[DataFramed AI Series #2] How Organizations can Leverage ChatGPT
Noelle Silver Russell is the Global AI Solutions & Generative AI & LLM Industry Lead at Accenture, responsible for enterprise-scale industry playbooks for generative AI and LLMs.
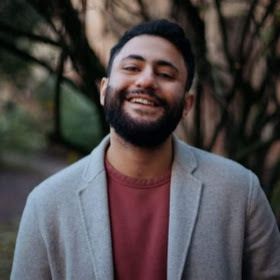
Adel is a Data Science educator, speaker, and Evangelist at DataCamp where he has released various courses and live training on data analysis, machine learning, and data engineering. He is passionate about spreading data skills and data literacy throughout organizations and the intersection of technology and society. He has an MSc in Data Science and Business Analytics. In his free time, you can find him hanging out with his cat Louis.
Key Quotes
There's so many places to get started with generative AI. I think one of the important things to do as an organization is to think holistically, to not narrow in. There are very easily accessible, low-hanging fruit. But as you think about it, these kinds of model are going to be pervasive. It will be disruptive to every line of business, to marketing, to sales, to finance, to legal. Where you start though, maybe it's in alignment with where most people think they would start, which is in conversations. Most of us have invested in chatbots as enterprise companies. Most of us, we're not extremely happy with the results. So that's a great place to start, but it's so important when you do choose to start there that you actually think, how does improving that customer experience in the chatbot, how does it change the rest of our workflows? What is impacted because of this bot? Now we can think about document intelligence, right? Now we have a GPT model that can support document intelligence. So now I don't need to hand off to a live agent if a birth certificate is needed or an insurance form is needed. I can actually do that ingestion, analysis, and extraction, all within the conversation and all completely robotic. So I think it's really thinking about more end-to-end from, I call it front office, middle office, back office. How do we make sure that when we're thinking about this model, we think about all of them?
One of the roles I have at Accenture is to create job aids. Every enterprise needs to really think about how it wants to frame the use of generative AI to augment the ingenuity of the individual? Both Microsoft and even Accenture share this as a core mission in the company, augmenting human possibility. And rather than saying, I'm going to leverage this technology in order to completely replace jobs, what we wanna be thinking instead is how do I augment them to do more? it's actually quite capitalist-focused. How do I not get rid of people, but actually use those people to do more things, to accelerate, create a bigger funnel, handle more customers. There is no lack of capability, no lack of growth that a company would want to see. So this actually accelerates growth by creating more efficiencies in your human talent pool. I think it's interesting when I end up delivering a generative AI solution. The humans that are using it are quite delighted, very happy. As a matter of fact, I delivered a PoC, not for production, and they're like, can we just start using it now? Like they wanted to use it right away. So I think there is another maybe myth there that we think the humans that are gonna see this are gonna be threatened by it, but I actually find those that I give this technology to are delighted to have it, are excited to use it, are not sad at all to have certain parts of their job that they don't have to do anymore. I do think the burden's on the leadership of an organization to set that culture, number one, and to create these job aids that help them use this technology in the right way.
Key Takeaways
When incorporating generative AI into the way you work, it is your responsibility to work with the AI tool, rather than have it work for you. In the case of creating dashboards using CoPilot in PowerBI, the output you get is only as good as what you ask for.
There is often a tradeoff between business efficiencies and customer experience, with the ability to use AI to automate customer-facing tasks, you can now give great customer experience, while also being efficient in your processes.
When looking to apply use cases for AI in your business, think about processes where AI can help throughout the entirety of the process, rather than how it can help on one particular task.
Transcript
Adel Nehme:
Noel Silver Russell, it's great to have you on the show.
Noelle Silver Russell:
Wonderful. I'm so excited to be here. Thanks for having me.
Adel Nehme:
So you are the global AI solutions lead and generative AI and LLM industry lead Accenture. You've been in the AI and technology space for over two decades now, maybe to break the ice and set the stage for our conversation. When was the last time a space and technology data AI was moving as fast as generative AI is moving today?
Noelle Silver Russell:
Yeah, I mean, of course, if you look at the data, there probably has not been anything that's moved this fast. However, a lot of the same news, press cycles, excitement really did happen as I got involved in AI in production early on in my days at Amazon Alexa. So I feel like a lot of the same things we were saying, this is the first time we put AI in the hands of a consumer. It's the first time. consumers can interact with AI freely, all these things we're saying now. So I sense a lot of the same patterns and actually we'll probably talk about this today. There's so many things we learned as we built those models, not just at Amazon, but also at Microsoft at scale that gosh, I hope we learned from those mistakes. Like it wasn't too long ago, as you mentioned, 10 years ago. But yeah, so I feel a lot of familiarity to this kind of scaling motion and interest. But I'm glad it's taken even more, you know, even more people are interested in ... See more
Adel Nehme:
That's definitely the case. You know, the crux of today's conversation and our special here is how organizations, especially enterprise organizations, can create value with generative AI. You know, tools like ChatGPT are on top of mind for almost every executive, every business leader. Maybe let's take a step back first and try to understand the different modes of value these tools unlock for organizations. So maybe walk us through your own words. What are the different levers of value creation ChatGPT unlocks for organizations today?
