Why AI will Change Everything—with Former Snowflake CEO, Bob Muglia
Bob Muglia is a data technology investor and business executive, former CEO of Snowflake, and past president of Microsoft's Server and Tools Division. As a leader, Bob focuses on how innovation and ethical values can merge to shape the data economy's future in the era of AI. He serves as a board director for emerging companies which seek to maximize the power of data to help solve some of the world's most challenging problems.
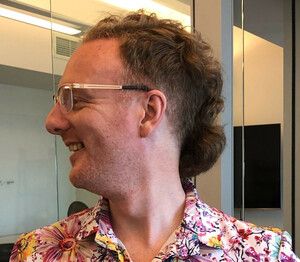
Richie helps individuals and organizations get better at using data and AI. He's been a data scientist since before it was called data science, and has written two books and created many DataCamp courses on the subject. He is a host of the DataFramed podcast, and runs DataCamp's webinar program.
Key Quotes
With the advancements we're seeing in artificial intelligence, I've come to realize that there is a very high probability that I will see artificial general intelligence and be able to make use of it in my lifetime. And I think it's going to happen within 10 years.
Everyone should be looking at data lakes and having people play with them. Playing with it in the sense of trying to find solutions. One of the really fascinating things about this technology, and it cannot be understated, is the importance of the large language model artificial intelligence technology that's emerged over the last 12 to 18 months. For the first time. You know, we have intelligence in a computer program. We've never had that before. Independent intelligence, intelligence that's independent of human intelligence. I mean, humans could use intelligence and build a program and programmatically the machine would follow that very rigorously, would always do a very good job of that. But the intelligence always came from the person. Now the intelligence can come from these models, these large language models, it's an incredible step forward because it allows you to put some glue essentially into every application to make that application more effective. And almost anybody can take and prototype something, if you have some technical capability you can prototype something with GPT-4 and Langchain and a few other tools that you have and hook something up. And pretty quickly like in a week or so, come up with a demo that is interesting and potentially compelling for a given company. What's encouraging is that the tools for enterprises to do this are going to emerge in the next 3 to 12 months. It's going to be much more straightforward because of these modern data platforms like a Snowflake or Databricks or Microsoft or Google. They're all going to make it a lot easier to build these tools. And we saw a lot of that yesterday in the announcement Snowflake did. A good part of what they announced at their summit this summer [Snowflake Summit] were tools to simplify the incorporation of AI into applications for companies.
Key Takeaways
Data governance is crucial in the age of AI. Companies should ensure they have clear processes about what data is public, what data is private, and how to handle each, especially when fine-tuning AI models.
Artificial General Intelligence (AGI) is advancing at a rapid pace and is likely to be a reality within the next decade. Professionals in the data space should prepare for its impact on various sectors, including medicine, business processes, and scientific discovery.
The pace of innovation in the data space is accelerating, with new models and tools being introduced frequently. Continuous learning and adaptability are crucial for data professionals to stay relevant such as the use of data lakes for AI projects.
Transcript
Richie Cotton: Welcome to DataFramed. This is Richie, and today I'm talking to a data legend. Bob Muglia was Microsoft's first technical hire for the SQL Server team back in 1988, and over the two decades he spent at that company, he ran a lot of teams building data and business software. Including Office and Net and Azure.
In 2014, he became the CEO of Snowflake where he helped to define the vision of the modern data stack and guided the company into becoming the data warehousing giant. It's today. Bob has just written a book called The Datapreneurs. The first half is a retrospective, celebrating the entrepreneurial spirit in the world of data that he encountered throughout his career, and the second half turns to his new passion, artificial intelligence.
It's a great mix of practical advice and insights about the possible future of AI. That means we've got a lot to talk about. I'm really excited to pick Bob's brain and hear his thoughts on everything from data warehousing to the future of ai.
Hi Bob. welcome to the show. Really great to have you here.
Bob Muglia: It's great to be here, Richie.
Richie Cotton: I'd like to dive in and talk a little bit about your book The Datapreneurs. So, first of all, can you tell me what is a Datapreneur?
Bob Muglia: Well Datapreneur is shortened for Data Entrepreneur and the name came from when I was working with my co-author, Steve Ham, and started writing a narrative of the book on the early, in the early days. We weren't ... See more
And Steve had mentioned that he felt like there was a story in here for a book. and after thinking about it a bit, I said, you know, Steve, I'd love to do this about the people I make the story about the people I've worked with, the data entrepreneurs throughout my career, cuz I've been fortunate to work with some amazing people.
And immediately he shortened it to the, you mean the data entrepreneurs? And I thought that's a good name. So that's where it came from.
Richie Cotton: Nice. And can you tell me why you think this sort of idea of entrepreneurialism is important in the world of date?
Bob Muglia: I think creating things is important. I think learning is important. I feel like we're on this earth to understand everything about the universe and understand the world around us. And to do that we need to create new things. cuz you need the tools, we need tools to learn more.
And that really comes from people who are entrepreneurial and the realization I had, and when I was writing this book, which was actually something that I had, I didn't realize. For all those years I was working at Microsoft was that even though I spent over 20 years at Microsoft, a very large technology company, they're actually a lot of entrepreneurs at Microsoft and I was fortunate to work with a lot of them.
I wound up in my career moving around in the product teams at Microsoft a lot. I tended to go into an area that was having problems or a new area for the company. Spend a couple of years in there, get it started, and then move on to a different area of the company. And so I was fortunate to, to work with a lot of different people doing entrepreneurial work, particularly during the 1990s when Microsoft was doing so many things to lead the computer industry.
Richie Cotton: It does seem like a fascinating time of Microsoft in the 1990s, and in fact your book opens with this sort of vision from B Bill Gates back in 1990 about information at your fingertips. So, maybe you can tell me a bit about what that was all about.
