How a Chief AI Officer Works with Philipp Herzig, Chief AI Officer at SAP
Philipp Herzig is the Chief AI Officer at SAP. He’s held a variety of roles within SAP, most recently SVP Head of Cross Product Engineering & Experience, however his experience covers intelligent enterprise & cross-architecture, head of engineering for cloud-native apps, a software development manager, and product owner.
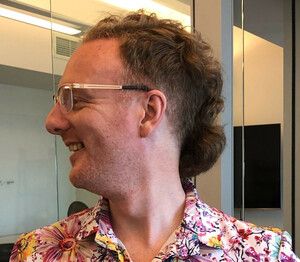
Richie helps individuals and organizations get better at using data and AI. He's been a data scientist since before it was called data science, and has written two books and created many DataCamp courses on the subject. He is a host of the DataFramed podcast, and runs DataCamp's webinar program.
Key Quotes
There's all these things that change all day long, right? The tech changes, the LLM changes, the tools change. But what shouldn't really change are our values. Like, how do we think about how the world looks in an AI age and what is important to us when we design AI into our workflows and applications? And that is also something that we are continuously evolving.
I think it's always important to stay hungry and foolish and keep trying things. But it also can get daunting sometimes, in particular in this fast paced environment that we are in. I can only give this advice to everyone to just continue. There will be also lots of stressful and painful days. But I think overall, if you can train your resilience in the current times quite well. And so far, I think the reward is much higher, bottom line. And if I were just going to go back and say, yeah, know what you're subscribed to, know what you're up to, but then also have the resilience and the patience to work it through.
Key Takeaways
Incorporate AI across all business functions, not just in isolated areas. This approach allows for a comprehensive and cohesive AI strategy that aligns with overall business objectives and enhances various operational areas simultaneously.
Develop and adhere to an AI ethics policy. This commitment helps maintain trust and accountability, ensuring AI applications respect user privacy, data security, and ethical considerations, aligning with long-term business values.
Engage multiple departments from the onset of AI projects to ensure broad acceptance and integration. Collaboration across departments such as product engineering, legal, and commercial teams can facilitate a more robust and scalable AI deployment.
Transcript
Richie Cotton: Welcome to DataFramed. This is Richie. With every company trying to get in on the act with AI, questions start to arise about who ought to be in charge of these initiatives. At the moment, it's a bit of a toss up between the Chief Technology Officer, the Chief Information Officer, and the Chief Data Officer, or maybe a committee with all three taking responsibility for some projects.
Some forward looking organizations have decided that AI isn't an important enough part of the business that it deserves a dedicated seat in the C suite. The Chief AI Officer role is, naturally, fairly new, so today we're going to explore what such a job actually involves, where it fits into the rest of the organization, and what success as a CAIO looks like.
Our guest is Philip Herzig, the Chief AI Officer at the enterprise software company SAP. He's held a variety of roles in that company on both the engineering and product sides. These days he's tasked with leading the company's business AI strategy. Let's find out about his job. Hi Philip, welcome to the show.
Philipp Herzig: Thank you so much, Richie. Pleasure to be here with you today.
Richie Cotton: Excellent. So there are a lot of technical C suite positions. You've got chief data officer, chief technical officer, chief information officer. How does the chief AI officer role differ from these?
Philipp Herzig: That's a great question. So, I mean, first of all, it's a new role, right? that we also at SAP, we have established at ... See more
For all the functions. I mean, as one of the largest enterprise application vendors, right? Specifically out of Europe. We have to take not of course only the adoption internally, right? For our processes and for our people into account. But obviously how we, think about the products, how we bring AI all to our products.
We can spend a little bit more time, I think, about our product strategy in a minute. And then really think this end to end through, right? How do we do legal aspects, the commercial aspects? And as we all know, right, who are also regularly listening to this podcast, there's so many things that happen every day.
