Cleaning Data in R
Learn to clean data as quickly and accurately as possible to help your business move from raw data to awesome insights.
Start Course for Free4 Hours13 Videos44 Exercises
Create Your Free Account
or
By continuing, you accept our Terms of Use, our Privacy Policy and that your data is stored in the USA.Training 2 or more people?Try DataCamp For Business
Loved by learners at thousands of companies
Course Description
Overcome Common Data Problems Like Removing Duplicates in R
It's commonly said that data scientists spend 80% of their time cleaning and manipulating data and only 20% of their time analyzing it. The time spent cleaning is vital since analyzing dirty data can lead you to draw inaccurate conclusions.In this course, you’ll learn a variety of techniques to help you clean dirty data using R. You’ll start by converting data types, applying range constraints, and dealing with full and partial duplicates to avoid double-counting.
Delve into Advanced Data Challenges
Once you’ve practiced working on common data issues, you’ll move on to more advanced challenges such as ensuring consistency in measurements and dealing with missing data. After every new concept, you’ll have the chance to complete a hands-on exercise to cement your knowledge and build your experience.Learn to Use Record Linkage During Data Cleaning
Record Linkage is used to merge datasets together when the values have issues such as typos or different spellings. You’ll explore this useful technique in the final chapter and practice the application by using it to join two restaurant review datasets together into a single dataset.For Business
Training 2 or more people?
Get your team access to the full DataCamp library, with centralized reporting, assignments, projects and moreIn the following Tracks
Importing & Cleaning Data with R
Go To Track- 1
Common Data Problems
FreeIn this chapter, you'll learn how to overcome some of the most common dirty data problems. You'll convert data types, apply range constraints to remove future data points, and remove duplicated data points to avoid double-counting.
- 2
Categorical and Text Data
Categorical and text data can often be some of the messiest parts of a dataset due to their unstructured nature. In this chapter, you’ll learn how to fix whitespace and capitalization inconsistencies in category labels, collapse multiple categories into one, and reformat strings for consistency.
- 3
Advanced Data Problems
In this chapter, you’ll dive into more advanced data cleaning problems, such as ensuring that weights are all written in kilograms instead of pounds. You’ll also gain invaluable skills that will help you verify that values have been added correctly and that missing values don’t negatively impact your analyses.
- 4
Record Linkage
Record linkage is a powerful technique used to merge multiple datasets together, used when values have typos or different spellings. In this chapter, you'll learn how to link records by calculating the similarity between strings—you’ll then use your new skills to join two restaurant review datasets into one clean master dataset.
Comparing strings50 xpCalculating distance50 xpSmall distance, small difference100 xpFixing typos with string distance100 xpGenerating and comparing pairs50 xpLink or join?100 xpPair blocking100 xpComparing pairs100 xpScoring and linking50 xpScore then select or select then score?100 xpPutting it together100 xpCongratulations!50 xp
For Business
Training 2 or more people?
Get your team access to the full DataCamp library, with centralized reporting, assignments, projects and moreIn the following Tracks
Importing & Cleaning Data with R
Go To TrackCollaborators
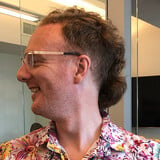
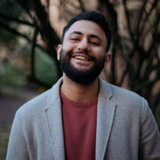
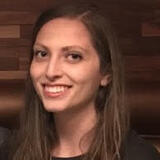
Prerequisites
Joining Data with dplyrMaggie Matsui
See MoreCurriculum Manager at DataCamp
Maggie is a Curriculum Manager at DataCamp. She holds a Bachelor's degree in Statistics and Computer Science from Brown University, where she spent lots of time teaching math, programming, and statistics as a tutor and teaching assistant. She's passionate about teaching all things data-related and making programming accessible to everyone.
FAQs
Join over 13 million learners and start Cleaning Data in R today!
Create Your Free Account
or
By continuing, you accept our Terms of Use, our Privacy Policy and that your data is stored in the USA.