Bob Muglia: Yeah, in 1990 at a industry conference, such is long gone, but used to be the cat's meow called Comdex in Las Vegas in the fall. Used to be the big show every year in the late eighties and early nineties. Bill gave a talk that was a pretty milestone a speech that he gave in 1990 where he described a vision of being able to get access to any information that you wanted from your desktop, and you have to put yourself in the perspective of 1990 and where things were.
Most people did not have email. Email was, unusual. There was no internet back then. People were doing things on pencil and paper for much of what business was happening. Large companies had computers but people still worked, with pencil and paper and a lot of things.
And so, PCs were really just growing back then. And this vision was one where people would get access to any information that they needed to do their job. And while the approach that Microsoft took for on it, which was a very PC centric, very window centric approach, was unsuccessful, the vision I think was incredibly successful the idea that we could get access to information, what really fulfilled the vision ultimately was the internet.
And tools like Google, which have allowed us to have access to incredible amounts of information. And of course, people have access now to all sorts of information within their companies. and it just, more and more data keeps coming at us. I.
Richie Cotton: The idea that information isn't successful at your fingertips now just seems absolutely
Bob Muglia: It does, doesn't it?
Richie Cotton: really was.
Bob Muglia: it's, and it's, it's really, and you know what's fascinating is the thing that's interesting, we'll talk more about this in the podcast is that what's happening now is we're moving from just being able to find this information to having these, bots answer things for us, answer questions for us.
I find myself more and more just asking questions. Of AI to find an answer to a question I have, and it's the easiest and most convenient way to do it.
Richie Cotton: Absolutely. And I would love to talk more about bots and AI in a moment, but I think there's still some fascinating stories you have from your time at Microsoft that I'd love to sort of hear a bit more about. So you have a great story about when you were working on SQL Server and you've installed a data center in your house so you could test the software.
Can you just tell me a bit more about that?
Bob Muglia: Yeah, in the early two thousands, I think it was about 2002, 2003, I was working on a house, here in the Seattle area. And I was running at the time the Windows server team and our management group and, a number of different server products. And the thing was, That we were really targeting.
One of the things that was Microsoft did a really good job of was building software that could be used by smaller companies and bringing business systems and databases and general business technology into companies of all sizes. Cuz again, in the early nineties, most small business ran on pencil and paper and that all changed with PCs and mostly was Microsoft software and then a set of applications that were built by third party companies.
On top of That software to allow it to happen. And we had built a product that was targeted at small businesses. It was called Small Business Server, and it put everything together on one server and it worked pretty darn well. But mid-size businesses, if what you were trying to do was bigger than that one server could do.
You were pretty much on your own. And so I decided to set up what was essentially a mid-size business, in a data center, in my house. It's actually just below me in where I'm sitting right now, and at one point I had 11 Windows servers running in that environment.
And it was very complicated to keep it running. But that was the idea. The idea was to understand, What it took to set up this environment and work with it. And so I was having people coming out all the time, fixing problems and things, and I would be in project reviews with the people that were running these teams.
And sometimes I would know more about their software than they would because I just spent the weekend trying to install the darn thing. and I knew what the problems, were associated with that. And so it was very beneficial. It was a very beneficial hands-on experience. And in general it speaks to the attitude that I think is appropriate for people when you run organizations.
Which is to understand what's going on as best as possible and can get your hands dirty wherever you can. You shouldn't do the jobs, certainly you need to empower people to do the jobs, but you really wanna understand what people are doing. that was a firsthand way to do that in a circumstantial, which is pretty hard otherwise who thinks, normally people don't run this software.
You have to be specialized IT people to run this software. So I just decided to put on an IT hat on weekends.
Richie Cotton: That's pretty amazing. I think there's a great lesson to be learned there for anyone who's involved in developing products that you really have to actually try using them yourself in order to ensure that they work as you expected.
Bob Muglia: We used to call it Microsoft. We called it eating your own dog food. That was what we always called it. And I mean it was an important part of the of what people did in building software, but you know, when you're working on a word processor, everybody can relate to that.
A SQL database, it's not everybody interacts with it directly. Let's say it, let's say that way. So it does certainly,
Richie Cotton: It seems like, that was a very sort of productive time at Microsoft in terms of making data more accessible to people. It's like the big push for Excel. Equal server. Do you have a sense of like what the biggest impacts of your work from that time or from Microsoft's work at that time are on the data world?
Bob Muglia: I mean, I really do believe that Microsoft democratized data and business computing for the masses. If you look historically, business systems were big, expensive, they were the. space of very large companies. And what Microsoft did was it took that technology and it made it accessible to everybody.
And I'm very proud of the work we did back then because essentially we automated business and we did that for literally millions of companies around the world. We were able to get businesses automated because of the software that we built. And, we may not have created the end application that they ran.
If you're a dentist office, you run a dental application. But it was probably running on small business server inside that dentist office, in the late nineties, early two thousands. And so we were providing the infrastructure that made that possible. And I would, and to me that was a pretty amazing thing.
windows server really was the first server system that brought that level of business computing out to a broad audience. Now we have the cloud and the cloud. Is it even more of a democratizing that force because it's, it makes access even easier. It used to be you had to set up ha bring in special people with white gloves and cranes and stuff to set up the mini computer.
We made it so you could just set it up on a little pc in the closet. And now of course it's even easier. You just, sign up for service.
Richie Cotton: It was a pretty incredible innovation bank at the time, and I like that you've mentioned that. Now everything's done in the cloud, which is essential part of the modern data stack. And of course you were. In building that in your time at Snowflake. So, can you talk through first what does the modern data stack consist of?