Noelle Silver Russell:
Yeah, that's a great question. I actually try to offer this every time I have a conversation, really with anyone about this technology, but specifically those that run organizations. I think there's a couple of different things. One, and probably the first thing that people think about when they think about GPT is customer experience. But it's a little bit different now because in the past, we used to try to attain business efficiencies and optimization, but almost at the cost of customer experience, right? Almost as a trade-off that either you make your business run better or you focus on a better customer experience, but you couldn't have both. And that is something that's really new about the ability for this technology to, yes, reduce friction for our customers, make it easier for them to do what they want to do, but at the same time, that allows us to deflect work from humans that ultimately had to handle those problems as they went through a flow. And so I think having the ability to do both business optimization and customer experience evolution. at the same time with the same model is something that's really, really impactful right now. I think another maybe myth I can bust that I often hear people talk about is that it is GPT or generative AI in general is a conversational agent. And so it's more about fixing chatbots and making them better. And what we're now seeing is really an evolution on business activity, business-to-business or process to process communication, right? How do I? get a, you know, I would love for example, for me to be working in one system and it detects certain signals from my work and be able to trigger natural language requests to other systems so that it's ready by the time I get there. And those are the types of things we're seeing now, right? How do we get systems to talk to each other without them having to be hard-coded to communicate to each other? And that I think is a pretty exciting opportunity as well.
Adel Nehme:
That's a really great framework of thinking about it, systems to systems communication without necessarily hard coding that. You mentioned a myth busting here, maybe as you're talking to executives, right, based on your conversations, what do you think are the most common misconception other than that myth that you just mentioned that leaders tend to have about generative AI and chat GPT and GPT in general?
Noelle Silver Russell:
Yes, I think, you know, Chat GPT, as it got very, very exciting and a lot more people started using it, I think people tended to think that that was the entirety of the technology. That Chat GPT, this web application, which is literally all it is, is a web application, that that was the capability. And what I try to, again, I do a lot of myth busting in my day to day, I try to encourage them to think. much bigger than that. That is one web application, but the underlying model is really what's interesting. And that that underlying model, another kind of myth, is that people think that that's the model they have to use. They don't realize that there's, you know, a collection of models out there. For example, you know, in an earlier session, AWS has a whole collection of types of models. Different cloud platform providers are gonna provide their own collection of foundation models to choose from. the capability is really quite expansive beyond just what you can do in ChatGPT. And most people are amazed by what ChatGPT can do. So just imagine how that changes when you can create your own private version of that, when you can fine tune it on your company, your company's data, when you can do it on a cloud platform that you have built and architected to be secure and private. All of these things we've been working for. almost a decade to build, right? This concept of a secure cloud infrastructure to run applications, generative AI now can sit native inside that environment. And most people, when they think of chat GPT and adding it to their enterprise, they think it's this external thing and they worry about the external nature of it, but really doesn't, in practice, it doesn't work that way. We actually are going to internalize it and make it part of our cloud infrastructure.
Adel Nehme:
That's very great. And I really love how you kind of, you know, create the separation between ChantGPT the application and, you know, GPT the model. We're going to talk about how that fine tuning layer of building, you know, APIs on top of your organization's data is going to play out in practice. But first, what I want to talk to you about is use cases, right? You know, a lot of organizations right now are looking at this technology and they're thinking, okay, what can I do with this technology? What should I prioritize first? Where are the low hanging fruits that I should approach with generative pre-trained transformers or, you know, generative AI in general? You know, while these models are extremely performant on some tasks, they tend to also hallucinate. They tend to provide wrong answers. They may even provide harmful answers in certain contexts. Maybe walk us through, given that nature of models where they are today as well. What is a use case prioritization framework that you can adopt as organizations are looking to adopt these use cases?
Noelle Silver Russell:
Yeah, absolutely. There's so many places to get started. And I think one of the important things to do as an organization is to think holistically, to not narrow in on, to your point, there are very easily accessible, low-hanging fruit. But as you think about it, this kind of model is going to be pervasive. It will be disruptive to every line of business, to marketing, to sales, to finance, to legal. So it will be pervasive. Where you start though, I think is... Relatively, maybe it's in alignment with where most people think they would start, which is in conversations. Most of us have invested in chat bots as enterprise companies. Most of us, we're not extremely happy with the results, right? They're still there though. We still are like, well, it's better than nothing, but it does have room for improvement and in some cases, significant improvement. So that's a great place to start, but it's so important when you do choose to start there that you actually think, how does... improving that customer experience in the chat bot, how does it change the rest of our workflows, right? What is impacted because of this bot? Now we can think about document intelligence, right? Now we have a GPT model can support document intelligence. So now I don't need to hand off to a live agent if a birth certificate is needed or insurance form is needed. I can actually do that ingestion, analysis, extraction, all within the conversation and all completely robotic. So I think it's really thinking about more end to end from, I call it front office, middle office, back office. How do we make sure that when we're thinking about this model, we think about all of them? Another easy point maybe to kind of round it out is maybe you don't want to change, like a lot of people consider their current digital engagement platform as like the lifeblood of their company. Maybe you don't want to mess around with that right now with this super innovative technology. there is an incredible opportunity for you to use GPT on the backend to analyze how those conversations are happening, to do named entity recognition, to do summarization. Like we used to have to build one model for each of these things. Now we have one model that does all of these things. And that can really help us get efficiencies where maybe we've either they're on our backlog for a data scientist to do, or maybe we've just forgotten long forgotten the ability to do it because of our resource constraints. Now you have a model that literally can augment the capabilities of that data science team.
