Modeling with Data in the Tidyverse
Discover different types in data modeling, including for prediction, and learn how to conduct linear regression and model assessment measures in the Tidyverse.
Start Course for Free4 Hours17 Videos49 Exercises
Create Your Free Account
or
By continuing, you accept our Terms of Use, our Privacy Policy and that your data is stored in the USA.Training 2 or more people?Try DataCamp For Business
Loved by learners at thousands of companies
Course Description
In this course, you will learn to model with data. Models attempt to capture the relationship between an outcome variable of interest and a series of explanatory/predictor variables. Such models can be used for both explanatory purposes, e.g. "Does knowing professors' ages help explain their teaching evaluation scores?", and predictive purposes, e.g., "How well can we predict a house's price based on its size and condition?" You will leverage your tidyverse skills to construct and interpret such models. This course centers around the use of linear regression, one of the most commonly-used and easy to understand approaches to modeling. Such modeling and thinking is used in a wide variety of fields, including statistics, causal inference, machine learning, and artificial intelligence.
For Business
Training 2 or more people?
Get your team access to the full DataCamp library, with centralized reporting, assignments, projects and moreIn the following Tracks
Tidyverse Fundamentals with R
Go To Track- 1
Introduction to Modeling
FreeThis chapter will introduce you to some background theory and terminology for modeling, in particular, the general modeling framework, the difference between modeling for explanation and modeling for prediction, and the modeling problem. Furthermore, you'll start performing your first exploratory data analysis, a crucial first step before any formal modeling.
Background on modeling for explanation50 xpExploratory visualization of age100 xpNumerical summaries of age100 xpBackground on modeling for prediction50 xpExploratory visualization of house size100 xpLog10 transformation of house size100 xpThe modeling problem for explanation50 xpEDA of relationship of teaching & "beauty" scores100 xpCorrelation between teaching and "beauty" scores100 xpThe modeling problem for prediction50 xpEDA of relationship of house price and waterfront100 xpPredicting house price with waterfront100 xp - 2
Modeling with Basic Regression
Equipped with your understanding of the general modeling framework, in this chapter, we'll cover basic linear regression where you'll keep things simple and model the outcome variable y as a function of a single explanatory/ predictor variable x. We'll use both numerical and categorical x variables. The outcome variable of interest in this chapter will be teaching evaluation scores of instructors at the University of Texas, Austin.
Explaining teaching score with age50 xpPlotting a "best-fitting" regression line100 xpFitting a regression with a numerical x100 xpPredicting teaching score using age50 xpMaking predictions using "beauty score"100 xpComputing fitted/predicted values & residuals100 xpExplaining teaching score with gender50 xpEDA of relationship of score and rank100 xpFitting a regression with a categorical x100 xpPredicting teaching score using gender50 xpMaking predictions using rank50 xpVisualizing the distribution of residuals100 xp - 3
Modeling with Multiple Regression
In the previous chapter, you learned about basic regression using either a single numerical or a categorical predictor. But why limit ourselves to using only one variable to inform your explanations/predictions? You will now extend basic regression to multiple regression, which allows for incorporation of more than one explanatory or one predictor variable in your models. You'll be modeling house prices using a dataset of houses in the Seattle, WA metropolitan area.
Explaining house price with year & size50 xpEDA of relationship100 xpFitting a regression100 xpPredicting house price using year & size50 xpMaking predictions using size and bedrooms100 xpInterpreting residuals100 xpExplaining house price with size & condition50 xpParallel slopes model100 xpInterpreting the parallel slopes model50 xpPredicting house price using size & condition50 xpMaking predictions using size and waterfront100 xpAutomating predictions on "new" houses100 xp - 4
Model Assessment and Selection
In the previous chapters, you fit various models to explain or predict an outcome variable of interest. However, how do we know which models to choose? Model assessment measures allow you to assess how well an explanatory model "fits" a set of data or how accurate a predictive model is. Based on these measures, you'll learn about criteria for determining which models are "best".
Model selection and assessment50 xpRefresher: sum of squared residuals100 xpWhich model to select?50 xpAssessing model fit with R-squared50 xpComputing the R-squared of a model100 xpComparing the R-squared of two models100 xpAssessing predictions with RMSE50 xpComputing the MSE & RMSE of a model100 xpComparing the RMSE of two models100 xpValidation set prediction framework50 xpFitting model to training data100 xpPredicting on test data100 xpConclusion - Where to go from here?50 xp
For Business
Training 2 or more people?
Get your team access to the full DataCamp library, with centralized reporting, assignments, projects and moreIn the following Tracks
Tidyverse Fundamentals with R
Go To TrackCollaborators
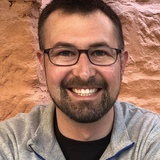
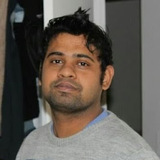
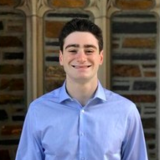
Prerequisites
Data Manipulation with dplyrAlbert Y. Kim
See MoreAssociate Professor of Statistical & Data Sciences, Smith College
What do other learners have to say?
Join over 13 million learners and start Modeling with Data in the Tidyverse today!
Create Your Free Account
or
By continuing, you accept our Terms of Use, our Privacy Policy and that your data is stored in the USA.