Bob Muglia: So, the modern data stack, which really didn't exist 10 years ago. I mean, it came into existence. I say the 20 15, 20 16 timeframe, it started taking shape and basically, it was this idea that with the cloud you could run data analytics and you could deliver this data analytics first and foremost as a service.
So that, people didn't have to run it themselves, that it could be run by another company on your behalf, and so you didn't have to understand how to set up the system and all those other things. So it was just delivered as a service. Like an application, but it's a software service.
The second thing that made the modern data stack really different is because it leveraged the cloud. and in particular as the technology was built for the cloud, of which Snowflake was really the first example of that it could scale, the databases could scale way beyond what they could scale before, and they could handle any type of data up until that point.
Databases were very limited in how much data they could store and how many users they could work with simultaneously. So every company wound up breaking up their data into different databases that turned into effectively silos that had to be maintained separately and kept in sync. And it was a mess and it was just a complete mess cuz data was all over the place.
It wasn't the same in this in different places. People were coming up with. Different answers for the same problem because they were looking at different numbers cuz the systems they were working with were different. And it was because there were technical limitations that made that impossible.
And the cloud fixed that by making it possible to essentially work with any type of data. To put data of essentially any size. I mean, literally, petabyte plus sizes of data you could start to work with. And then also it allowed you to put as many users on that data simultaneously.
Remarkably, a system like Snowflake one, instance of Snowflake for a company can support an entire company and there needs to only be one copy of the data. And everyone can see the same thing. And that technology is now pervasive across the modern data stack. And then the third element of it, which is very important, is that SQL databases are used at the center of it and the data is modeled for SQL databases.
In other words, the data is structured in a way that these relational SQL databases of which are quite mature today are able to work with it. And, the SQL databases I think of are the slicer DICs of data. They can chop it up and slice it any different way you want and look at it and aggregate it, put all these kind of functions on it.
And they're just incredible arithmetic machines. And collectively, those, things are core components Now. It's gotten to the point where it's really a very complete solution. the modern data stack where you have ways of getting data in through data pipelines. You have tools to do data transformation to turn it into, the state that you can work with it, the SQL database.
More and more, there's tons of machine learning solutions on top. Obviously BI solutions. Tools like Tableau and Power bi. Really there's just dozens of companies involved in it. And we're now to the point where there are really five distinct modern data stack platforms. Two. Non-cloud providers, snowflake and Databricks, they're sometimes called Super Clouds.
They sit across multiple clouds and provide a consistent experience across the physical clouds. And so Snowflake and Databricks have an offering, but there's also equivalent or roughly equivalent offerings from Microsoft with, they just denounced something called Azure Fabric or Microsoft Fabric which is their modern data stack product.
Amazon has a whole set of products that collectively are, provide a data solution and then Google with their BigQuery line has it. So we've got five different platforms people can choose from. And if you're doing data analytics, you should choose one of them for sure.
Richie Cotton: It's like, yeah just pick one of the five and you're probably gonna be
Bob Muglia: mean, I have an opinion.
I, I'm an advocate of Snowflake. I'll always be a Snowflake advocate. I help to build the platform so it's appropriate that I have that. But I'm very, it's, to me, it's very rewarding. To see all of these platforms pursuing a similar path, and they're all trying to build a similar system now they start in a different place.
Databricks started with the machine learning and Snowflake started with the database, the analytic database or data warehouse. And so what you start with determine somewhat what you build in the end. But the products that all five of these companies are building are actually quite similar.
Richie Cotton: That's interesting that you see that kind of convergence then that machine learning and like the data warehouse need to come together at some point. Do you have any other sort of trends you're seeing around that sort of thing then?
Bob Muglia: Yeah, I mean, I think a couple things have happened. you know, One is that the emergence. Of Data Lakes as a repository for all data has clearly become the approach that large companies are taking. And what's happened in the last couple of years is that there are now some industry standards for how you format data in a data lake to make it accessible, to the modern data stack and do a SQL database.
And so there's two technologies people are using. One is called Iceberg. Which is preferred, which is what Snowflake has chosen as their primary solution. Google has also chosen it, and Amazon I think slightly prefers it, although I do believe they're supporting both. The other solution is called Delta.
It came from Databricks, and it is being supported by both Databricks and Microsoft right now. So you have two different formats. We're in a little bit of a beta versus VHS scenario perhaps on this, cuz we do have two different formats that customers can choose. my intuition says that, Some of these companies are gonna support both formats.
It is unlike bean vhs, you can't build a recorder that supports both. You can't do that. With this, you can, and you can support both, and I think we'll start to see that over the next few years, at least with some of the major cloud providers. Snowflake and Databricks are pretty set. I mean, Databricks is pretty much Delta and Snowflake is pretty much iceberg, but we'll be interested to see what like an Amazon or Microsoft does.
Richie Cotton: Okay. Yeah, I mean, it does seem very calm that you end up with two technologies competing. I mean, I guess, at least a few years ago, it was very much like r or Python and both doing kind of very similar things in the data space and yeah there's tons of examples of these things.
Bob Muglia: And typically one wins. I mean, typically if you look at it historically, one tends to get more traction than the other, but we'll see over time on this one. Like I said, maybe because. You can support both. It won't be that big. It won't be that big of a deal. I mean, the other big thing that, we're seeing right now, the other big trend is injecting machine learning solutions into these data stacks and really the products to make it possible to build complete end-to-end machine learning.
Intelligent or data applications and those, snowflake just had a whole bunch of announcements yesterday. Today Databricks is doing a whole bunch of announcements on this. there's a lot of new technology coming out that's making it easier for people to build solutions that incorporate machine learning, and in particular the new large language models and artificial intelligence into their data stack.
Richie Cotton: The technology is very promising. Do you have any advice for organizations that are wanting to try and modernize? Steady to try and adopt all this new technology.