Every, every week, new models come around the corner. Or there's something happening on the regulatory side of the house. So the question is really like, what is the center of excellence or like the nucleus in the company that takes all those signals, all those concepts into account and, fit them together.
differently or every single day, right? And there's every day there are new opportunities, new risks. And in this role, the way how we interpret it is really take an end to end view, But at the same time, it's also just a relatively small department because at the end of the day, and that's part of our strategy as we embed AI specifically all in our enterprise applications is then to work with all the connected board areas, right?
Product and engineering, technology department, the CTO, the CIO for internal adoption our sales and marketing teams closely together, right? To then scale the entire approach across the entire portfolio that we have today. It's
Richie Cotton: Okay, so that sounds like you're working with a lot of different teams there. So you mentioned product engineering, the commercial teams, legal teams, the whole lot. Okay, so, uh, can you go into any more depth? Like, what do your day to day responsibilities look like?
Philipp Herzig: So, first of all, as part of this Chief AI Officer mandate we have basically all the roles in there, right? So we have, for example, part of my team, that was the team also before, right? So AI is not a new thing. I was Before that, I was on the engineering side of the organization, right? This is also why this cross collaboration and this work is so natural to us as a team because I was actually part of the product engineering board area within SAP, right?
Where all the applications like our ERP, our HR applications, finance, supply chain, procurement applications like Concur, Fieldglass, Ariba, SuccessFactors, some of them you may know, are located within SAP. And, I was always the cross engineering guy, if you will, right, looking top to bottom, looking at our user experience, looking at our process integration, our technology adoption with respect to our technology platforms.
And AI, we also did in the last couple of years there as well, and we moved it out. Directly on our CEO Christian Klein. And engineering is still with us where we build platform services, like for example, Joule, our generative AI copilot, which all of the applications are adopting, where we build what we call the generative AI hub, which is kind of the glue, if you will.
Where we bring the best of large language models or foundational models together with the real time data that is stored within SAP and non SAP systems. A couple of other reuse services for document information extraction, data attribute other recommendation services that are kind reuse services that you can embed in multiple different applications.
So we build those, but that's part of our engineering layer that we provide as a service platform service to our own applications. But then of course, we have a dedicated product, a partner management team. We have a dedicated marketing team. We have a dedicated go to market and sales team. We have a dedicated implementation adoption team that helps, you know, customers than all the innovations that we are building to adopt them very quickly, but also funnel the feedback from those customers back very quickly.
And as you can imagine, what does my daily job look like? Like all of those things. So every day we look at what's, new on the product side of the house, right? How can we bring Directly to the customers. How can we enable our field with that? How can we turn the first customers right? Really to adopt also our latest innovations with that.
what is the impact on our marketing approach? and we are reviewing all of those, things, every single day in order really to move really, really quickly and in alignment with our customer's expectations.
Richie Cotton: Okay, so it sounds like you've got quite a lot of different AI products going on there. I'd like to get into a bit more about where your role sits within the organization. So you said that you work with a lot of different teams. So who do you report to?
Philipp Herzig: Yeah, so I report directly to our CEO, Christian Klein.
Richie Cotton: And then Who is in your team? Like who reports to you?
Philipp Herzig: So within my team, there's, for example, Walter Sun, who joined us last year in September Microsoft there working also in the business application space you know, around Dynamics 365 and, some of their platform services, and he runs all of our AI engineering functions, both our research that we are doing with universities, you know, like Stanford and Berkeley and a few others in Germany, like Hasselbladner Institute or the Technical University in Munich and a few more up onto all the services I described already, right?
So really on the technology platform, all those reuse services that we are building. And then we have, as I said, right, we have a dedicated leader, a very renowned leader within SAP Jan, who reports to myself, looking after go to market and, spreading basically across all the regions enablement material, right, and, how we position, how we are selling, then also AI our customers.
We have a dedicated marketing team. Product and partner management as we also as part of our strategy. We are partnering with many of the companies with Microsoft, with Amazon, with Google and many more, NVIDIA. then on the other side, of course, we enable all the other partners, right? So obviously SAP is also happily partnering very closely with all the global service partners.
and consulting organizations around the world in order to also scale from an implementation and consulting perspective as well.
