for me. I'm really excited about helping people have confidence to do things. making use of all of the tools that are emerging and, and maybe using this. tipping point with TPT and large, large language models becoming part of vernacular to reinvigorate your confidence, reinvigorate your thirst for learning and say, I've got 40 years working ahead of me, 50 years, 60 years, we're all going to be working for a real long time, right?
Because if we're all going to live to 100, we're all going to be working until our 80s. how do I build into my sort of my patterns, my habits, a thirst for learning? Okay. I thirst for re educating myself and reinvigorating my career prospects. And how do I reach out and use those incredible online resources to become a more confident user of data?
I'm passionate about that because I think it really will assist millions of people to become confident literate. And economically productive and I'm also excited about it because it feels like now is a time when people are being exposed to a much greater extent than they have been. And so it's a good time to make that mind shift and to say, okay, I'm going to jump on this bandwagon and I'm really going to learn.
So that would be an area that I'm really passionate
Richie Cotton: Fantastic. I like the idea of just having this thirst for learning because you, you can have a long career. That's brilliant. I think it's a great note to end on. So thank you very much, Kendra.
Kendra Vant: and excited. Thank you, Richie. It's been a real pleasure.
blog
Top 32 AWS Interview Questions and Answers For 2024
blog
Top 5 Business Intelligence Courses to Take on DataCamp
DataCamp Team
3 min
podcast
Avoiding Burnout for Data Professionals with Jen Fisher, Human Sustainability Leader at Deloitte
podcast
Becoming Remarkable with Guy Kawasaki, Author and Chief Evangelist at Canva
tutorial
How to Create a Waterfall Chart in Tableau
Eugenia Anello
8 min
tutorial