Bob Muglia: Well, I mean, right now what I would say is that everyone should be looking at it and having people that are. Playing with it. I'll say that. Playing with it in the sense of trying to find solutions. One of the really fascinating things about this technology and, it cannot be understated, the importance of the large language model, artificial intelligence technology that's emerged over the last 12 to 18 months.
For the first time, we have intelligence. In a computer program. We've never had that before. Independent intelligence. Intelligence is independent of human intelligence. I mean, humans could use intelligence and build a program and programmatically the machine would follow that very rigorously, would always do a very good job of that.
But the intelligence always came from the person. Now, the intelligence can come from these. these large language models and foundation models, and it's incredible step forward because it allows you to put some glue essentially into every application to make that application more effective.
And almost anybody can take and prototype. Something, if you have some technical capability, you can prototype something with g PT four and Lang Chain and a few other tools that you haven't hooked something up and pretty quickly, like, you know, a a week or or so come up with a demo that, that is interesting and potentially compelling for a given company.
Now, productizing that. Is a slightly different story and getting it into a format that's useful to your customers and in particular you have confidence that it will not go awry. That it will follow your values and do what you want it to do. That takes a bit more work. But what's encouraging is that, The tools for enterprises to do this are going to emerge in the next three to 12 months.
And right now, I mean, in order to do it, you have to grab your wrench and your screwdriver and your hammer and you put the whole thing together yourself, and you can do it. It's gonna be much more straightforward because of these modern data platforms like a Snowflake or a Databricks, or.
Microsoft or Google, whatever, they're all gonna make it a lot easier to build these tools, and we saw a lot of that yesterday in the announcement. Snowflake did a good part of what they announced at their summit this summer was tools to simplify the incorporation of AI into applications for companies.
Richie Cotton: That's really interesting. I like the idea what you said it's released. Build a demo, but it's very hard to get like a robust product. Actually made evolving ai and it just seemed like there are so many companies just rushing to build AI into their products at moment. Like basically everyone's doing it.
Data come included. And so, can you talk a bit more about how you think it's gonna be made easier to build AI products?
Bob Muglia: Yeah, I mean, first of all, the first question everybody has to ask. Ask right now is what model am I going to use in order to get my intelligence from? And you essentially have two choices. You can go with the commercial models like G T three five or GPT four, which are very powerful. And they're, they have the strongest reasoning capabilities, but they're relatively expensive to run.
I mean, they're costly. And, for some enterprise solutions that may be fine. There are certainly some solutions that are more general purpose, where the economics of that don't work out very well and the alternative is to look in the open source community at smaller models that are not as capable.
But are still quite capable and are much, much less expensive to run. And you'll hear that world is changing at a breathtaking pace. I mean, like every week there's new models being introduced in the open source community that are, have more parameters or there's actually some standardized benchmarks that are being used to score these models in terms of their quality. And, there's no question that something like GPT four as the highest quality. But in just a couple of months we've washed open source models, creep up and get better and better.
And in many cases, they're good enough. And so, incorporating those into a solution is still a bit tricky. you need to be somewhat of a data scientist to do it today, but that's gonna change with these tool sets that are coming from the modern data stack providers.
In the last couple of days, snowflake talked about AI factories, which are essentially a way in which you can do the the fine tuning. You take a pre-trained model that's already been built by somebody else, and then you fine tune it with your customer data to actually understand the characteristics of your particular application.
And then you can apply that, and then you can apply that into a solution. The other technology that is. Very much hand in glove to this, that has emerged as being very important are vector databases. Vector databases are a storage mechanism or a database that can store content, quite often text that has a certain semantic associated with it.
So what you do is you take Text that you have, maybe your product support messages or your Slack internal messages or any corpus of information that is interesting and you run it through a large language model that creates these vectors or these embeddings that are numbers, in a multidimensional space that describe.
The semantics of what, the meaning of what that text is. And then you can store that in a vector database and then perform what's called a vector search against it or a similarity search to find similar, where you literally, you ask a question and you run it through that same embedding model. You get a set of vectors out, you can hand those vectors to the vector database, which will look for similar patterns.
And then give you text content that is consistent with that question. And then, if that text has the answer to the question that is being asked, if you feed that into a large language model, you're much more likely to get an accurate answer. it's a way of taking knowledge that you have inside your organization.
And combining it with intelligence because the two are hand in hand. databases and data storage. And the internet has always been about the accumulation of data and ultimately knowledge. And the difference between data and knowledge is that data is raw information. Knowledge is it that information that's been analyzed, some sort of analysis has been done on it, and conclusions have been reached from it.
And it becomes knowledge when there's some conclusion that comes from it. And what's interesting is that knowledge that's been generated by people can be provided to these large language models and they are better, but they also are helping to generate, they also can go through this process of analysis and actually generate knowledge and answers from raw data, which is also another quite exciting thing that you can do with these language models.
Richie Cotton: Thank you. There's a lot to
Bob Muglia: I never
Richie Cotton: Um,
so
Bob Muglia: there's so much you can do right now. I mean, there's just so much you can do right now that literally you couldn't do 18 months ago. I mean, none of us could do it and now we all can do it.
Richie Cotton: absolutely. It's very exciting times. So I'd maybe, I'd like to get a bit more into vector databases. I, I feel like when it comes to generative ai So the large language models take a lot of the live light, but actually, you need to combine them with a vector database there to really unlock the power.
So, I think you've mentioned Pine Cone briefly. do you want to talk a bit about like maybe what's happening in the Vector database space? Like who are all the different players and what can you do?
Bob Muglia: It's really funny because, a year ago, or nobody even knew where this stuff was. I mean, a vector database was like vector. What? And it, because these language models and the embeddings that they create are effectively vectors. They've become very, important and essentially they're just a way, essentially, they're just a way of storing information that is associated with some sort of semantic.
