Dealing with Missing Data in Python
Learn how to identify, analyze, remove and impute missing data in Python.
Comience El Curso Gratis4 Horas14 Videos46 Ejercicios
Crea Tu Cuenta Gratuita
o
Al continuar, acepta nuestros Términos de uso, nuestra Política de privacidad y que sus datos se almacenan en los EE. UU.¿Entrenar a 2 o más personas?Pruebe DataCamp para empresas
Preferido por estudiantes en miles de empresas
Descripción del curso
Tired of working with messy data? Did you know that most of a data scientist's time is spent in finding, cleaning and reorganizing data?! Well turns out you can clean your data in a smart way! In this course Dealing with Missing Data in Python, you'll do just that! You'll learn to address missing values for numerical, and categorical data as well as time-series data. You'll learn to see the patterns the missing data exhibits! While working with air quality and diabetes data, you'll also learn to analyze, impute and evaluate the effects of imputing the data.
Empresas
¿Entrenar a 2 o más personas?
Obtenga acceso de su equipo a la biblioteca completa de DataCamp, con informes centralizados, tareas, proyectos y másEn las siguientes pistas
Caja de herramientas Python
Ir a la pista- 1
The Problem With Missing Data
GratuitoGet familiar with missing data and how it impacts your analysis! Learn about different null value operations in your dataset, how to find missing data and summarizing missingness in your data.
Why deal with missing data?50 xpSteps for treating missing values50 xpNull value operations100 xpFinding Null values100 xpHandling missing values50 xpDetecting missing values100 xpReplacing missing values100 xpReplacing hidden missing values100 xpAnalyze the amount of missingness50 xpAnalyzing missingness percentage100 xpVisualize missingness100 xp - 2
Does Missingness Have A Pattern?
Analyzing the type of missingness in your dataset is a very important step towards treating missing values. In this chapter, you'll learn in detail how to establish patterns in your missing and non-missing data, and how to appropriately treat the missingness using simple techniques such as listwise deletion.
Is the data missing at random?50 xpGuess the missingness type100 xpDeduce MNAR100 xpFinding patterns in missing data50 xpFinding correlations in your data100 xpIdentify the missingness type50 xpVisualizing missingness across a variable50 xpFill dummy values100 xpGenerate scatter plot with missingness100 xpWhen and how to delete missing data50 xpDelete MCAR100 xpWill you delete?100 xp - 3
Imputation Techniques
Embark on the world of data imputation! In this chapter, you will apply basic imputation techniques to fill in missing data and visualize your imputations to be able to evaluate your imputations' performance.
Mean, median & mode imputations50 xpMean & median imputation100 xpMode and constant imputation100 xpVisualize imputations100 xpImputing time-series data50 xpFilling missing time-series data100 xpImpute with interpolate method100 xpVisualizing time-series imputations50 xpVisualize forward fill imputation100 xpVisualize backward fill imputation100 xpPlot interpolations100 xp - 4
Advanced Imputation Techniques
Finally, go beyond simple imputation techniques and make the most of your dataset by using advanced imputation techniques that rely on machine learning models, to be able to accurately impute and evaluate your missing data. You will be using methods such as KNN and MICE in order to get the most out of your missing data!
Imputing using fancyimpute50 xpKNN imputation100 xpMICE imputation100 xpImputing categorical values50 xpOrdinal encoding of a categorical column100 xpOrdinal encoding of a DataFrame100 xpKNN imputation of categorical values100 xpEvaluation of different imputation techniques50 xpAnalyze the summary of linear model100 xpComparing and choosing the best adjusted R-squared100 xpComparing density plots100 xpConclusion50 xp
Empresas
¿Entrenar a 2 o más personas?
Obtenga acceso de su equipo a la biblioteca completa de DataCamp, con informes centralizados, tareas, proyectos y másEn las siguientes pistas
Caja de herramientas Python
Ir a la pistaColaboradores
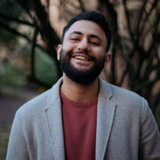
Suraj Donthi
Ver MasDeep Learning & Computer Vision Consultant
¿Qué tienen que decir otros alumnos?
Únete a 13 millones de estudiantes y empeza Dealing with Missing Data in Python hoy!
Crea Tu Cuenta Gratuita
o
Al continuar, acepta nuestros Términos de uso, nuestra Política de privacidad y que sus datos se almacenan en los EE. UU.