Richie Cotton: Okay. So it's not just working with internal teams. You're doing a lot of partnerships with other companies as well. Since that external facing side to the role too.
Philipp Herzig: Yes, exactly.
Richie Cotton: Okay. So, when you're working with so many different teams, I imagine like the communication flow and like just managing the logistics of doing so many different teams is a challenge.
Can you talk me through how you approach that?
Philipp Herzig: We have kind of, for all the various functions, we have very close and regular alignment meetings. Give me just one example. Already last year, specifically when the entire hype, right, around chat GPT and what do we now have to do with respect to generative AI, What does this mean for us, right? And how do we bring this into our applications?
How can we help, right, to make HR more productive, or shared service centers more productive, sales more productive, services, marketing? And we formed a little group already last year, it was January or something, where a small team and thought hard do we make HR more productive? how do we, build the first scenarios basically because our strategy just here to back up a little is really, first of all, SAP does not strive so much for a general Purpose technology platform, right?
That helps customers to build AI, like, tools for comp engineering or retrieval, augmented generation or data pipelines yourself, right? And then you have some smart people who can build those things like a chatbot or digital assistant or something. Our strategy is that these things are embedded in the applications and just turn them on and just work out of the box, right?
And. So the question first was, what were high value use cases that we would develop that where customers say like, okay, that is actually something where I see generative AI embedded into an SAP application actually helps me. And we figured those cases out very quickly and just started executing.
It was a small, nimble, but very capable team that we've started with in order to, to move that forward. And it turned over the last year. It turned out into the standard call. We run it on a, on a weekly basis when we actually wanted to release our first scenarios was actually on a daily basis where with all the teams, right?
We went through all the challenges that are there right in order to productize them and put them in front of the customer. And we are still running it ever since. So all the graph, meanwhile, released via this alignment call released. 39 generative AI scenarios in Q4 and Q1 alone. There are 40 more in Q2.
Till end of this year, there's a hundred more that we are releasing and this is how we are aligning that, right? And every, every time somebody comes up with a new challenge, right? Or a new thing that needs to be addressed or that needs to be rolled out because we come up with a new concept on the commercial side of it.
And we are doing this all in this one, single call, if you will, in order to really let the flow of information flow throughout as a, as a obviously very large company. And so far it worked very well.
Richie Cotton: Okay. So it seems like you just do lots and lots of communication with lots of different teams, just to make sure everything's
Philipp Herzig: I appreciate it. And then also as part of my previous gig, just also how do we align architecturally, because I think that's also something that I'm very at least very happy with. SAP is a very heterogeneous company, right? We have many lines of businesses, right, in the various areas. But also as part of my previous role, and that helps obviously a lot I was running what we call cross product architecture.
So that was a approach we established in the last three years to really get all the various lines of businesses, no matter whether it's Concur or whether it's SuccessFactors or Ariba or SAP S4 HANA, to agree on what we wanted to do. the common technology foundation, a common set of platform services and capabilities that everybody has to adopt in order to come up what we call suite qualities, right?
So a set of qualities that are equal, right, amongst the various applications. Very simple things like that. All the mobile apps, right? That the login works the same way. All of them are using, let's say, Face ID or have the same look and feel. Down to, okay, the identity management is, for example, harmonized.
And that was a huge program we did over the last three years. And that obviously helps a lot, right? Because now we could design also the AI foundation for all the applications the same way. So when I talk, for example, about juul, our generative AI copilot, this is not like a JUUL for HR or a JUUL for finance or a JUUL for supply chain.
No. It's one jewel that sits at the heart, right, of the architecture and all the applications are connecting into it. And the more applications you connect to it, the more powerful the assistant eventually becomes.
Richie Cotton: Okay. So that seems like an interesting thing about AI is because you require all this data, having a single AI connected to lots of different things is going to be more powerful than having lots of individual. Yes. Okay. All right. So, I'd like to know a bit about what constitutes success. So, how do you know if you're successful in your job?