And that's the really key thing is that if you look at, how search has historically worked, It's historically been a textual based search where you're looking for key, words in text, and that's, the way we've done it for now. that's simplistic going forward.
I mean, in the future, I think what people are, what we're gonna be doing more of is taking information, vectorizing it, running it through, machine learning models to actually understand the semantics. That is within the that content and then using more natural language questions to find out information, and then augmenting the model.
With knowledge that comes from the Vector database. Even GPT four was trained two years ago. So there's no information that's more relevant that's more up to date than that. The way you can bring it up to date is by providing it with more information in the question that you're asking.
So you can take information that you, if you have a question that you're asking, You can vectorize that and get the semantics of that question and look in the content that you already have to find relevant content, and then feed that to GPT four, which will then give you a much more reliable answer.
Richie Cotton: It's pretty amazing stuff. so for organizations who are wanting to adopt gener of AI products, where do they get started? Cause it seems like there are so many different technologies and they're all moving pretty quickly, so.
Bob Muglia: The first thing I would say is get your data assets in order. I mean, that's the first thing, which is, most companies are still not fully on the modern data stack. we're still in the transition to the cloud for data analytics. And you really have to have done that.
In order to really be able to take advantage of this, you need to get your data together in one place. You need to have it in a solution where you can govern it and manage it. One of the really important things to remember is that when you take your data and you bring it all together, You need to secure it because in an odd way, when data is under a person's desk, it's oddly secure, right?
it's hard to get to that information, but when you have it in a centralized situation, you have to really determine access control and things like that, which is really what the modern data stack is all about, which is being able to accumulate all your data in one place, provide access to that information in a governed and controlled sense.
So that the people who have access should have only the access they're allowed and others shouldn't be able to get at it. Now, once you've done that, Now, I mean, again, I think You look at your modern data stack provider, whichever one you're on, and probably look to the tools that they're providing or the partners that they're working with are providing to help to facilitate this process of building these intelligent data applications that include artificial intelligence and large language models.
I mean, there are. A small number of organizations, on the planet that can just push all the stuff together. And they have the chefs and the, data engineers to do it. But most companies don't have that expertise. And the fortunate thing is we're just literally months away from not needing that expertise.
So right now I would experiment, I would play, I would try and understand what applications are the most valuable. So, I would try and understand where you want to build something. And then I would, work with your provider, your modern data pro stack provider, whether it's Snowflake or Databricks or Microsoft or whoever it is.
And work with the tool sets and the partners that they encourage you to work with to actually build these solutions. Cause again, the tools are gonna improve at a very rapid pace, very rapid.
Richie Cotton: That just seemed like a really important point though This. Worth repeating the idea that you do need to get all your data into a sensible position, understand what you have, and make sure it's accessible by the right people. Before you start thinking about like, put this into ai.
Bob Muglia: Otherwise, you're just data wrangling. You're just messing around. Your biggest issue is just finding the data and getting it into the right format. And fortunately, that was a problem that was really hard to solve. Eight years ago, and now it's a very solvable problem. It's a very solvable problem for any organization of any size.
People just have to go through and do that and that is a core step in preparing them to really move forward into this artificial intelligence world.
Richie Cotton: You touched upon. Data privacy briefly, but more generally, can you provide some guidance on how companies can use ai in an ethical manner?
Bob Muglia: Is it privacy or privacy? I always joke about that. It's.
Richie Cotton: Uh, Is it, I'm very British in my pronunciations.
Bob Muglia: That comes out, the British side of it comes out. I just had to, I had to kid you there, Richie? this is a key issue for everybody and honestly, it's a lot of work. It's a lot of work for any organization to do this. And while the tools exist, in the modern data stack to actually enforce the privacy and things like that the hardest part is actually understanding the roles within your organization.
And making the business decisions as to who gets access to what data. And this is actually incredibly important. I mean, it's something everybody cares about. how you manage your customer's data is of concern to everybody. So, building those policies, establishing those policies, Essentially in stone. I mean, make them very rigid and well understood within your organization and then implementing them through your technology stack are really critical. And it's something that every organization needs to do as they centralize their data. And it's actually one of the hardest things.
It's honestly one of the hardest things cuz you have to make a bunch of business decisions. As to who gets access to what information. And that's somewhat, The technology is always challenging, but it's always the people side of these things. That's the most challenging.
Richie Cotton: Oh yeah, certainly. I'm sure like you tell data scientists, they're not allowed
Bob Muglia: And they don't like that. They don't like
Richie Cotton: says, yeah,
Bob Muglia: Or marketing people, I mean, but you really have to ask yourself what information should you know, your product marketing people have access to? I mean, it's a fair question, right? And if you're in the sales organization, you should probably have access to your customers.
But not somebody else's customer information.
Richie Cotton: Are there any common mistakes that you think businesses make when it comes to this? So,
Bob Muglia: They just don't do it. They just don't do what I just described. I mean, honestly, people don't put they do not. We didn't ditch Snowflake. I'll just say this. This was Mark one of our, early on, one of the biggest things was gaining control. We did, that was years ago. I mean, it's been fixed for years but early on, making sure that.
Only the right people have access to all of the information. Now I have to distinguish in Snowflake, it's really important there's customer data. The customers would load into Snowflake, and we never had access to that. Never. But then there's all the data we collect about that, which was a tremendous amount of information and some of it, which you could consider reasonably proprietary, like schema information, things like that.
And so it's really important that, that information only be accessible to people in the circumstances within a company where it's appropriate. To do so, and it really is a policy conversation. That's why the hardest thing about this isn't even enforcing it in the systems. It's establishing the business policies around it.
And it and almost nobody had this a few years ago because again, the data was all over the place. Nobody could find it. It's very secure. When you can't find it, it turns out.