Adel Nehme:
What that's a really interesting use case that you mentioned here, which is really the ability to kind of, you know, telescope the ability of a data team to create a lot of different natural language use cases, even if they're only on the backend, right, that create efficiencies. You know, you mentioned here how chat GPT and GPT models are going to transform, you know, every single line of business within the organization. Walk us through how should leaders think about the human component here? You know, there's a lot of talks about automation, the potential displacement of jobs. What the actual risks? What are the? You know, what's hype versus reality here in this situation, right? And how do you see that conversation playing out in the future, whether, you know, you're a leader or you're someone in the organization that's looking at this technology as a potential displacer or threat.
Noelle Silver Russell:
Yes, and I've been talking about this since actually Alexa. Alexa also created this sense that AI was going to replace people. And it's honestly not the first time, right? When robotic processes were launched in Detroit in the car industry of the United States, a lot of people also then were like, but it's going to displace people. And there was a bit of displacement, but there was also a replacement and an evolution of skills. And I think we're in that similar moment now. as any new innovation comes in, there's going to be things we as humans no longer do. I often will refer to like elevators. There was a time when a human was in the elevator and opened and closed the doors for us as humans. And now sometimes you get in and you don't even tell it what to do once you've stepped in the elevator. You've already predefined that beforehand, as scary as that might be. So I think it's a matter of really reframing and redefining. One of the roles I have at Accenture, for example, is to create job aids, right? So every enterprise needs to really think about how do I wanna frame the use of generative AI to augment the ingenuity of the individual? Both Microsoft and even Accenture share this as a core mission in the company, augmenting human possibility. And rather than saying, I'm going to leverage this technology in order to completely replace jobs, what we wanna be thinking instead is how do I augment them to do more? it's actually quite capitalist focused, right? Like how do I not get rid of people, but actually use those people to do more things, to accelerate, create a bigger funnel, handle more customers. There is no lack of capability, no lack of growth that a company would want to see. So this actually accelerates growth by creating more efficiencies in your human talent pool. And especially, I think it's interesting when I end up delivering a generative AI solution. The humans that are using it are quite delighted, very happy. As a matter of fact, I delivered a POC, not for production, and they're like, I mean, can we just start using it now? Like they wanted to use it right away. So I think there is another maybe myth there that we think the humans that are gonna see this are gonna be threatened by it, but I actually find those that I give this technology to are delighted to have it, are excited to use it, are not sad at all to have certain parts of their job. that they don't have to do anymore. But I do think the burden's on the leadership of an organization to set that culture, number one, and to create these job aids that help them use this technology in the right.
Adel Nehme:
I couldn't agree more, you know, especially on that augmentation aspect. And I think there is a deftly an opportunity to reframe the narrative from fear to excitement. I agree with you there, right? I think, you know, um, you know, Microsoft said, uh, and when they launch copilot for office, right? Like the elimination of drudgery. And I think that message really resonated with me as well. Maybe walk me through when you talk about a organization where larger language models are working side by side with people on variety of tasks across different lines of businesses. What does the new skill set of the future, what does the future of work look like and the needed skills to succeed with AI?
Noelle Silver Russell:
Yeah, so I guess this is probably a great opportunity to maybe introduce to some and reinforce for others the concept of prompt engineering or query engineering. How are we going to, because a large language model, I'll tell you, I was just using the new, the copilot version for Power BI from Microsoft. So cool. I have the ability to go in and it's a text box, just like ChatGPT. I type in what I want and it generates me a Power BI dashboard. However, just like with, AutoML, if you don't actually know Power BI, you won't even know what to ask for. You won't know what widgets you can use. There's a level of skill set that is still, even though it's going to completely change the amount of time it takes me to build a dashboard, I still have to know what a dashboard is, what widgets display the best information, what my leaders want. That's not going to be intuitive to a robotic process. It's not even something we can change. Because let's face it, our leaders change what they want all the time. Right? So this is where our humans were augmented. We don't have to build the models. We don't have to build the model of information ourselves, but we can use this AI to get us to that first draft much, much faster. And I think that's where some of the delight is, honestly, is that, oh my gosh, I now don't spend half my day building the dashboard, only then to focus on the story the data is telling. I now spend 10% of my day. building it with the augmentation of AI, and I spend the rest of my day trying to refine that story. And I think that is where a lot of the human capability right now is lost. People don't even have a chance to think about what they're building. They just spend most of their time, as you mentioned, in the drudgery of generating that content.