Philipp Herzig: So first of all, it's all about basically three main aspects, right, that we always consider. First of all, it's do we deliver the right scenarios for our customers, right? So that it really has the relevance to be adopted at the end of the day. So the ultimate success metric for us is clearly adoption specifically in the cloud, right?
I mean, you can build That's most amazing thing in the world. Nobody actually adopts it. Probably built the wrong thing. So we are entirely guided by adoption and making sure it can be easily turned on, out of the box, it's available as a service, because we figured out over the last couple of years that specifically, you know, if it's not delivered out of the box, not as a service, adoption usually is very hard.
that's number one. The second one is clearly reliability. I mean, we all know what the limitations of large language models are, right? With all those things, hallucinations, only has been trained on public data up to a certain point in time. Of course, they do not know the current realities of your business.
Like, oh, where are my sales orders? Where are my general ledger accounts? where is my current goods receive or where's the delivery right of a certain of certain goods, et cetera, et cetera. So we need to bring together in a reliable, secure, and data privacy preserving way the real time data of the, SAP systems together with the large language models in order to.
Bring in the contextual knowledge you need in order to make actually the relevant uses cases to happen. But of course, we want to do that in a very secure and trustworthy way because this is what our customers come to expect from SAP for decades. And the last piece I'd like just to mention is also our approach to responsible AI.
So, SAP was one of the first companies to establish what we call an AI ethics policy back in 2018. And it guided us well through the years. So this is really, there's all these things, Richie, that change all day long, right? The tech changes, the LLM changes, tools change. But what shouldn't really change are our values.
Like, how do we think about, how the world looks in an AI age and what is important to us when we design AI into our workflows and applications. and that is also something that we are continuously evolving and that's also part of one important success metric that we're always sticking and staying true to our values when we design specifically generative AI into, into our apps.
Richie Cotton: like that your sort of main judgment of all criteria for success is just like you're shipping AI products and your customers appreciate those. I'm wondering like in more depth, can you talk about like, Japanese specific metrics or targets that are used to evaluate your success as a chief AI officer?
Philipp Herzig: Well, we obviously want to get as much customers to adopt our things there in total. I mean, overall, what I can just share is we have already, I mean, I mean, AI overall is not a new topic for SAP. We have roughly 27, 000 enterprise customers right around the world who are using our AI capabilities.
on a regular basis and yeah, of course we our ambition is to heavily continue that growth from an adoption perspective in the years to come and specifically with, with generative AI
Richie Cotton: It seems like you've got a lot of different products and projects on the go. Can you talk me through how you prioritize which AI projects are going to be most important?
Philipp Herzig: prioritization is always done from a value perspective. So what has the highest value? And it could be multiple things, right? It could be. potential top line impact for a customer, In particular, if you think about Gen AI, the usual Gen AI scenarios in sales and services or marketing so front office related roles, could be bottom line improvements, cost of goods sold improvements through generative AI.
Or just simply as we target that with Jewel to rethink the way how people interact with the system in a way more delightful and easy fashion compared to, you know, rather classical click through all the UIs to accomplish your task fashion. And that is usually the way how we, evaluate the scenario.
So when we go through the scenarios, we are always looking at it from a what's the return on investment from a customer perspective. So, what is really the benefit that helps the customer to do something faster or easier, right? Or helps them to achieve more with less. So those are always questions that we ask already very early in the product lifecycle before we go further down the road in order then to implement and spend our resources those cases.
And this is how we run it for the most part.
Richie Cotton: Okay. So, the three things you mentioned there were improvements to the top line, so increasing revenue, and then improvements to the bottom line, so it's cost saving, and then working better with your software. So I guess it's a customer experience improvement. Can you Go into more depth, just explain to the audience, like, so what sort of projects are going to help improve revenue?
What sort of projects are going to help save costs and what sort of benefits you get from working differently with your software?
Philipp Herzig: So just give you a couple of examples, that we are having. I mean, one of the more classical scenarios that we have been just just to draw on a few numbers on that we have been doing for decades is data entry in your system, right? There's still across all the different business processes.