Richie Cotton: That's absolutely fascinating. And to say like, the sort of general theme is like, if you want to use ai, you gotta do data governance, right? It's if you wanna play with your toys, you gotta clean your room
Bob Muglia: Well, And you know, the thing about it is that you really wanna be careful because you wanna make sure that when you are, for example, doing fine tuning on these models, That you're doing so in a way only with information that should be put into those models. Right. you don't wanna contaminate those models with data that they shouldn't be looking at because especially the, you have very little control.
I mean, it's always, it's statistically, once the data's in there, it's statistically determined what the damn thing's gonna say. we don't have direct ability to control it. We only have indirect by control it by tweaking the weights associated with the neural network.
Richie Cotton: And I suppose it's one of the hardest things to deal with then because it's only gonna cause a problem very occasionally. So if you've got like a 1% chance of or even like, no 0.1% chance of like some data, like being regurgitated by the ai, it's only gonna like, appear very
Bob Muglia: It's ter. It's a terrible thing cuz it's indeterminate when it would happen, but I'll make the point. It's not hard if you set it up correctly to begin with and you only do the training, you're fine tuning on data that the model should be looking at. It's fine to fine tune this model on a specific customer's data.
As long as that fine tuning is associated with only that one customer, I mean, that's a fine thing to do. And in fact, that's exactly the way some of these new products are working. One of the, one of the companies that I'm involved with is called Domi and what they're focusing on is using these large language models to take a business contract, which is formatted.
It's very formatted in a way that's, Text and paragraphs and things like that, and inverting that contract into a data document that describes the data within that contract, and then allowing you to use that to build new contracts and things like that. And, the way they have done this and managed to maintain privacy, which I think a best way to do it is they have.
They took a model. They have, it is all done through open source models and they have, there's a standard open source model that, that's been trained on your general information. Then they do additional training on that model with business contracts that are openly available on the internet, so that are similar to customer contracts, but are not, that don't have customer relevant data in it.
And then, and they call that the green loop. Okay. The green loop, because it's all safe, everything's safe in there, and then when you read it, then when you load documents, to do the analysis, you load a number of documents. These contracts into do umami and it goes through what's called the red loop where it does another level of fine tuning and training that's based on the contents of that customer set.
But that information is all contained within a customer and it's never escapes from that customer. And that's the right way to do it. Where you have general open training to understand English and understand general business terms, things like that. But then when you're dealing with the specifics of a business and a specific of a customer, that training has to stay isolated and again, this is doable.
You just have to do it. You just have to structure it, and the tools will facilitate this over time.
Richie Cotton: Okay, so I, it just sound like, the tooling not with the problem is just, it's really a case of getting those business processes right, to make sure that you're very clear on what data is
Bob Muglia: And they'd have tools that make that easy, right? I mean look, the DO AMI team did, these are data scientists. These are sophisticated engineers. I mean, this is top people in the world doing this. And they built a bespoke system that's specifically designed to do what they're trying to solve.
That's great. They're an I sv. They can do that. If you're a enterprise and you're trying to incorporate this into your business applications, you probably want to use tools that help facilitate it. And again, while those tools don't quite exist and we're just on the cusp of a whole bunch of things coming out and we, within 12 to 18 months, it's gonna be, we're gonna go from rags to riches, I think.
Richie Cotton: I absolutely look forward to that. That sounds amazing. I want to take a little side step into science fiction now because you mentioned Isaac Asimov like several times in your book, and so I like to know a bit about how Isaac Asimov in his science fiction and other writing is influenced your opinions on ai.
Bob Muglia: when I was young I read a large amount of Isaac ESMs novels. The man was unbelievably prolific. I mean, he wrote and edited almost 470 books, which is crazy. Having written one book and knowing how difficult, it's, the idea of four 70 is beyond my comprehension. And he wrote about intelligent machines in the form of robots.
over 70, almost 80 years ago, for goodness sakes. And he first defined these things he called the laws of robotics in the early 1940s before digital computing was invented. If you can really even imagine that. And, he was the first writer to imagine an intelligent machine.
As something that is a tool created by man to help people versus some. Being that we shouldn't have, Frankensteinian being that it was, that, that we were tampering with God. I mean, that was everything before, that was when, anything of a, of any kind of legends or anything where people were creating some entity or machine, it was always, we were tampering with God before then.
And Moff didn't see it that way. He saw this as a technical project and he did, he was the one who coined the term robotics. So, Which is really the technology of building robots as tools to help people. And he very much saw that there's be a lot of complexity of.
Intelligent robots living and working with people that are highly imperfect. And he came up with these three laws, the initial three laws of robotics. You know that a robot may not harm a human being or through an action, allow a human being to come to harm. That's the first law. A robot must obey the orders given to it by human beings, except where Souras would conflict with the first law.
That's the second one. And then the third law is a robot must protect its own existence as long as such protection does not conflict with the first or second law. His stories, that he wrote in the 1940s and the 1950s on robotics were really parables of, essentially machines that were perfectly obeying these three laws, interacting with much less perfect humans.
And the challenges of that, and, one of the, one of the criticisms of asthma's laws is that, What does it mean to injure a human being or to allow an, in a human being to come to harm? Of course, it's a very vague term, and we can think of that in very broad ways.
It turns out that as Mo's writings were largely about what that term means. I mean, he explored across these different stories, different kinds of harm and, how that harm was inflicted and, how the robot had to respond to that because it was following the laws. And I just think that, These ideas of these laws which were burned into the brains, a posr brain that ov invented.
We are gonna have anything quite like that with our. AI in large language models. We can't burn laws into the neurological circuits of a large language model but we can build the rules in which these things operate. And I do believe these laws Our useful guidelines for us as we create intelligence.