Adel Nehme:
It's pretty incredible when you think about the efficiency gains. You know, you mentioned here the Power BI example is an excellent example. You know, at DataCamp, we're pretty data science focused as well. Uh, one, uh, small task that we were trying to chat with you with is, you know, creating a machine learning workflow from ATC and Python, right? You still need to know to say, create a logistic regression,
Noelle Silver Russell:
Yes.
Adel Nehme:
use this accuracy metric, create these features, right? So very, very foundational to have that conceptual knowledge. But just as an aside, it's pretty crazy to imagine a future where creating a dashboard takes 10% of the day, right? Like the time warping effect of generative AI is very interesting. Noel, you mentioned here delivering POCs to customers and they're very excited about them. I'm not gonna ask you about what specific POCs look like, but maybe walk us through examples of organizations who have succeeded in adopting large language models with tools like ChatGPT, GPT-3, GPT-4. love to hear some of the examples that you've seen.
Noelle Silver Russell:
Now, I am a little biased because I am an MVP, Microsoft MVP in AI, so I have early access to a lot of the technology that we're now seeing come to market today. But I can show you a couple of different organizations that you will eventually be able to see the same things in the products you see every day that Microsoft just announced, Copilot, that it's going to show up in all of our tools. It's not quite there yet, but it's going to be any day now. I always wait, because I find, like, I think... GPT launched on Azure Open AI Service on a Saturday night, which I didn't know we did. So now I'm constantly on alert. But Microsoft is a great example, but also take a look at even GitHub, one of Microsoft's of course acquisitions, but it wasn't always. But GitHub ended up building out this copilot feature. I was a beta tester on that copilot feature, and I also was part of a team that is re-imagining what software engineering looks like at a company like Accenture. I mean Accenture has 700,000 employees, many of them are writing code every day. So what happens when we can refactor, even if it's minutes, 10 minutes, 15 minutes, across hundreds of thousands of employees, that productivity is expansive. So thinking about how do I use this technology, and again, not just to reduce friction for customer conversation, which is an easy go-to. from when we're using ChatGPT. But how do I ask it questions like you were mentioning, asking it functional questions? How do I, I don't know how many of us have spent time looking at code only, you know, like the worst thing that happens, you spend an hour, maybe two, looking at a piece of code and someone walks behind you and is like, oh yeah, yeah, it's right there. And you're like, oh. So one of the things I have to say, like the pair programming capability, right, of this, many companies realize, that even if they don't touch their line, even if they don't touch a customer, they can actually just change the way their engineers work on a daily basis. And now, there was a model, Codex. Codex has now been wrapped into GPT-4. So now GPT-4 can not only reduce friction for your customers and conversations, it can also help your engineers write better code. We're now seeing this one model do so many vastly different use cases across the enterprise. You can even think about it doing legal triage. Right? Uh, doing RCA for help desks for employees. Um, I mean, there's this one model now gets to serve a bunch of these capabilities within an organization. And I think that's what a lot of companies are realizing. They start with one, but then they're instantly moving on to what's our second, third, fourth use case. We light up across the company.
Adel Nehme:
Interesting you mentioned legal here. I was reading a report yesterday from Goldman Sachs that said that you know almost 40% of You know the legal industry right of tasks are subject to augmentation with
Noelle Silver Russell:
Absolutely.
Adel Nehme:
Generative AI right given how much document triage there is how much synthesis understanding summarization all these things very interesting You know you mentioned, you know use cases here. We talked about successful examples but I'd be remiss not to talk about the challenges associated with deploying generative AI in production. You know, I want to make sure that we speak deeply about these challenges because this is relatively in nascent technology, it's new, there's a lot of risks associated with these technologies for organizations. You don't want to be that brand that says something harmful in your chat bot, right? So what are the most common challenges that organizations face when deploying these models and how can they mitigate these challenges?