There's still like tons of paper. And with paper, I also don't be like printed paper, but email and PDF and digital paper is still paper. and still there's like a plethora, right, of processes that require you to tediously, manually enter all of those things, into transactions, into, into screens, right?
And just, you know, Transmit basically what's on paper into that system, and I think there's a lot. There's a tremendous amount of potential to just give you a few numbers alone and conquer, for example, just with B2B in the background, we are processing like somewhere in the range of 60 to 100 million invoices, right?
fully automated fashion, for example, right? And it's just in the system fully itemized, right? They're just in there. There's no manual error prone and tedious work just to key those, key those in. And everybody who has filed an expense report exactly knows how tedious that sometimes can be.
And we can save a hell of a lot of time. effort and money by that, right? In a, in a high triple digit million number range. We also look, for example, into shared service center, right? Think about accounts payables, for example, or accounts receivables. If you have a large shared service center where, all the various complaints, let's say, for example, accounts payables, right?
You have all your suppliers and they are complaining because you may have not paid them, and the contractually agreed on times because maybe of quality issues or right, blocks the payment to the supplier. And you easily, depending on your size of the company, you can, like, have hundreds, thousands of these cases basis.
And then, of course, it starts with simple things like, oh, how can I catch up with the threat and the discussion that was already happening via email maybe with that supplier? So, you know, Imagine there's a new person looking at that thread and generative AI, instead of reading all that emails, helps you to just catch up with that thread in like virtually no time, right?
Versus reading 10, 20 minutes per case through that email. And then you get suggestions what you do with it, right? You can say like, okay, maybe you do a, do a recommendation and maybe give a voucher or give some more time or maybe give a certain discount or all those things, which would be then recommended by a tool.
And then once you have to resolve that problem with that ticket, it then also, of course, helps you, for example, to generate an email. And not just a email like a standard thank you email, or we solve that email, but one that is specifically written with the business context in mind. So having the reference to the invoice number, maybe to the purchase order, right?
So that also the supplier has a good chance to reconcile it again back in their business much faster and much easier. So it's not about Creating a plain vanilla email, but really one with, with context that usually if you would craft this manually, getting all those fields out and so on, the email can easily take you, I don't know, 20, 30 minutes to write, and now we can just do it in seconds, And multiply that with the volume of cases. that's a huge time saver. And it's not only a huge time saver. It will also help, right, to improve it. Either your supplier experience, right, with your suppliers because you're reacting faster, you're resolving the cases faster. And our accounts receivable side of course also helps with the customer experience because obviously customers will be much happier because you resolve the cases faster too.
And those are maybe two examples that I can share. I think that are relatable that are not too deep in the, in the business process world.
Richie Cotton: Yeah, absolutely. It always feels amazingly clunky, like how much effort it takes just to either pay money to another business or receive money from a business. And so just automate that, that just seemed like an ideal use case for AI, speeding things up. All right. So can you talk me through what are the big challenges with your role?
Philipp Herzig: I think overall. One of the big challenges that we deal with every day is how can we absorb the massive change and the massive transformation that is happening at this, to be honest, unprecedented speed. Right. I mean, we had some, some other huge technology disruptions before, everybody always relate compares like generative AI was currently happening with the internet or what's happened with mobile or the introduction of the microchip and the PC.
And the difference is all those trends were maybe as disruptive, but they were not as fast. As this currently happens, because all the infrastructure is there or lots of infrastructure at least is there and it's so accessible and, taking that in and determining what are the right products to build, but then also helping our customers at scale, right?
Not just in a certain market or for a certain pocket of customers. That's simple. that's usually easy, but really scale it. throughout all the various departments within SAP, but then also reach all the customers, the same narrative, with the same message, right? And also keeping them to date challenge.
I just had a, just to give you an example, I just had a partner meeting today and they just told us, Philip, you need to help us to keep up with this pace, right? It's just too fast. That's on the one hand side, it's a, it's a good problem to have, but it's also a clear challenge, right? Because it's, it's, pretty tricky to everything that happens on the tech side, right?