And the other thing that to me was even more remarkable is that ASMO put aside the robot stories for almost 30 years. And then he came back to him in the 1970s and he wrote a few novels late in his career. And in those latter novels some of his robots were more advanced and had become what we might call a.
A g I or artificial general intelligence, or maybe even a super intelligence. And, in as MO'S writings at that point, these robots were interacting with, leaders of ppu a society and having you an influence on it. They're very benevolent, okay, because they were following the laws, but in it, the robots realized that the laws were insufficient and asma added.
What he called the zeroth law, which is, a robot may not harm humanity. Or through inaction, allow humanity to come to harm. And when you hear, Jeffrey Hinton and folks talking about existential risk of artificial intelligence, it's really about what happens if these things become super intelligent and get beyond us.
And, there again, I just really think that what Moff was trying to say is we need to make sure we put rules and regulations in place to ensure that the tools that we're creating. These entities, whatever they are, are actually working on our behalf. And again, I think that this is a good approach to, it's not, you're not gonna, that's not how you build the large language model, but it wasn't how you built the Positronic brain either.
in there. It was simply the rules in which these things should operate. I just think there's a lot to learn from it.
Richie Cotton: Absolutely just seemed like, fiction science. Fiction in particular is very good for trying to figure out, how the future ought to be.
Bob Muglia: Well, It's remarkable timing even is about right. I mean, even if you looked at where he was talking about, it was like the 2030s and stuff. I mean, it was all roughly the same timeframes and that he was talking about, I mean, I think he was a prophet. I just really think he was a prophet and there's a lot we can learn from what he wrote.
Richie Cotton: Absolutely. Maybe time to dig out some of his books from the library, I think.
Bob Muglia: One of the most enjoyable parts of writing this book is I've reread a whole bunch of the OV robot things and robot novels and robot stories, and they're really great. They're really fun. They're old and some would quaint perhaps, but they're really wonderful.
Richie Cotton: While we're talking about the future of AI and things like that. So your book has something you call the arc of data innovation with different steps that lead towards the idea of technological singularity. I think. think it's one of Ray Kurzweil's ideas, but can you tell me a bit
Bob Muglia: I have the book right? I have the book right here.
Richie Cotton: Oh, he's got his book.
Bob Muglia: Well, let see. Well, I first started writing the book. I knew there was always an arc of data innovation, that was a part of the book and it was an important element of it. But two years ago when I first started writing it, I really thought the arc ended in, the data economy and how data is gonna change our world.
And it has been changing our world. And, what's happened is and to me the big thing is that with the advancements we're seeing in artificial intelligence, I've come to realize that there is a very high probability that, I will see artificial general intelligence and may be able to make use of it in my lifetime.
And I think it's gonna happen within 10 years. So I mean, I had thought that the horizon for this, I've always believed this was coming. I mean, as inov and as a kid inov, I always believed these robots were gonna come someday, but I thought it'd be 2100 or something that we would see this and therefore I didn't think I would see any of it.
But now I think that, even a 60 plus year old will see, a g I and perhaps super intelligence. And a technological singularity. Let's talk about what it is a technological singularity is an an advancement in progress that is beyond human speed. And what we've been seeing, in a way for thousands of years, but very much since the advent of the digital computing is an acceleration of progress.
And that's what's represented in the arc of data innovation. It's really an acceleration of progress. Things are going so much faster. I go back to. When Bill did his information at your fingertip speech, things moved a lot slower then than they move right now. stunningly slower than they move right now.
And we have continued to see this progress in acceleration of pace of technological innovation, and there is no question. That these artificial intelligence systems that we're building are going to accelerate that. It will accelerate that. And another potential major accelerator is quantum computing, if, and when we see that, I didn't put that in the arc, but it's another big one potentially.
And ultimately on this, it's gonna continue to keep getting faster and faster. Does it ever get to the fast, fast? Well, We can't keep up. Well, I don't know. That's what a singularity is. And if that does happen, I just wanna make sure that wherever it's going in a direction that's beneficial to mankind, and I think we can do that.
I think we can achieve that. I've always been a technical optimist. I've always believed that you can specify what you wanna build, and I think we can be successful in this.
Richie Cotton: I've say that's like one of the bold claims made on the podcast that we're gonna have artificial super intelligence in our lifetimes. I'd be amazed if you're right, that's that's gonna be, it's gonna be astounding.
Bob Muglia: have artificial, like you I, I think you're gonna have artificial general intelligence really, because it's getting there at a very, at a fairly rapid rate. I mean, it really is. It's getting it's is increasing reasonably fast. Whether it gets, progressively smarter. That's a really interesting question.
Richie Cotton: So, do you think there are gonna be any important issues that these sort of more powerful AI are gonna be able to solve in sort of humanities near future?
Bob Muglia: Well, I think they're gonna help us in all kinds of things, right? I mean, I think they're gonna help us to accelerate. Medical science and drug discovery. They'll help us, in diagnosis of medical issues. They're gonna automate business processes and make business processes more efficient.
They will help us in, all kinds of potential discoveries. I think that between AI coming out and being able to see some and solve some problems, and then potentially quantum also coming. It's, quantum can solve a whole set of problems that are essentially completely unsolvable in the digital space.
And so it's gonna be a fascinating timeframe. There's a lot of discovery out there. We don't know very much about the universe, there's a lot more to learn and we're learning it faster and faster.
Richie Cotton: Definitely agree. There's so much more exciting stuff out there to learn. Always. Yeah. So, do you think there're gonna be any sort of unexpected consequences once you have incredibly powerful ai?
Bob Muglia: There's gonna be all sorts of unexpected. The thing about this, and this is really important, what, you know, we're talking about the existential risk a second ago. That's the long term risk, in terms of super intelligence. The short term risks are that these are powerful tools that can be used by people to do things on behalf of people, and like every other tool created by man.