Noelle Silver Russell:
Yeah, I think the first challenge is really understanding that you can't just deploy GPT, that it is an enterprise. framework, like there's an entire selection or collection of tasks that are required. Everything from, it's very similar, it's almost like MLOps, right, for generative AI. Like we still have to do data selection, we still have to create an inclusive data set, even though the data set in GPT is much, or generative AI in general, much smaller, it's still necessary and it still needs to be inclusive. We then also have to consider fine tuning, right, how do I, and this accuracy and hallucinations, how do I tell the model? what answers I want it to provide to the world. If I am a retail company, do I want someone to come and ask my bot what to do with prisoners of war? Do I want it even able or capable of answering that question? If you go to chat GPT, it absolutely could answer that. So how do we make sure that we've put guardrails up for what our bot is willing to answer? Because it can based on the, I know you had mentioned, pre-trained transformer. For those of us, you know, that pre-training, understanding, we don't have... direct lineage to what that data is. But we do know it's about, you know, it's 499 plus million websites that were scraped in this process. And all of those websites, we know for sure, were for the most part written by humans. And those humans are biased. And as you know, humans embellish and they attribute false information. And so the model cannot be blamed for amplifying patterns it sees in that data, especially if it's wrong. it's usually modeling a pattern of attribution that if you go and look at the lineage of data you're like Oh, I see where you might have come to that conclusion, even if it's wrong. So I always tell people, models are never wrong. They're just simply untrained. And we do need to take more time, more care, and more effort in training them. The two things maybe that I hear the most from industry is one, around that concept of hallucination. How do I make sure my model isn't wrong? And that's where fine tuning, that's where prompt engineering, chain of thought prompting, like there's lots of techniques now is generated. I love this new role called prompt defender, right? A prompt defend mechanism for like cyber security and risks associated with that. I feel like you'll probably talk about that in another session, but there's a lot of opportunity around generative AI to make sure what kind of prompts can be supplied and how do we protect against prompts that are intentionally trying to access data that should not be accessible. All of this extremely important. The second part though, leans more into kind of the responsible AI side of the house that because we are using a model that is pre-trained on all of this data, it will also have systemic biases and patterns of behavior that we do not want to amplify. of this entire enterprise framework, a human in the loop, meaning a team of humans that are monitoring, right? All this data, monitoring the responses and making sure today, luckily, there are companies like Microsoft, like Amazon, that have implemented frameworks, packages, tool sets for monitoring toxicity, for monitoring injustice, for monitoring bias. But the funny thing is, is those tools are not new. I think what's new, hopefully, is a new type of leadership that knows to look at that data and take action on it. In machine learning, we've been looking at bias in machine learning for a long time, but will our leadership, or will the teams that leverage these black boxes, will they know to look at the data that's being provided by these models and say, oh, toxicity, what does that number even mean to us? That, I think, is the burden today, is how do we get the implement? and users of this technology to think more deeply about what it means to be responsible.
Adel Nehme:
Yeah. And a lot of ways, you know, it seems like we have shifted the gear in the industry, right? If you kind of want to compare maybe AI to COVID, that's a weird comparison, but you know, COVID happened in early 2020 and there was, you know, this shifted mindset that, oh, we're in a global pandemic now, everything has changed. Right. And I think in a lot of ways, Genetive AI has unlocked that, oh, we're in an AI era now, we need to adopt AI and we need to... need to adopt AI, we need to adopt the best practices and responsible AI technologies, methods, best practices and frameworks, right? So we do see that gear shift quite a lot and I'm optimistic there.
Yeah, so maybe let's shift gears a bit and talk about the actual data privacy and data management best practices because that's very top of mind when thinking about responsible AI because there could be risk of data leakage, there could be risk of, as you mentioned, prompt injection, right? Walk us through maybe how organizations should be thinking about data management, data privacy, when trying to fine tune APIs on their own data. What are the risks there, and what are the best practices that you recommend?
Noelle Silver Russell:
Absolutely. I think one of the first things, so I've been working with, through my Microsoft MVP relationship, working with OpenAI for a while. I think a few years ago I had an opportunity to interview some of the executives at OpenAI. Very exciting. It was before they were cool like they are now, but very exciting stuff. The vision was there in the same way. But one of the things that they were... discussing and now we see is that investment of Microsoft of a cloud provider. And this is true, not just for Microsoft, for AWS, for Google. Like there is a reason why we're going to leverage a cloud provider to support our deployment of these types of models. One, and maybe we'll talk about a little bit more, but the tech stack is pretty deep, right? So there's a lot of specialized hardware that's necessary to run a model that's trained on this much data. And not every company new chipset and do that work. Not everybody's going to want to do that. So what's the next best thing is to run inside of an infrastructure that has been secured, that is already private, that you actually as a company have already spent a lot of money and resources and time and you probably have an entire team of DevOps people that control this tenant in a cloud provider of your choice. And so those native services already get the benefit of the running on. So that's why even OpenAI, right, runs its entire infrastructure, runs on Azure, because they themselves as a company realize that that's not, they want to be a model research company. They don't want to worry about elastic scale or elastic search or database usage or blob stores. Like they don't want to worry about, they don't want to create that. They don't want to build it. So they offload that to a cloud provider. So I think when you're thinking about data privacy and security, I always say it's going to be as secure as you are, right? these cloud providers, it's called a shared responsibility model, which means like the infrastructure, the data center, all of that is going to be controlled by your cloud provider. then you're going to build your own tenant that you're going to secure. And so that tenancy, that security, that well architected nature of that tenant is gonna be completely up to you. But the good news is most of the enterprises today have been working on building secure cloud infrastructure for quite some time. So the benefit now of course, is we can use this technology in the context of that.
Adel Nehme:
So that's, you know, we've talking here on data privacy and data security when it comes to, you know, using APIs, but maybe how should they should think about it when, you know, the population within the organization is using tools like ChatGPT, you know, at the time of recording, I think today or yesterday, we had this, you know, scandal with Samsung, engineers were using private data in ChatGPT, some leakage happened. What should be the guidelines, you know, of using these types of models.
