Beginning Bayes in R
This course provides a basic introduction to Bayesian statistics in R.
Start Course for Free4 Horas17 Videos56 Exercises
Create Your Free Account
or
By continuing, you accept our Terms of Use, our Privacy Policy and that your data is stored in the USA.Training 2 or more people?Try DataCamp For Business
Loved by learners at thousands of companies
Descrição do Curso
There are two schools of thought in the world of statistics, the frequentist perspective and the Bayesian perspective. At the core of the Bayesian perspective is the idea of representing your beliefs about something using the language of probability, collecting some data, then updating your beliefs based on the evidence contained in the data. This provides a convenient way of implementing the scientific method for learning about the world we live in. Bayesian statistics is increasingly popular due to recent improvements in computation, the ability to fit a wide range of models, and to produce intuitive interpretations of the results.
For Business
Training 2 or more people?
Get your team access to the full DataCamp library, with centralized reporting, assignments, projects and more- 1
Introduction to Bayesian thinking
LivreThis chapter introduces the idea of discrete probability models and Bayesian learning. You'll express your opinion about plausible models by defining a prior probability distribution, you'll observe new information, and then, you'll update your opinion about the models by applying Bayes' theorem.
Discrete probability distributions50 xpMost likely spin?50 xpChance of spinning an even number?50 xpConstructing a spinner100 xpSimulating spinner data100 xpBayes' rule50 xpThe prior50 xpThe likelihoods50 xpThe posterior100 xpInterpreting the posterior50 xpSequential Bayes50 xpBayes with another spin100 xp - 2
Learning about a binomial probability
This chapter describes learning about a population proportion using discrete and continuous models. You'll use a beta curve to represent prior opinion about the proportion, take a sample and observe the number of successes and failures, and construct a beta posterior curve that combines both the information in the prior and in the sample. You'll then use the beta posterior curve to draw inferences about the population proportion.
Bayes with discrete models50 xpConstructing a discrete prior50 xpUpdating by Bayes' rule100 xpContinuous prior50 xpWorking with a beta curve100 xpConstructing a beta prior100 xpUpdating the beta prior50 xpBayesian updating100 xpTesting a claim100 xpBayesian inference50 xpInterval estimates100 xpCompare with classical methods100 xpBayesian probability interval50 xpPosterior simulation50 xpSimulating from a beta curve100 xpWhy simulate?100 xp - 3
Learning about a normal mean
This chapter introduces Bayesian learning about a population mean. You'll sample from a normal population with an unknown mean and a known standard deviation, construct a normal prior to reflect your opinion about the location of the mean before sampling, and see that the posterior distribution also has a normal form with updated values of the mean and standard deviation. You'll also get more practice drawing inferences from the posterior distribution, only this time, about a population mean.
Normal sampling model50 xpReaction times100 xpUpdating by Bayes' rule100 xpBayes with a continuous prior50 xpA continuous prior100 xpComparing normal priors50 xpUpdating the normal prior50 xpThe normal posterior100 xpCompare with classical interval100 xpIs Jim slow?50 xpSimulation50 xpPosterior simulation100 xpPredictive simulation100 xpPrediction vs. inference50 xp - 4
Bayesian comparisons
Suppose you're interested in comparing proportions from two populations. You take a random sample from each population and you want to learn about the difference in proportions. This chapter will illustrate the use of discrete and continuous priors to do this kind of inference. You'll use a Bayesian regression approach to learn about a mean or the difference in means when the sampling standard deviation is unknown.
Comparing two proportions50 xpA discrete prior100 xpDiscrete posterior100 xpProportions with continuous priors50 xpIndependent beta priors100 xpPosterior of proportions100 xpComparison of proportions100 xpNormal model inference50 xpUniform prior100 xpLearning about a percentile100 xpBayesian regression50 xpTwo group model100 xpStandardized effect inference100 xpWrap-up and review50 xp
For Business
Training 2 or more people?
Get your team access to the full DataCamp library, with centralized reporting, assignments, projects and moreCollaborators
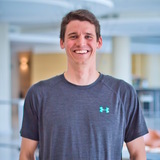
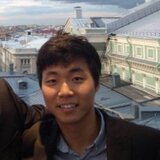
Jim Albert
Veja MaisProfessor, Bowling Green State University
What do other learners have to say?
Join over 13 million learners and start Beginning Bayes in R today!
Create Your Free Account
or
By continuing, you accept our Terms of Use, our Privacy Policy and that your data is stored in the USA.