It will be used for every possible purpose. The good, the bad, and unfortunately the evil. And everything we've ever built has been used that way, right? I mean, every, everything, the hammers have got lots of positive uses and they have some negative uses too. And the one thing that I think is purely negative is nuclear weapons.
You compare AI to nuclear weapons, to me, they're incomparable in one sense, which is that there's no. Productive use of a nuclear weapon and there's millions of productive uses of ai those potentially have some existential risk. Although if you ask me, I'm still more worried about the nuclear weapons, I think, than I am about the AI in terms of existential risk.
But but that's, I was a Cold War baby. I grew up in the, in, during that era the duck and Cover era. I remember what that was like. But, people are gonna do everything with this and there's gonna be, we're already seeing, terrible deep fakes being built.
Seems like every day the news has some news story of ai, whether it's positive or negative, whether, it's some new horrible deep fake that somebody's doing to, cause problems or whether it's a new Beatles song that includes John Lennon's voice in it, be created by artificial intelligence, which I would say is a, is certainly a positive thing you're doing.
Can be done.
Richie Cotton: Absolutely. I mean, it's just such a huge range of possibilities for good or bad for this thing. So maybe can you tell me like what you're most excited about? As far as a AI goes Well,
Bob Muglia: I guess, it's hard to put a finger on this because every single thing is going to be reinvented. again you have intelligence in a computer now for the first time and for the first time. A computer can actually understand and respond to English and other natural native languages.
That's remarkable because it lowers the barriers between man and machine dramatically. I have people been talking about these autonomous vehicles, all these things. You still need a way of communicating with the device that you're dealing with. I mean, I always wondered, until like last this year, I was wondering how are you gonna, when we have these autonomous Ubers, that take us from place to place.
How are we gonna tell the car where to drop us off in the end? Because every Uber conversation, every Uber ends they'll know what to do. So the fact that you can use English, so like for example, sequel.
Which has been such an important language in data analysis. I mean, it's the lingua franca of databases. it's going to become more of an intermediate language and English is going to become the primary language for data analysis. And people are gonna express their queries in English, which will then get translated into sql.
It's still gonna get translated into sql, but English is gonna become much more important. And that was not really. Foreseen as possible. I mean, there's a number of people trying to do it but it's only become really viable in the last really six months.
Richie Cotton: Absolutely. I mean, doing data analysis with just natural language is just such an amazing improvement in many cases. Absolutely brilliant. Not having to remembers syntax.
Bob Muglia: at the moment, the only model that's doing a decent job of it, I think is GPT four actually. I mean, I don't even know I don't know if there are any, you know that when I hear people are working with, Translating into sql, they seem to be using GPT four because they need the reasoning capabilities that model has.
So, like I say, it's really recent that this seems to be breaking through. I suspect, my, my view is that in, in 12 to 18 months, there'll be open source models. There'll be capable of doing that, but right now it's not true.
Richie Cotton: Alright, so before we wrap couple of not AI questions for you or, but there, there could be AI depending on how you answer them. So, first of all, like, if you've been involved in like so many different companies over the years, so I'm just wondering if you were gonna start a new company today, what sort of area would it be in?
Bob Muglia: Well, I think, what I would say to anyone is under take whatever domain you understand and see how you can use intelligence in the form of the zais to actually reinvent that space. Cuz every single application space has a potential to be reinvented. With ai now, it'll be interesting, AI can be additive to existing applications, so it's not clear that there'll be that, the incumbents are gonna lose in this battle.
And if they, if the incumbents do a good job of incorporating the intelligence, they're well positioned to be successful going forward in whatever category you're talking about. Like, for example, Adobe just added. I added generative AI capability to Photoshop. That's an example of how an incumbent can take and augment their tools with ai.
Will that be the successful road or will be at a successful road of a whole new product in that space? Unclear still right now, still unclear. But there are whole new category is that can be, that can be created. One of which, I mean, my favorite one that I am personally really interested in is understanding the semantic model of a business.
Understanding all of the nuances of that business and then having that be effectively structured in a business system. I think that takes the form of a knowledge graph of some kind that needs to be created. That technology is also still emerging. So it's just a whole, new areas. But the capability of using intelligence to be able to actually determine the business process is very powerful because in many of these large companies, I think the process has become so complicated.
People don't even understand them fully. And AI can help.
Richie Cotton: I like that idea that, start with like what you know, and then just think about how these new technologies can help you out. So yeah, just seem like that some great opportunities there for anyone. So I guess just to finish do you have any more a advice for aspiring data entrepreneurs?
Bob Muglia: Follow your dream, really. I think it's a great time. if you have an idea, if you would like to be entrepreneurial. It's a great time to do it. It really is a good time. there's a lot of new things you can work on. It's a very brave new world with this new technology.
Nobody has too big of a lead on it right now, so there's just a lot people can do. But, take what you know and apply it. In a way that really solves problems in a different way for people, but leverage your strengths. That's my best thing to say to everyone. Leverage your strengths.
Richie Cotton: Great advice. Thank you so much, Bob, for coming on the show. Loads of great advice throughout that's been, it's been great having you.
Bob Muglia: Great. Been good talking to you, Richie.
You’re invited! Join us for Radar: AI Edition
DataCamp Team
2 min
The Art of Prompt Engineering with Alex Banks, Founder and Educator, Sunday Signal
The Future of Programming with Kyle Daigle, COO at GitHub
A Comprehensive Guide to Working with the Mistral Large Model
Josep Ferrer
12 min
Serving an LLM Application as an API Endpoint using FastAPI in Python
How to Improve RAG Performance: 5 Key Techniques with Examples
Eugenia Anello