Noelle Silver Russell:
Oh my gosh. Yeah, this is a great question. So at Accenture, for example, months ago, like in the early part of this year, I was part of, there was just four of us in a room going, okay, we need to figure out how to like, lock this down. And so we ended up creating a center of excellence. That center of excellence ended up creating a set of guidelines for the company. We tied those guidelines to corporate policy. We had senior executive leaders of the company, these models in the wild. But the very next thing we had to do, and I think this is the the responsibility of a company, is that we then also gave them an enterprise sandbox so that they could play. It's very hard to tell someone, I always say when when you tell someone not to do something it almost you know, maybe I have too many children.
Adel Nehme:
hahahaha
Noelle Silver Russell:
You almost are like, I don't want to say like, don't do it, because that's going to make you want to do it more. But in this case, right, it is, we were one very transparent about the risks of exactly what we're seeing in the news today with some companies. There's a risk of that when you share any private data. So sure, go use it if you want to. You can use it to write an invite here, or maybe an agenda for a meeting there. But no client data, no Accenture data. But even then there's just risk in just like giving people the leeway to do that. So rather than say, no, you can't ever use this ever, like school systems did, right? We instead said, yeah, of course you can use it. Let's create an enterprise sandbox, which we built on OpenAI with Microsoft, create an enterprise sandbox, and you can now play in the context of our Azure tenant, Accenture's secured private Azure tenant. No data ingress or egress out of that tenant. already driven by security policies that we wrote. That will make the organization feel more comfortable. It'll give people a chance to play. So that's one of the things we do today. We have like this COE in a box where we provide guidance on these policies. We also then provide the ability for you to come and build an enterprise sandbox, whether it's on AWS, Azure, Google, wherever your cloud provisioning might be.
Adel Nehme:
I love that approach because at one point in time, you do preserve privacy, but I think it would be a massive disservice, especially, I can see
Noelle Silver Russell:
Yes!
Adel Nehme:
the education angle, but I don't see the enterprise organization complete barring it.
Noelle Silver Russell:
I agree.
Adel Nehme:
I saw a statistic a while ago that almost 73% of employees would refuse to work for a company that doesn't allow usage of Chatcha PT. So I think Chatcha PT is the new remote work in a lot of ways. So yeah, maybe walk me through as well, kind of thinking here about the data privacy. concept, you know, component of things. Let's talk about the responsible AI side of things as well. You know, a lot of the times, you know, I've been in data science for like six years, seven years now, transparency, explainability of models have been really important, right? The ability to understand why a model is making the decision it's making, right?
Noelle Silver Russell:
Yes.
Adel Nehme:
You know, I haven't seen a lot of, you know, compelling research on chat GPT or GPT models that are, they're easily explainable, right? Like a random forest is. Have we reached a moment where we have just accepted that black box models are here to stay?
Noelle Silver Russell:
Yes, that's an interesting question. I wouldn't say that. I think there's so many. I mean, granted, I am in the responsible AI community. I often speak on this topic. And I do find that many, like ethicists in general, would say, no, we are not at that stage.
Adel Nehme:
Yeah.
Noelle Silver Russell:
We are violently opposed to that stage. But I do see companies, for example, one of the really good news about... Azure OpenAI service when it launched, it launched with the Responsible AI Toolkit built in. That's new. Before it was an option, you could go out and get it, you could apply it, you could add the packages, but it's actually part of the solution. It's a tab in the deployment playground. That I think is part of what's different is that there is a call to action by enterprises to say, I don't want to use something, especially a black box AI model, that I cannot have some visibility to. I think Stanford Helm came up with this huge battery of metrics to measure, not just the accuracy and performance of these models, but also the toxicity and different levels of responsible metrics that we often would measure intentionally if I was doing that on site. So now seeing some, the challenge I think is mostly who's going to play well with the Stanford system of the world, who's going to make sure that they build. I was just talking to a partner. yesterday and they're like, oh, on our roadmap, we're building model cards that explain how decisions are made in this neural network. Because as you mentioned, I mean, it actually, we don't want to know, honestly. Like the whole point of a pre-trained model is that I don't want to know the details. I don't want to train it myself. But at the same time, there has to be some explainability. There has to be the read and audit ability. So one of my friends at Meta who runs the marketing organization there, like she was her must have said the word audit like 17 times in relation to her data science team in our very short conversation. And I realized that auditing a black box model is very, very difficult unless these types of mechanisms are provided. I wish it was a requirement, right? One thing I have noticed, especially as the economy kind of goes up and down, is that we have seasons of being very interested in responsible AI and doing the right thing and checking for accuracy, but as soon as things get tight, as soon as resources become less available, the economy takes a turn, as soon as that starts to happen, the teams that often get shed in those times are these responsible AI teams, right? And so that is something I'm looking forward to as we move into like maybe a conversation on regulation or policy. that we see where this isn't an option, right? Like building responsible tech is not on, the burden is not on the CEO, right? Like I always tell people I'm one acquisition away from the Death Star in any solution that I build. Like I never know who's gonna own it, who's gonna buy it. So how do I protect it? And honestly, I feel like regulation is one of the shining lights at the end of the tunnel that I'm looking for to help make this a requirement and not an option for enterprises.