To immediately absorb all the right products and then enable every customer and tell them, look, this is the way how we, how we design it. So doing that at scale for like hundreds of thousands. of enterprise customers is a big challenge. That's a,
Richie Cotton: I'm sure certainly keeping up the pace of AI development is a challenge for everyone. So I'm wondering what's your solution to this? Do you have any advice on how to keep up with uh,
Philipp Herzig: that's a, that's a fun question, but I also have, I think I have a little bit of an unconventional answer for you. So, first of all, I read a lot of things myself. So it helps a little bit that I'm still an engineer myself. So I go into many things also over the weekend. I'm trying out many things.
Some of those large language models, not some of them, I try them all of them myself. Try to run them locally as much as possible. Think of some of the open source models like Lama, right? Or the Mistral models. and then basic, and of course, read all the, read and hear podcasts like DataFrame, for example, and, and others to keep up to date, but also, you know, relate a little bit like different opinions, different point of views on what, what you People think about it.
And then what I basically do is not just hearing those things and trying to understand it for myself. But I sit down basically every week on the weekend and I write all of that stuff down in an email and it goes out to thousands of SAP employees. So it's basically a voluntary subscribe to it.
There's always a link and hey, if you want to. If you don't want to receive this anymore, click here. If you want to receive it, click there. And luckily I get more click there than click here things. And it meanwhile reaches a couple of thousand SAP employees on a weekly basis. And it's not just a rundown or a write down of all those things, but also actually, it's like a big action item.
Like, hey, have you seen this? And in this technology, there's this new model. please evaluate it deeper. It looks good to me. I've tried to following things out. I think that's helpful for this service. I think it's helpful for that service. Or when there is something happening in the market that may relate to some of Our products, right?
Either on the technology side or on the application side, it's like, hey, dear peer L1 leader, please check this out. I think you should do deeper dive on what that means and whether we can incorporate that or if it's of any use. And it's like a, it's both like a rundown or breakdown of all the things that happened in this week.
Plus, it's a Hey, here's an action item. Please come back next week and, please let me know if there's something that's, useful to you. And you know, that kind of process helps to not only digest and by writing it down again, but also to really, you know, reflect on where does this belong to in the company and figure out who should be on point, right, to evaluate whether that technology or that tool or whatever is coming out, but that research paper also read a lot of research papers, obviously and then to figure out what, what that would mean and if we can incorporate those things within our solution somewhere.
Richie Cotton: Okay. Actually, I have to say, I really like that approach. So when you read something interesting, you then think, well, who else in my company is this going to be relevant to? So you can say, okay, this is going to be great for this service or this product. And that way it actually gets used rather than just.
Philipp Herzig: Exactly, which then is kind of this, this translate, right? When this whole thing is not just a whole, here's the rundown, think for yourself, but here's already, I think there's an anchor point here, right? There's an, there's an idea, a concept, and I think you really should take a look. and that helps a lot that people then really take this more seriously and say like, okay, well, then I take a look at, maybe sometimes also turns out I was wrong, that's fine, right?
And, and the approach doesn't work because it's maybe not yet mature enough. Okay. We had this a couple of times. People came back and said, well, yeah, we tried it, but maybe version two. Let's see. That also happens, but, still they, they exposed themselves to, they, they got to know that. And obviously this is part for me of this whole organizational learning that needs to happen in order to stay up to date with what, what is happening.
Richie Cotton: So if you could go back to when you first started this role what advice would you give to yourself?
Philipp Herzig: I think it's always important to stay hungry and, foolish and, try out all these things. But, you know, it also can get daunting sometimes and in particular in this fast paced environment that we are in. And I can only give this advice to, to everyone to just continue.
There will be also lots of stressful and painful days. But I think overall, if, you can train your resilience in the current times quite well. And, and so far, I I think the reward is much higher. Bottom line. And yeah, if I would just gonna go back and say like, yeah, know what you're subscribed to, know what you're up to, but then also have the resilience and the patience to work it through.