Adel Nehme:
So let's jump in and regulation here. Walk me through what do you think good government regulation for AI and these types of tools should look like.
Noelle Silver Russell:
Yeah, I probably would start with the word collaboration, right? I think there's very, and not just collaboration with the big tech companies. I'm always surprised at how many lobbyists are employed by the big tech companies in these conversations, but really about consumers creating what I call kind of an inclusive data set, right?
Adel Nehme:
Yeah.
Noelle Silver Russell:
As we build these, I think there are lots of good examples of this. The EU just came out with, who knows if it'll pass, but, and maybe by the time someone hears this, it maybe it will. have. But even if it doesn't, it's a great set of guidelines for organizations to think about what to talk about. And the way that they built that was again by building an inclusive group of people across industries. Because when you build regulation, you really, I always think let's go for regulated industries first. Usually they're the highest risk. They also know regulation, so they're not foreign to the idea of being told what to do. Let's face it, engineers, data It's gonna be hard culturally to shift that monolith. However, organizations that are already this way, finance, healthcare, life sciences, there's opportunities for us to put in guardrails that are not far from guardrails they already have in other parts of their organization that will refine and protect users in some of the most important situations, right? Where their finances and independence is concerned or where their healthcare is concerned. So that's some of my guidance and I definitely have been working with I recently spoke at the Canadian consulate in New York to talk about where should we start? It's almost the same conversation you mentioned earlier with enterprises. What do we start with? Because if we try to boil the ocean with regulation, we'll just never... I mean, we're there now. We've been doing this for 20 years, and there's still no regulation. How do we pick something that we can identify and go after and begin without trying to do so much that we never get started?
Adel Nehme:
Yeah, and maybe harping on some what was a big story in the generative AI space a few weeks ago, that Future of Life letter on the moratorium of stopping AI research, right? Where do you stand on, you know, I see this debate happening within the AI community and the data science community is that regulation should be on the application level, not on the research level. Where do you stand on that debate?
Noelle Silver Russell:
Yeah, I would definitely lean more towards like research. Research and academia in general are also under a different level of regulation, a different level of usage. They are not production-based. They're not, you know, making money, which drives weird behavior, let's face it, in corporate organizations. So yeah, I do believe that research, and it goes along the lines of what I mentioned before. If I tell people not to do something, it's just gonna fuel the fire for those very specific people Yeah, we're gonna do this. I don't think there's harm in research It actually informs us as to things like there's lots of things that we researched I'll give you an example at Amazon Alexa We in our research organization found out that we could actually answer a question that was being asked to the device within about three words of the sentence meaning far by Like even if the three words were not contextually specifically about a topic, we could tell what question you were answering with 98% accuracy. However, it's very weird to have a device answer a question that you only have in your mind, right? Like it creeps people out. Like people don't like it. We'll see.
Adel Nehme:
What I wanna end on here in our conversation is, we talked about in our discussion about responsible AI, how organizations should adapt, but let's talk about the culture maybe a bit more deeply. data literacy quite a lot here on DataFrame. And I think AI literacy is something that we need to think about as well. We need to kind of combine data and AI literacy, especially as people, you know, as you mentioned, quite a lot of folks within organizations are interacting with tools like ChatGPT. How do you define AI literacy in the age of generative AI?
Noelle Silver Russell:
Yeah, so I often, I actually have a way that I frame this myself. I started a company called the AI Leadership Institute around this exact. kind of framework. And I always think, and I even explain myself when I introduce myself, I often will say this, that I like to go from the boardroom to the whiteboard to the keyboard. And I actually say any technologist should be able to do this, right? That as we build things, especially artificial intelligence, especially models that will serve as a foundation model to other companies, that we want to be able to explain it to a business from a business perspective, business value, business interests, but then also have a pretty technical discussion on, what does this take? One of my biggest concerns is around sustainability. Like, how much resources does this consume? What is the capacity that is required? Thinking about, you know, going from like that business level view to a highly, it's not high level, it's just an architectural level view. And then putting your fingers on the keyboard and building something. So I will go into an organization and do an executive briefing to get everyone in the company about artificial intelligence or even these foundation models, it's about what it means to be a data-driven organization, what it means to use data to build and leverage a model like GPT in order to gain insights. I love GPT models that I can ask questions like, what does my pipeline look like this week? What are the three accounts I should go after? Those insights are only available if we gathered the right data, trained the right model, me that information at the right time with the right levels of security. So I think it's really important to have that kind of end to end in an organization and to end understanding. And then of course, the keyboard part, I really like, I encourage organizations. Once the executives and the board are on, you know, have the same understanding, senior technical leadership is all on board. The best thing to do is get business and technology into a room and, you know, call it a hackathon, call it an innovation session. build something and you'd be amazed. I've done this at the Metropolitan Museum of Art. I've done it at Abbey Road Studios. You'd be amazed at what ends up happening when people who have the problem, the business, talk to people with the tech who have a solution. Like we came up with some pretty incredible stuff. One of them was even, you know, I think we had a story on NBC Nightly News because of its such interesting and most importantly accessible use cases. But without that, as you kind of in our own little bubble. And so it's really important for us to connect with the business and use our skills to make their vision a reality.
Adel Nehme:
In a lot of ways, you know, you know marrying that skill set but also having the different folks within the organization Speak to each other is an important aspect of creating that culture of AI literacy and the illiteracy but maybe as you Look at you know, the future of work and how leaders can foster AI literacy Do you think that the current education system is well prepared for the changes that are coming within the job market? Skillsets needed. What do you think needs to change to bridge that gap if not?
Noelle Silver Russell:
Yes, interesting. I don't, I would say that there are pockets of, of educational systems with leaders that are proactively technology focused and technology leaning. I will tell you in Florida, we have a mayor in Miami who's very technology focused mayor Suarez. Technology first. And as a result, the schools, universities, colleges in this area are also kind of building on that momentum. And then, so I recently had a fireside chat with the president of Miami Dade College. I'm in Miami, Florida. And we talked about, and she was one of the first to say, I will not ban this technology. I will equip my teachers and my professors and my students on how to use this to solve problems. And I thought like, that's kind of what we need, but at the same time, There's a bit of evangelism that's necessary to go spread that beyond just those who are lucky enough to have leadership that drives that conversation. What happens if you're in an organization, in a university, in a school? that doesn't see things that way. How can you show them the light, right? And so I often say build something. So one thing I did for Miami-Dade, I built what I eventually called the Intelligent Student Assistant, but it literally leveraged a GPT, or a large language model, wasn't GPT at the time, a large language model to reduce the amount of workload a faculty member got on scheduling TAs, and people would constantly ask what their grades were, when grades were coming out, did they get an assignment, that we could check within a system. And so that intelligent system ended off offloading 30% of the time of a faculty member. And like I said, the faculty was not worried that their jobs would be taken. They were ecstatic that they did not have to do this work anymore. And we did that without anyone asking. We just built it to be helpful. And it lit up the entire network of colleges to be like, What can we use this for? So I think that's why the power of learning by doing is so powerful, because if you build something useful, people will then start to see the opportunity that lies within this technology.
Adel Nehme:
And you mentioned here the importance of evangelism. I think you're doing an excellent job of that.
Noelle Silver Russell:
Thanks.
Adel Nehme:
Noelle. You know, as we close out our chat, I opened up our conversation saying, you know, when was the last time you saw space and technology AI move this fast, right? I want to end my conversation on a similar note. Where do you see the space being in 12 months from now?
Noelle Silver Russell:
So I think interestingly enough, we've already started to see it, but the models will just get better. And there'll be more provisioned models specific to industry. We're already starting to see it, right? I think Bloomberg recently released, or at least announced it's Bloomberg, meaning financially specific GPT model. We'll see, Microsoft Research has one on bio GPT. There's lots of, right now it's in its nasancy, but what happens to all these companies that are serving medical communities, patient communities, and now they don't even have to fine tune the model that much, right? I still have to go into a company and I still have to get its data and fine-tune the model to get it to answer what happens when we reduce what I call the time to value or time to market for these companies. So I feel like in 12 years we'll start to see an even bigger shift of organizations of all sizes starting to leverage this technology in meaningful ways.
Adel Nehme:
That is going to be very interesting. I'm excited for the app store for models. Noelle, before we wrap up, any final call to action before we wrap up today.
Noelle Silver Russell:
Yeah, I would encourage everyone to really learn by doing. There are some incredible GitHub repos available if you are a practitioner, even if you're not. I always reference GitHub as kind of tracing paper that artists use, right? Even if you're not proficient, you can use tracing paper and create pretty fantastic artwork. It's kind of like paint by numbers. A lot of our GitHub repos are this way. Microsoft, AWS, Google have all released samples. generative AI samples that you can build. Some of them even have released all of the infrastructure as code, I will not name it per each one, but you know what I mean, right? The scripts that are necessary. So you hit one button, launch a couple bash or shell scripts and it will build for you in your infrastructure. So just get in there and start playing around with this. This is exactly the time. Don't be that person two years from now that's like, I remember hearing about that. I should have dove in. Just dive in now, there's no harm in that and learning by doing will set the path for your way forward using this technology.
Adel Nehme:
I couldn't agree more. Learn by doing is something that we live by here at Data Camp. Thank you so much, Noelle, for joining us today. It was a really insightful discussion.
Noelle Silver Russell:
Yeah, super fun. Thank you for having me.
You’re invited! Join us for Radar: AI Edition
DataCamp Team
2 min
The Art of Prompt Engineering with Alex Banks, Founder and Educator, Sunday Signal
The Future of Programming with Kyle Daigle, COO at GitHub
A Comprehensive Guide to Working with the Mistral Large Model
Josep Ferrer
12 min
Serving an LLM Application as an API Endpoint using FastAPI in Python
How to Improve RAG Performance: 5 Key Techniques with Examples
Eugenia Anello