Richie Cotton: All right, super. So, I have to say you weren't selling me the role to be able to say, yeah, you need a lot of resilience and a lot of pain here, but I like that there's some good rewards with the role as well. Okay. So, can you tell me what you're most excited about in the world of AI at the moment?
Philipp Herzig: I think just top of my mind right now is I think just last week is the release of, how do you pronounce this? Is this UDIO or something? Because I'm also just on my personal level when it comes to AI progress, because I'm a want to be a musician for 20 years. I think we just reached the next leap in terms of music generation.
So that is something that is like, extraordinary to see and knowing what it takes also, you know, to play yourself and create and design and play music for years. But no, I think overall it's just exciting to see really that, with AI in general, I think we are at the tipping point of an entirely new century and having the privilege, right, to be on the forefront and, being able to influence and design also both in a relevant, but also responsible way, this technology for enterprises around the world, right? I mean, The largest companies on this planet rely on SAP, and I think simply the impact that we can create with that, again, in irrelevant, but at the same time, responsible way that is this, just mind boggling on the impact that this can create in the next years to come.
So this is, this is what, what motivates me every day because it's, it's really, really impactful, the work that we can do.
Richie Cotton: Okay. Yeah, you're right. There's so much amazing stuff going on. I would also like to second the idea of having AI generated music. It's I always think like It would be kind of nice to be a rock star, but also I have zero musical talent. So if technology can bridge that gap, then so much the better.
Philipp Herzig: I don't think that everybody was going to be a rock star, right? Because while it's impressive, right? At the end of the day, there's still always some, music. And this is something where I honestly believe Jenny and I will never take this away, music, also programming, right? These are more like liberal arts than, just.
mathematical things. And, you know, there's a big difference between a solid and well constructed song and a song that touches people. And, to be honest, I think this will never go away.
Richie Cotton: Yeah. Okay. Maybe my rock star aspirations have been dashed once again. All right. So, do you have any final advice for aspiring chief AI officers?
Philipp Herzig: I think the chief AI officer, for the most part, needs really a heterogeneous set of skills. So I think on the one hand, obviously it helps a lot to, be knowledgeable in AI and know the technology. You don't, I don't think you have to be the top most expert in that domain, like 10 years data science, right?
And, written tens of research papers like with Google DeepMind and others. But I think it's in particular the combination of skills, right? You need to understand technology well enough in order to understand its implications what that means, where the market is going and combine that with the skills on how to identify the right scenarios, do a lot of stakeholder management, come up with the right architecture find the right messaging and narrative from a marketing perspective determine how you can actually also, you know, from a, from a cost, but also from a revenue or sales perspective, right?
Relate that so that you come up with a viable product. So all these various dimensions I think are critical. As a chief AI officer to be really successful at the end of the day. So it really needs a, I think, a well rounded profile, right? Of all these various things in order to bring those concepts together.
So I can I can only recommend aspiring chief AI officers to really look at this topic, not purely from, let's say, one angle, like, let's say technology, for example, but really, you know, read and, educate yourself in a holistic fashion with all the various aspects in order to be successful.
I think that is critically important to bring all those various concepts really together in the, in the right manner.
Richie Cotton: Okay. So, really broad set of skills. It sounds like this can be a role for generalists but you also need some pretty high aspirations, I guess, if you can bring all those teams together. All right. Super. Thank you for your time, Philip. This is very insightful stuff.
Philipp Herzig: No, thank you so much, Richie, for having me. It was a pleasure. Thank you so much for having me on the show.
blog
What is OpenAI's GPT-4o? Launch Date, How it Works, Use Cases & More
blog
AI Ethics: An Introduction
Vidhi Chugh
9 min
podcast
The 2nd Wave of Generative AI with Sailesh Ramakrishnan & Madhu Iyer, Managing Partners at Rocketship.vc
podcast
The Venture Mindset with Ilya Strebulaev, Economist Professor at Stanford Graduate School of Business
tutorial
Phi-3 Tutorial: Hands-On With Microsoft’s Smallest AI Model
tutorial